This is the multi-page printable view of this section. Click here to print.
W&B App UI Reference
- 1: Panels
- 1.1: Line plots
- 1.1.1: Line plot reference
- 1.1.2: Point aggregation
- 1.1.3: Smooth line plots
- 1.2: Bar plots
- 1.3: Parallel coordinates
- 1.4: Scatter plots
- 1.5: Save and diff code
- 1.6: Parameter importance
- 1.7: Compare run metrics
- 1.8: Query panels
- 1.8.1: Embed objects
- 2: Custom charts
- 3: Manage workspace, section, and panel settings
- 4: Settings
- 4.1: Manage user settings
- 4.2: Manage billing settings
- 4.3: Manage team settings
- 4.4: Manage email settings
- 4.5: Manage teams
- 4.6: Manage storage
- 4.7: System metrics
- 4.8: Anonymous mode
1 - Panels
Use workspace panel visualizations to explore your logged data by key, visualize the relationships between hyperparameters and output metrics, and more.
Workspace modes
W&B projects support two different workspace modes. The icon next to the workspace name shows its mode.
Icon | Workspace mode |
---|---|
Automated workspaces automatically generate panels for all keys logged in the project. This can help you get started by visualizing all available data for the project. | |
Manual workspaces start as blank slates and display only those panels intentionally added by users. Choose a manual workspace when you care mainly about a fraction of the keys logged in the project, or for a more focused analysis. |
To change how a workspace generates panels, reset the workspace.
Undo changes to your workspace
To undo changes to your workspace, click the Undo button (arrow that points left) or type CMD + Z (macOS) or CTRL + Z (Windows / Linux).Reset a workspace
To reset a workspace:
- At the top of the workspace, click the action menu
...
. - Click Reset workspace.
View a panel in full-screen mode
In full-screen mode, the run selector displays and panels use full full-fidelity sampling mode plots with 10,000 buckets, rather than 1000 buckets otherwise.
To view a panel in full-screen mode:
- Hover over the panel.
- Click the panel’s action menu
...
, then click the full-screen button, which looks like a viewfinder or an outline showing the four corners of a square. - When you share the panel while viewing it in full-screen mode, the resulting link opens in full-screen mode automatically.
To get back to a panel’s workspace from full-screen mode, click the left-pointing arrow at the top of the page.
Add panels
You can add panels to your workspace, either globally or at the section level.
To add a panel:
-
To add a panel globally, click Add panels in the control bar near the panel search field.
-
To add a panel directly to a section instead, click the section’s action
...
menu, then click + Add panels. -
Select the type of panel to add.
Quick add
Quick Add allows you to select a key in the project from a list to generate a standard panel for it.
For an automated workspace with no deleted panels, Quick add is not available. You can use Quick add to re-add a panel that you deleted.
Custom panel add
To add a custom panel to your workspace:
- Select the type of panel you’d like to create.
- Follow the prompts to configure the panel.
To learn more about the options for each type of panel, refer to the relevant section below, such as Line plots or Bar plots.
Share a panel
This section shows how to share a panel using a link.
To share a panel using a link, you can either:
- While viewing the panel in full-screen mode, copy the URL from the browser.
- Click the action menu
...
and select Copy panel URL.
Share the link with the user or team. When they access the link, the panel opens in full-screen mode.
To return to a panel’s workspace from full-screen mode, click the left-pointing arrow at the top of the page.
Compose a panel’s full-screen link programmatically
In certain situations, such as when creating an automation, it can be useful to include the panel’s full-screen URL. This section shows the format for a panel’s full-screen URL. In the proceeding example, replace the entity, project, panel, and section names in brackets.
https://wandb.ai/<ENTITY_NAME>/<PROJECT_NAME>?panelDisplayName=<PANEL_NAME>&panelSectionName=<SECTON_NAME>
If multiple panels in the same section have the same name, this URL opens the first panel with the name.
Embed or share a panel on social media
To embed a panel in a website or share it on social media, the panel must be viewable by anyone with the link. If a project is private, only members of the project can view the panel. If the project is public, anyone with the link can view the panel.
To get the code to embed or share a panel on social media:
- From the workspace, hover over the panel, then click its action menu
...
. - Click the Share tab.
- Change Only those who are invited have access to Anyone with the link can view. Otherwise, the choices in the next step are not available.
- Choose Share on Twitter, Share on Reddit, Share on LinkedIn, or Copy embed link.
Email a panel report
To email a single panel as a stand-alone report:
- Hover over the panel, then click the panel’s action menu
...
. - Click Share panel in report.
- Select the Invite tab.
- Enter an email address or username.
- Optionally, change can view to can edit.
- Click Invite. W&B sends an email to the user with a clickable link to the report that contains only the panel you are sharing.
Unlike when you share a panel, the recipient cannot get to the workspace from this report.
Manage panels
Edit a panel
To edit a panel:
- Click its pencil icon.
- Modify the panel’s settings.
- To change the panel to a different type, select the type and then configure the settings.
- Click Apply.
Move a panel
To move a panel to a different section, you can use the drag handle on the panel. To select the new section from a list instead:
- If necessary, create a new section by clicking Add section after the last section.
- Click the action
...
menu for the panel. - Click Move, then select a new section.
You can also use the drag handle to rearrange panels within a section.
Duplicate a panel
To duplicate a panel:
- At the top of the panel, click the action
...
menu. - Click Duplicate.
If desired, you can customize or move the duplicated panel.
Remove panels
To remove a panel:
- Hover your mouse over the panel.
- Select the action
...
menu. - Click Delete.
To remove all panels from a manual workspace, click its action ...
menu, then click Clear all panels.
To remove all panels from an automatic or manual workspace, you can reset the workspace. Select Automatic to start with the default set of panels, or select Manual to start with an empty workspace with no panels.
Manage sections
By default, sections in a workspace reflect the logging hierarchy of your keys. However, in a manual workspace, sections appear only after you start adding panels.
Add a section
To add a section, click Add section after the last section.
To add a new section before or after an existing section, you can instead click the section’s action ...
menu, then click New section below or New section above.
Manage a section’s panels
Sections with a large number of panels are paginated by default if they use the Standard grid layout. The default number of panels on a page depend on the panel’s configuration and on the sizes of the panels in the section.
- To check which layout a section uses, click the section’s action
...
menu. To change a section’s layout, select Standard grid or Custom grid in the Layout grid section. - To resize a panel, hover over it, click the drag handle, and drag it to adjust the panel’s size.
- If a section uses the Standard grid, resizing one panel resizes all panels in the section.
- If a section uses the Custom grid, you can customize the size of each panel separately.
- If a section is paginated, you can customize the number of panels to show on a page:
- At the top of the section, click 1 to
of , where<X>
is the number of visible panels and<Y>
is the total number of panels. - Choose how many panels to show per page, up to 100.
- To show all panels when there are a large number of them, configure the panel to use the Custom grid layout. Click the section’s action
...
menu, then select Custom grid in the Layout grid section - To delete a panel from a section:
- Hover over the panel, then click its action
...
menu. - Click Delete.
If you reset a workspace to an automated workspace, all deleted panels appear again.
Rename a section
To rename a section, click its action ...
menu, then click Rename section.
Delete a section
To delete a section, click its ...
menu, then click Delete section. This removes the section and its panels.
1.1 - Line plots
Line plots show up by default when you plot metrics over time with wandb.log(). Customize with chart settings to compare multiple lines on the same plot, calculate custom axes, and rename labels.
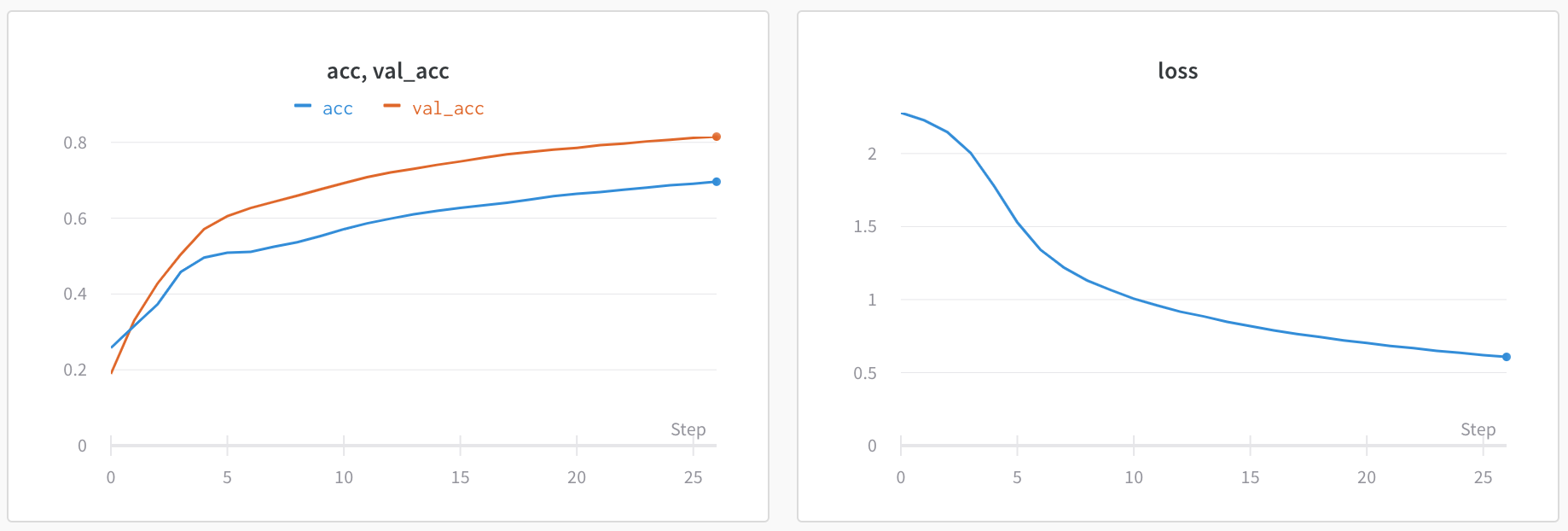
Edit line panel settings
Hover your mouse over the panel you want to edit its settings for. Select the pencil icon that appears. Within the modal that appears, select a tab to edit the Data, Grouping, Chart, Expressions, or Legend settings.
Data
Select the Data tab to edit the x-axis, y-axis, smoothing filter, point aggregation and more. The proceeding describes some of the options you can edit:
wandb.log()
that you use to log the y-axis.- X axis: By default the x-axis is set to Step. You can change the x-axis to Relative Time, or select a custom axis based on values you log with W&B.
- Relative Time (Wall) is clock time since the process started, so if you started a run and resumed it a day later and logged something that would be plotted a 24hrs.
- Relative Time (Process) is time inside the running process, so if you started a run and ran for 10 seconds and resumed a day later that point would be plotted at 10s
- Wall Time is minutes elapsed since the start of the first run on the graph
- Step increments by default each time
wandb.log()
is called, and is supposed to reflect the number of training steps you’ve logged from your model
- Y axes: Select y-axes from the logged values, including metrics and hyperparameters that change over time.
- Min, max, and log scale: Minimum, maximum, and log scale settings for x axis and y axis in line plots
- Smoothing: Change the smoothing on the line plot.
- Outliers: Rescale to exclude outliers from the default plot min and max scale
- Max runs to show: Show more lines on the line plot at once by increasing this number, which defaults to 10 runs. You’ll see the message “Showing first 10 runs” on the top of the chart if there are more than 10 runs available but the chart is constraining the number visible.
- Chart type: Change between a line plot, an area plot, and a percentage area plot
Grouping
Select the Grouping tab to use group by methods to organize your data.
- Group by: Select a column, and all the runs with the same value in that column will be grouped together.
- Agg: Aggregation— the value of the line on the graph. The options are mean, median, min, and max of the group.
Chart
Select the Chart tab to edit the plot’s title, axis titles, legend, and more.
- Title: Add a custom title for line plot, which shows up at the top of the chart
- X-Axis title: Add a custom title for the x-axis of the line plot, which shows up in the lower right corner of the chart.
- Y-Axis title: Add a custom title for the y-axis of the line plot, which shows up in the upper left corner of the chart.
- Show legend: Toggle legend on or off
- Font size: Change the font size of the chart title, x-axis title, and y-axis title
- Legend position: Change the position of the legend on the chart
Legend
- Legend: Select field that you want to see in the legend of the plot for each line. You could, for example, show the name of the run and the learning rate.
- Legend template: Fully customizable, this powerful template allows you to specify exactly what text and variables you want to show up in the template at the top of the line plot as well as the legend that appears when you hover your mouse over the plot.
Expressions
- Y Axis Expressions: Add calculated metrics to your graph. You can use any of the logged metrics as well as configuration values like hyperparameters to calculate custom lines.
- X Axis Expressions: Rescale the x-axis to use calculated values using custom expressions. Useful variables include**_step** for the default x-axis, and the syntax for referencing summary values is
${summary:value}
Visualize average values on a plot
If you have several different experiments and you’d like to see the average of their values on a plot, you can use the Grouping feature in the table. Click “Group” above the run table and select “All” to show averaged values in your graphs.
Here is what the graph looks like before averaging:
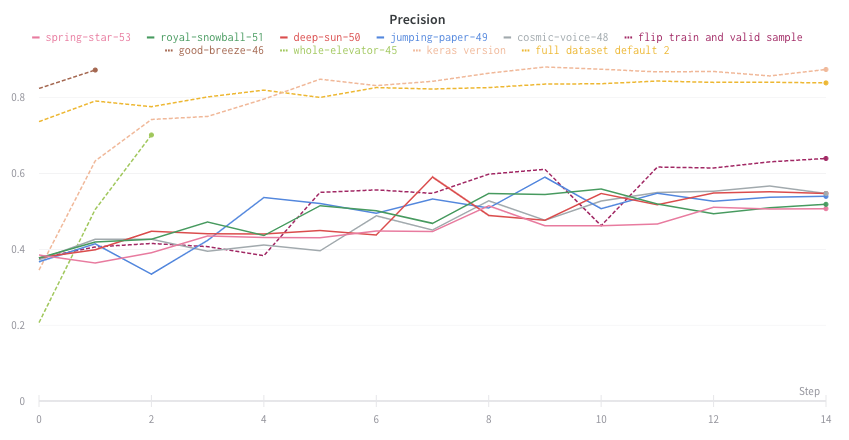
The proceeding image shows a graph that represents average values across runs using grouped lines.
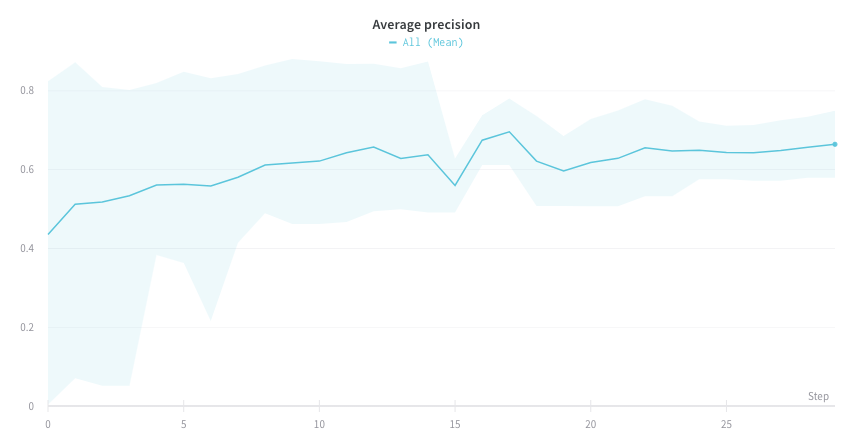
Visualize NaN value on a plot
You can also plot NaN
values including PyTorch tensors on a line plot with wandb.log
. For example:
wandb.log({"test": [..., float("nan"), ...]})
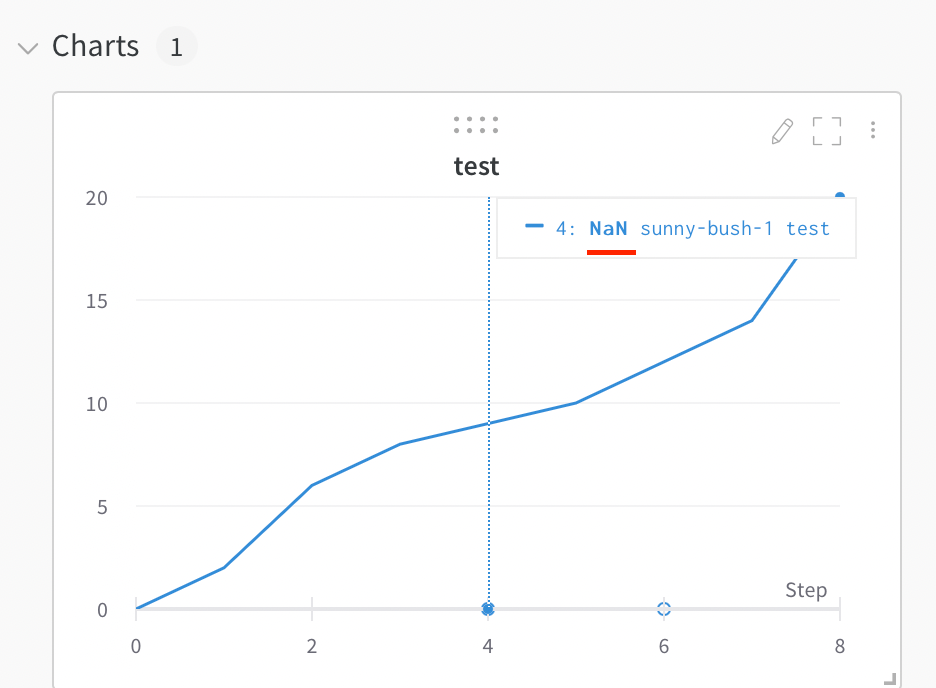
Compare two metrics on one chart
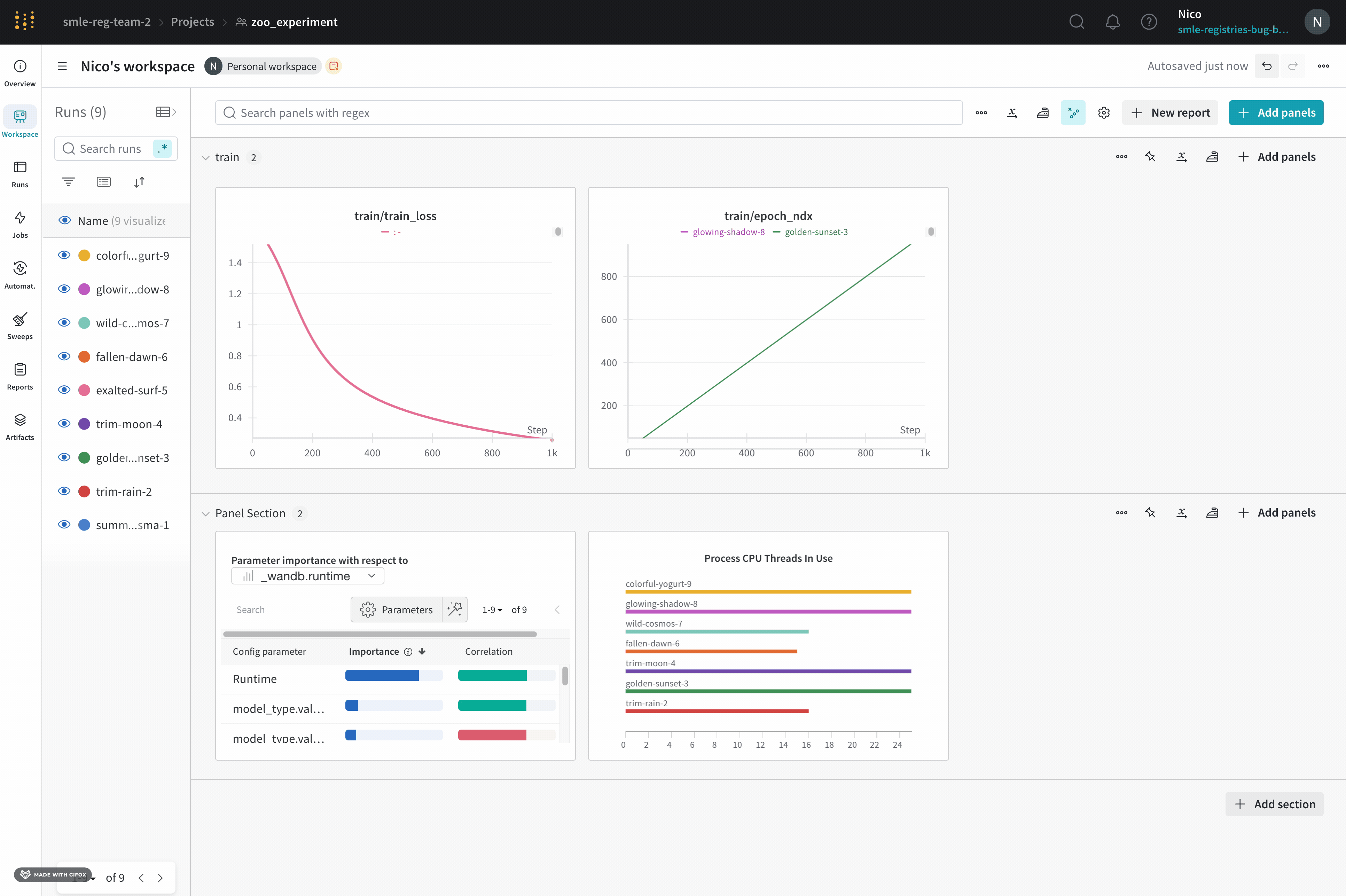
- Select the Add panels button in the top right corner of the page.
- From the left panel that appears, expand the Evaluation dropdown.
- Select Run comparer
Change the color of the line plots
Sometimes the default color of runs is not helpful for comparison. To help overcome this, wandb provides two instances with which one can manually change the colors.
Each run is given a random color by default upon initialization.
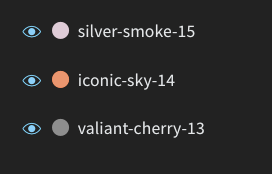
Upon clicking any of the colors, a color palette appears from which we can manually choose the color we want.
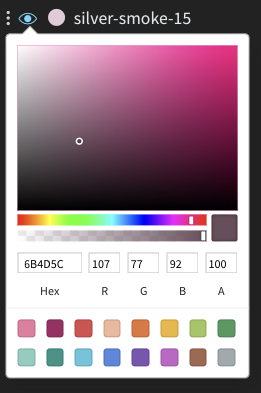
- Hover your mouse over the panel you want to edit its settings for.
- Select the pencil icon that appears.
- Choose the Legend tab.
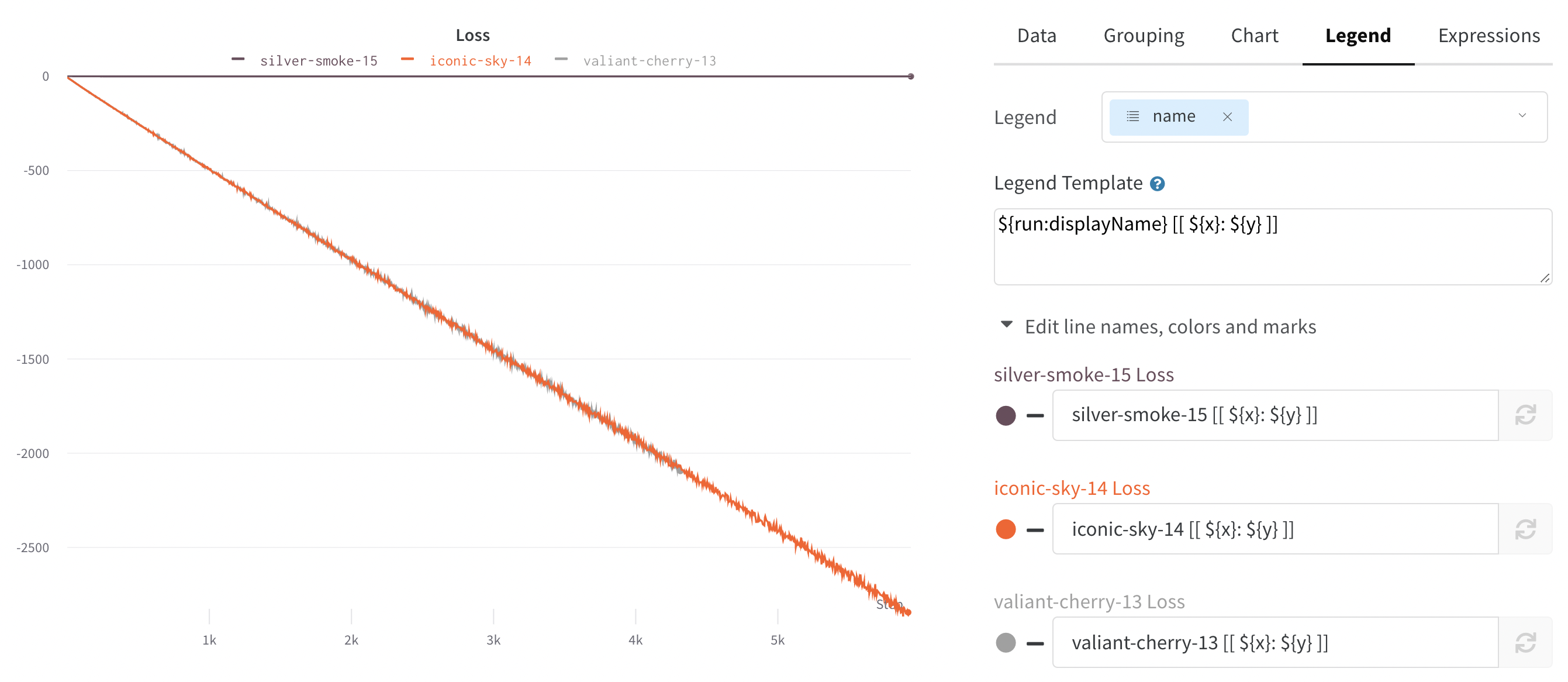
Visualize on different x axes
If you’d like to see the absolute time that an experiment has taken, or see what day an experiment ran, you can switch the x axis. Here’s an example of switching from steps to relative time and then to wall time.
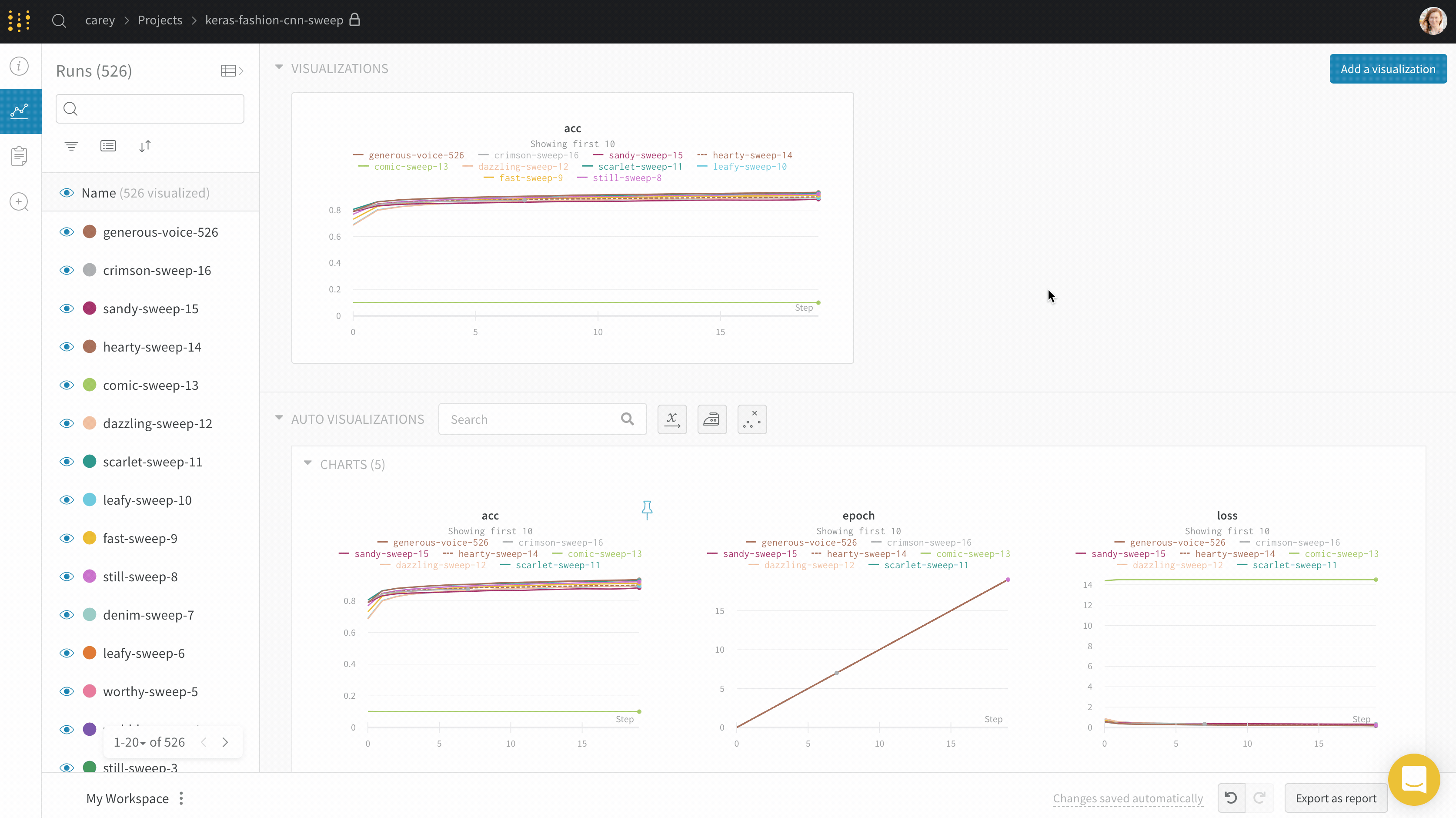
Area plots
In the line plot settings, in the advanced tab, click on different plot styles to get an area plot or a percentage area plot.
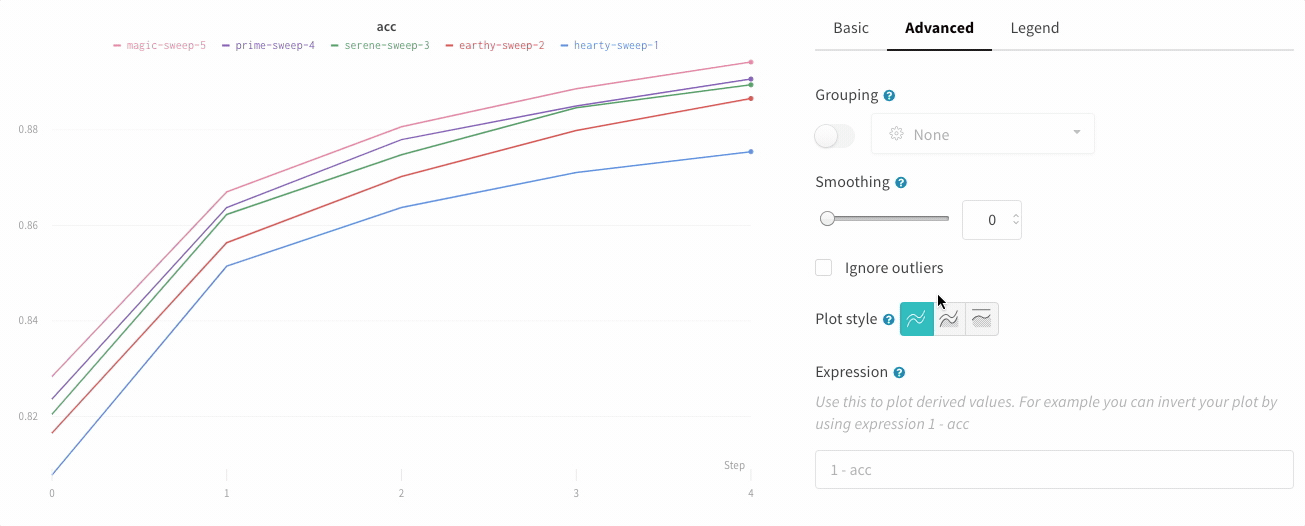
Zoom
Click and drag a rectangle to zoom vertically and horizontally at the same time. This changes the x-axis and y-axis zoom.
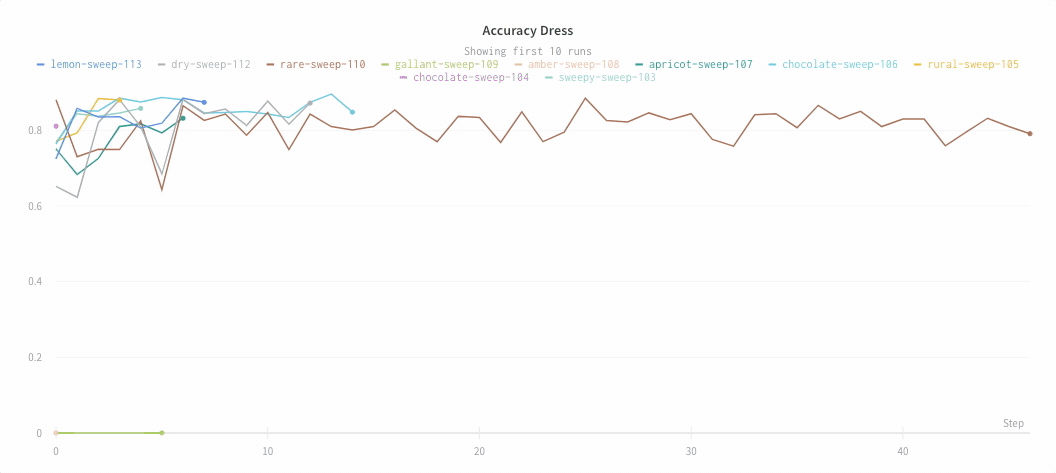
Hide chart legend
Turn off the legend in the line plot with this simple toggle:
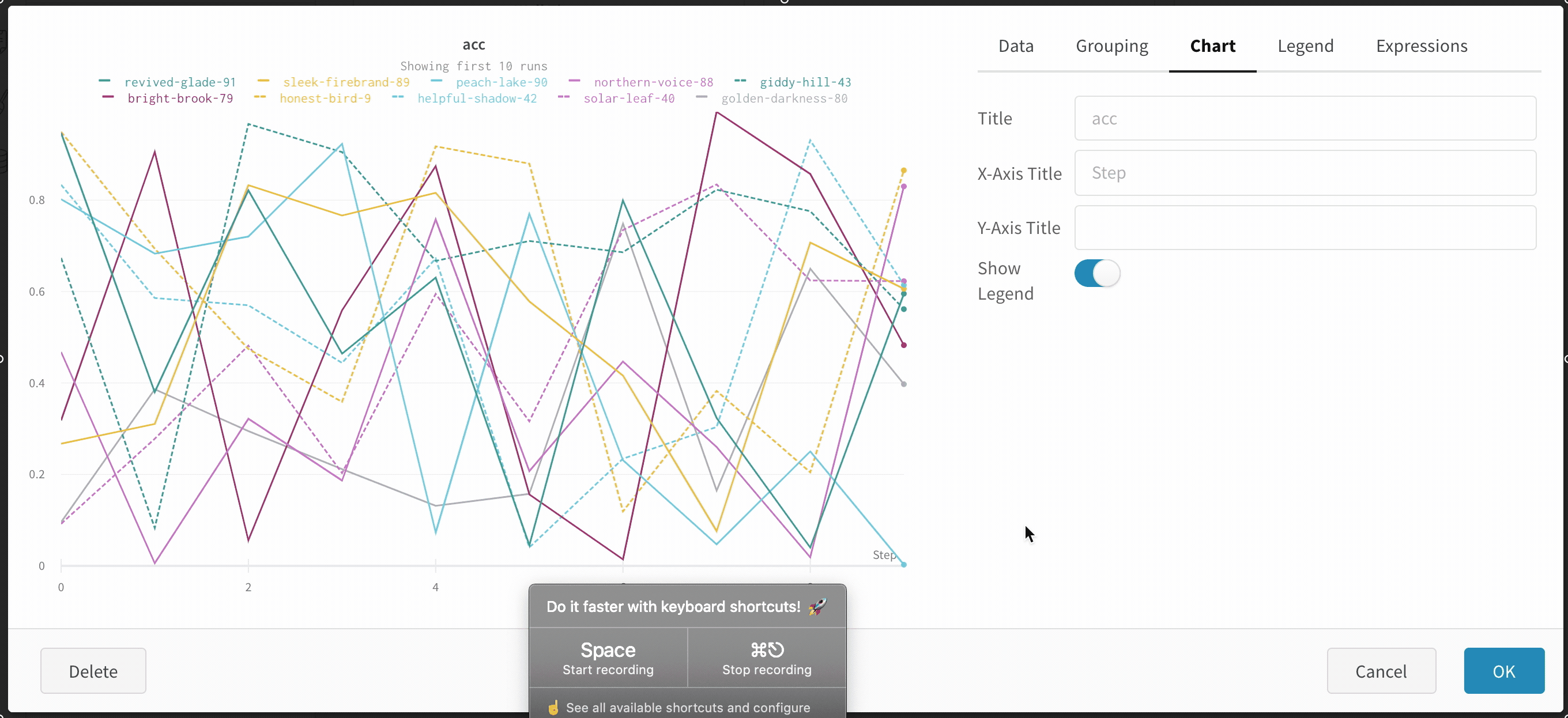
1.1.1 - Line plot reference
X-Axis
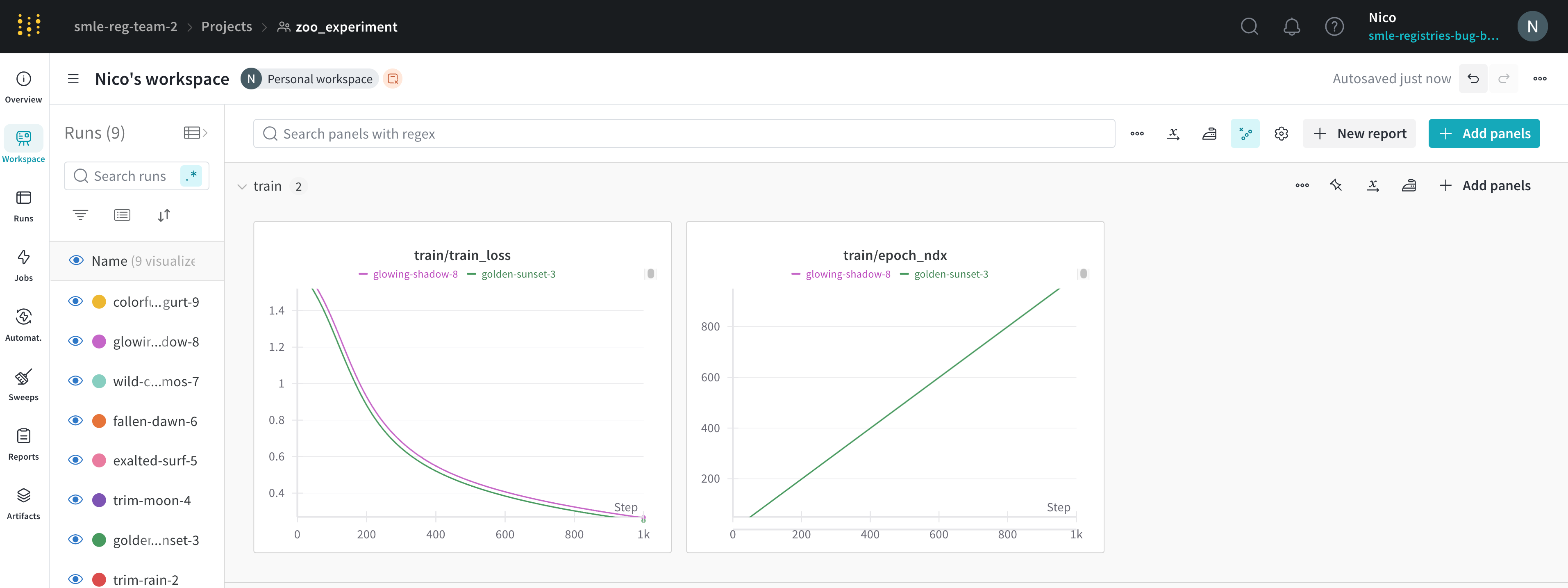
You can set the x-axis of a line plot to any value that you have logged with W&B.log as long as it’s always logged as a number.
Y-Axis variables
You can set the y-axis variables to any value you have logged with wandb.log as long as you were logging numbers, arrays of numbers or a histogram of numbers. If you logged more than 1500 points for a variable, W&B samples down to 1500 points.
X range and Y range
You can change the maximum and minimum values of X and Y for the plot.
X range default is from the smallest value of your x-axis to the largest.
Y range default is from the smallest value of your metrics and zero to the largest value of your metrics.
Max runs/groups
By default you will only plot 10 runs or groups of runs. The runs will be taken from the top of your runs table or run set, so if you sort your runs table or run set you can change the runs that are shown.
Legend
You can control the legend of your chart to show for any run any config value that you logged and meta data from the runs such as the created at time or the user who created the run.
Example:
${run:displayName} - ${config:dropout}
will make the legend name for each run something like royal-sweep - 0.5
where royal-sweep
is the run name and 0.5
is the config parameter named dropout
.
You can set value inside[[ ]]
to display point specific values in the crosshair when hovering over a chart. For example \[\[ $x: $y ($original) ]]
would display something like “2: 3 (2.9)”
Supported values inside [[ ]]
are as follows:
Value | Meaning |
---|---|
${x} |
X value |
${y} |
Y value (Including smoothing adjustment) |
${original} |
Y value not including smoothing adjustment |
${mean} |
Mean of grouped runs |
${stddev} |
Standard Deviation of grouped runs |
${min} |
Min of grouped runs |
${max} |
Max of grouped runs |
${percent} |
Percent of total (for stacked area charts) |
Grouping
You can aggregate all of the runs by turning on grouping, or group over an individual variable. You can also turn on grouping by grouping inside the table and the groups will automatically populate into the graph.
Smoothing
You can set the smoothing coefficient to be between 0 and 1 where 0 is no smoothing and 1 is maximum smoothing.
Ignore outliers
Rescale the plot to exclude outliers from the default plot min and max scale. The setting’s impact on the plot depends on the plot’s sampling mode.
- For plots that use random sampling mode, when you enable Ignore outliers, only points from 5% to 95% are shown. When outliers are shown, they are not formatted differently from other points.
- For plots that use full fidelity mode, all points are always shown, condensed down to the last value in each bucket. When Ignore outliers is enabled, the minimum and maximum bounds of each bucket are shaded. Otherwise, no area is shaded.
Expression
Expression lets you plot values derived from metrics like 1-accuracy. It currently only works if you are plotting a single metric. You can do simple arithmetic expressions, +, -, *, / and % as well as ** for powers.
Plot style
Select a style for your line plot.
Line plot:
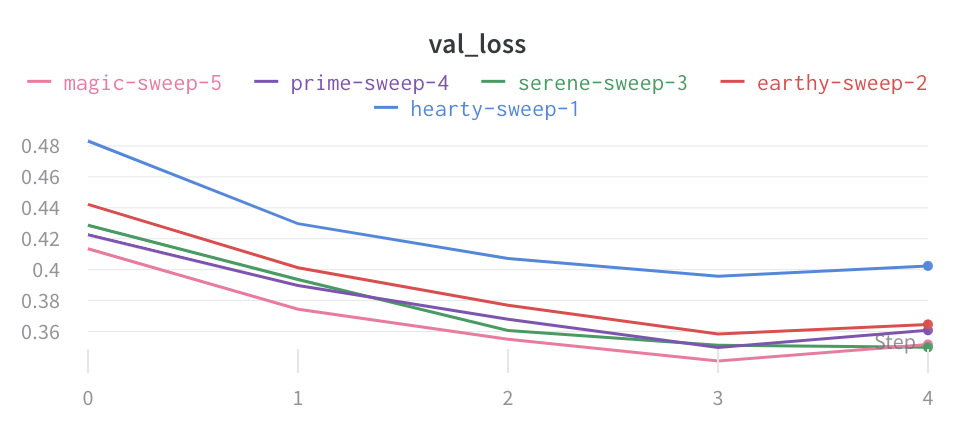
Area plot:
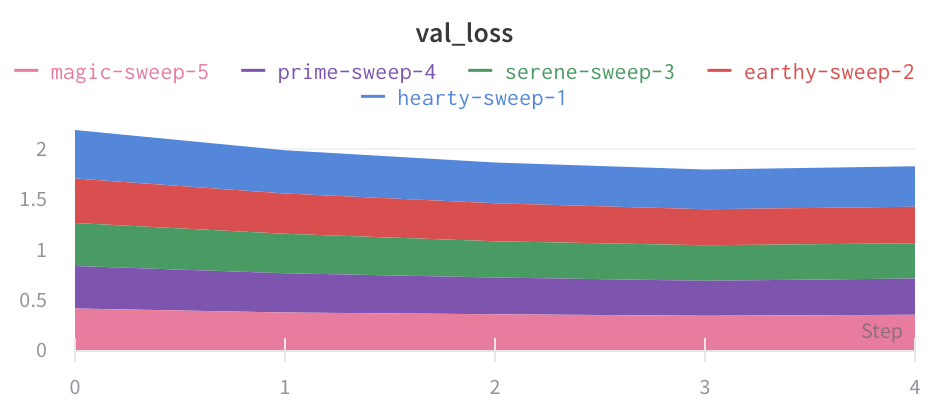
Percentage area plot:
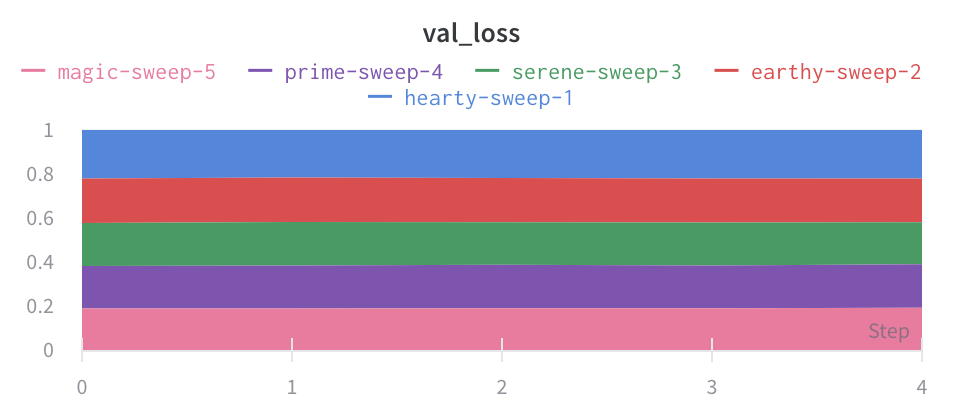
1.1.2 - Point aggregation
Use point aggregation methods within your line plots for improved data visualization accuracy and performance. There are two types of point aggregation modes: full fidelity and random sampling. W&B uses full fidelity mode by default.
Full fidelity
When you use full fidelity mode, W&B breaks the x-axis into dynamic buckets based on the number of data points. It then calculates the minimum, maximum, and average values within each bucket while rendering a point aggregation for the line plot.
There are three main advantages to using full fidelity mode for point aggregation:
- Preserve extreme values and spikes: retain extreme values and spikes in your data
- Configure how minimum and maximum points render: use the W&B App to interactively decide whether you want to show extreme (min/max) values as a shaded area.
- Explore your data without losing data fidelity: W&B recalculates x-axis bucket sizes when you zoom into specific data points. This helps ensure that you can explore your data without losing accuracy. Caching is used to store previously computed aggregations to help reduce loading times which is particularly useful if you are navigating through large datasets.
Configure how minimum and maximum points render
Show or hide minimum and maximum values with shaded areas around your line plots.
The proceeding image shows a blue line plot. The light blue shaded area represents the minimum and maximum values for each bucket.
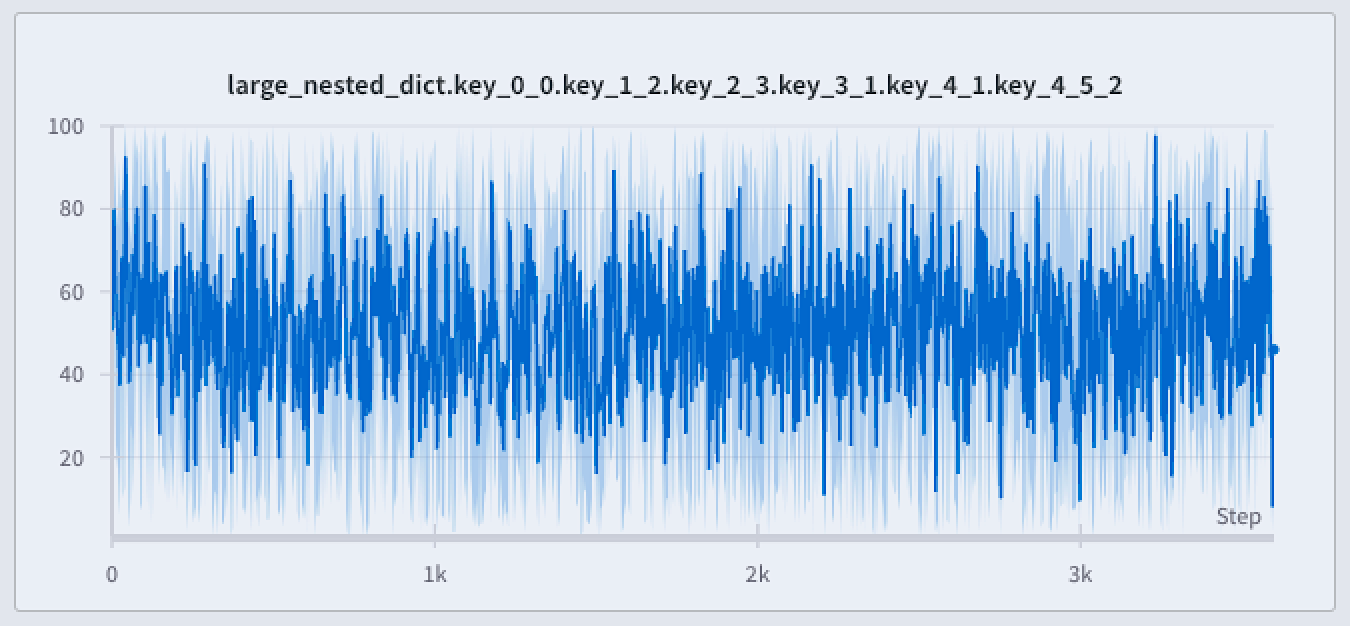
There are three ways to render minimum and maximum values in your line plots:
- Never: The min/max values are not displayed as a shaded area. Only show the aggregated line across the x-axis bucket.
- On hover: The shaded area for min/max values appears dynamically when you hover over the chart. This option keeps the view uncluttered while allowing you to inspect ranges interactively.
- Always: The min/max shaded area is consistently displayed for every bucket in the chart, helping you visualize the full range of values at all times. This can introduce visual noise if there are many runs visualized in the chart.
By default, the minimum and maximum values are not displayed as shaded areas. To view one of the shaded area options, follow these steps:
- Navigate to your W&B project
- Select on the Workspace icon on the left tab
- Select the gear icon on the top right corner of the screen next to the left of the Add panels button.
- From the UI slider that appears, select Line plots
- Within the Point aggregation section, choose On over or Always from the Show min/max values as a shaded area dropdown menu.
- Navigate to your W&B project
- Select on the Workspace icon on the left tab
- Select the line plot panel you want to enable full fidelity mode for
- Within the modal that appears, select On hover or Always from the Show min/max values as a shaded area dropdown menu.
Explore your data without losing data fidelity
Analyze specific regions of the dataset without missing critical points like extreme values or spikes. When you zoom in on a line plot, W&B adjusts the buckets sizes used to calculate the minimum, maximum, and average values within each bucket.
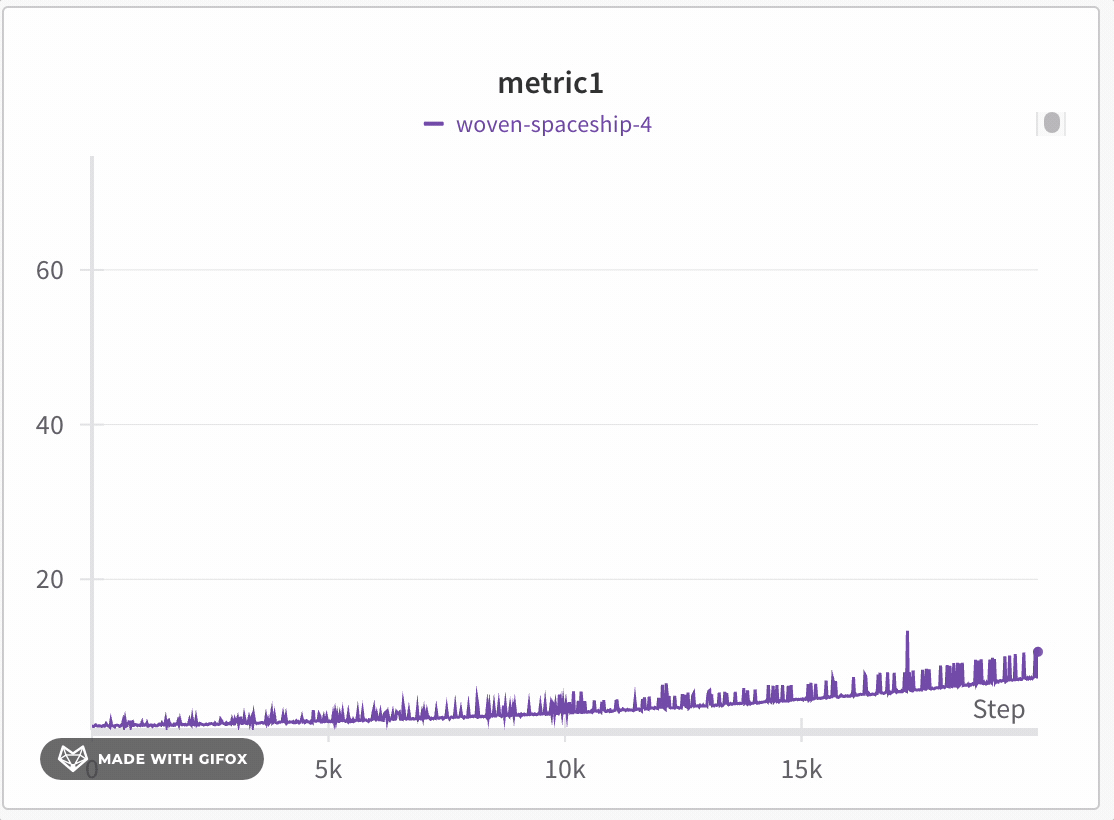
W&B divides the x-axis is dynamically into 1000 buckets by default. For each bucket, W&B calculates the following values:
- Minimum: The lowest value in that bucket.
- Maximum: The highest value in that bucket.
- Average: The mean value of all points in that bucket.
W&B plots values in buckets in a way that preserves full data representation and includes extreme values in every plot. When zoomed in to 1,000 points or fewer, full fidelity mode renders every data point without additional aggregation.
To zoom in on a line plot, follow these steps:
- Navigate to your W&B project
- Select on the Workspace icon on the left tab
- Optionally add a line plot panel to your workspace or navigate to an existing line plot panel.
- Click and drag to select a specific region to zoom in on.
Line plot grouping and expressions
When you use Line Plot Grouping, W&B applies the following based on the mode selected:
- Non-windowed sampling (grouping): Aligns points across runs on the x-axis. The average is taken if multiple points share the same x-value; otherwise, they appear as discrete points.
- Windowed sampling (grouping and expressions): Divides the x-axis either into 250 buckets or the number of points in the longest line (whichever is smaller). W&B takes an average of points within each bucket.
- Full fidelity (grouping and expressions): Similar to non-windowed sampling, but fetches up to 500 points per run to balance performance and detail.
Random sampling
Random sampling uses 1500 randomly sampled points to render line plots. Random sampling is useful for performance reasons when you have a large number of data points.
Enable random sampling
By default, W&B uses full fidelity mode. To enable random sampling, follow these steps:
- Navigate to your W&B project
- Select on the Workspace icon on the left tab
- Select the gear icon on the top right corner of the screen next to the left of the Add panels button.
- From the UI slider that appears, select Line plots
- Choose Random sampling from the Point aggregation section
- Navigate to your W&B project
- Select on the Workspace icon on the left tab
- Select the line plot panel you want to enable random sampling for
- Within the modal that appears, select Random sampling from the Point aggregation method section
Access non sampled data
You can access the complete history of metrics logged during a run using the W&B Run API. The following example demonstrates how to retrieve and process the loss values from a specific run:
# Initialize the W&B API
run = api.run("l2k2/examples-numpy-boston/i0wt6xua")
# Retrieve the history of the 'Loss' metric
history = run.scan_history(keys=["Loss"])
# Extract the loss values from the history
losses = [row["Loss"] for row in history]
1.1.3 - Smooth line plots
W&B supports three types of smoothing:
- exponential moving average (default)
- gaussian smoothing
- running average
- exponential moving average - Tensorboard (deprecated)
See these live in an interactive W&B report.
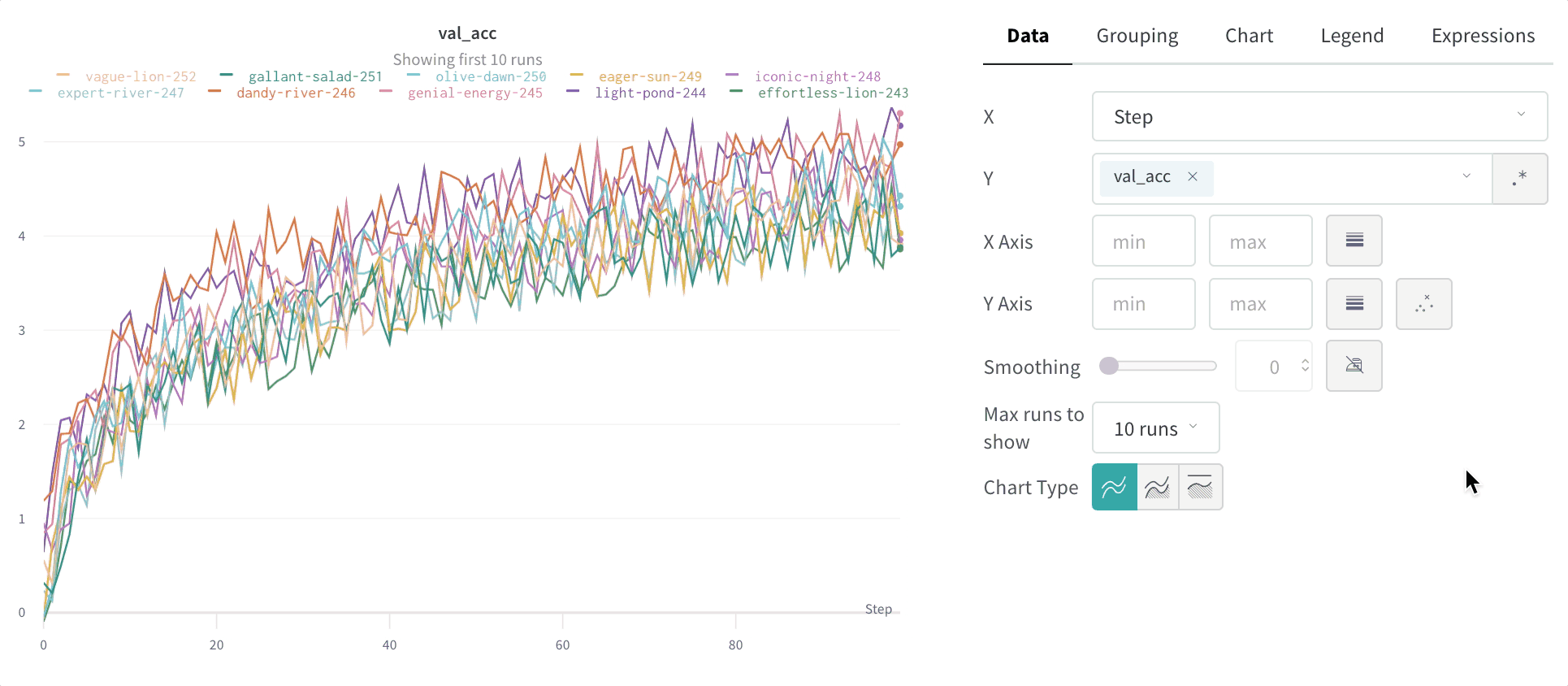
Exponential Moving Average (Default)
Exponential smoothing is a technique for smoothing time series data by exponentially decaying the weight of previous points. The range is 0 to 1. See Exponential Smoothing for background. There is a de-bias term added so that early values in the time series are not biased towards zero.
The EMA algorithm takes the density of points on the line (the number of y
values per unit of range on x-axis) into account. This allows consistent smoothing when displaying multiple lines with different characteristics simultaneously.
Here is sample code for how this works under the hood:
const smoothingWeight = Math.min(Math.sqrt(smoothingParam || 0), 0.999);
let lastY = yValues.length > 0 ? 0 : NaN;
let debiasWeight = 0;
return yValues.map((yPoint, index) => {
const prevX = index > 0 ? index - 1 : 0;
// VIEWPORT_SCALE scales the result to the chart's x-axis range
const changeInX =
((xValues[index] - xValues[prevX]) / rangeOfX) * VIEWPORT_SCALE;
const smoothingWeightAdj = Math.pow(smoothingWeight, changeInX);
lastY = lastY * smoothingWeightAdj + yPoint;
debiasWeight = debiasWeight * smoothingWeightAdj + 1;
return lastY / debiasWeight;
});
Here’s what this looks like in the app:
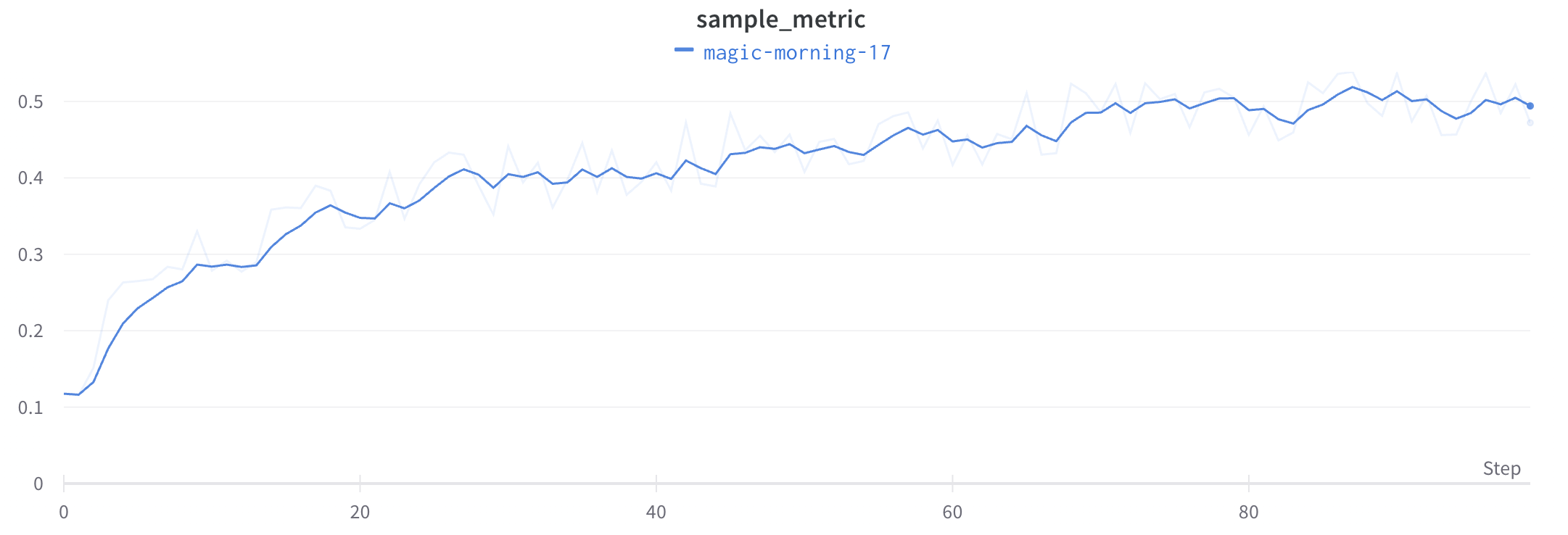
Gaussian Smoothing
Gaussian smoothing (or gaussian kernel smoothing) computes a weighted average of the points, where the weights correspond to a gaussian distribution with the standard deviation specified as the smoothing parameter. See . The smoothed value is calculated for every input x value.
Gaussian smoothing is a good standard choice for smoothing if you are not concerned with matching TensorBoard’s behavior. Unlike an exponential moving average the point will be smoothed based on points occurring both before and after the value.
Here’s what this looks like in the app:
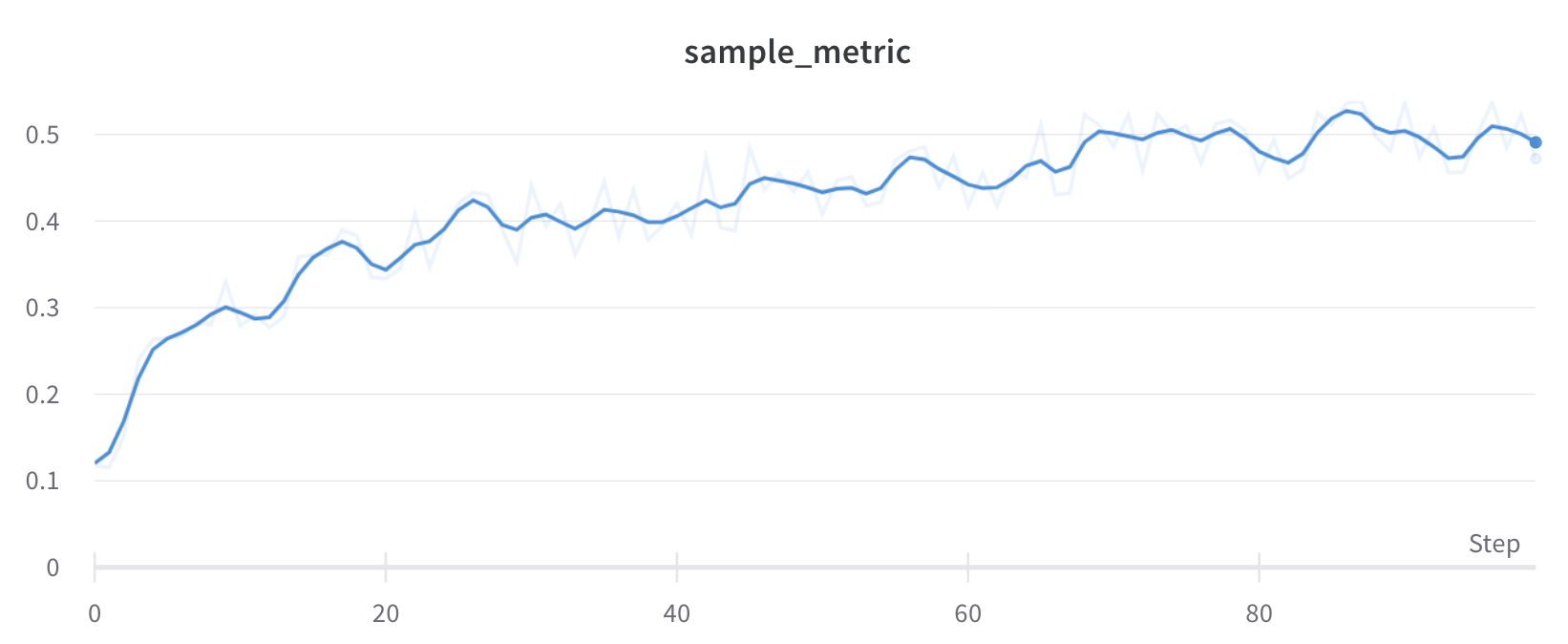
Running Average
Running average is a smoothing algorithm that replaces a point with the average of points in a window before and after the given x value. See “Boxcar Filter” at https://en.wikipedia.org/wiki/Moving_average. The selected parameter for running average tells Weights and Biases the number of points to consider in the moving average.
Consider using Gaussian Smoothing if your points are spaced unevenly on the x-axis.
The following image demonstrates how a running app looks like in the app:
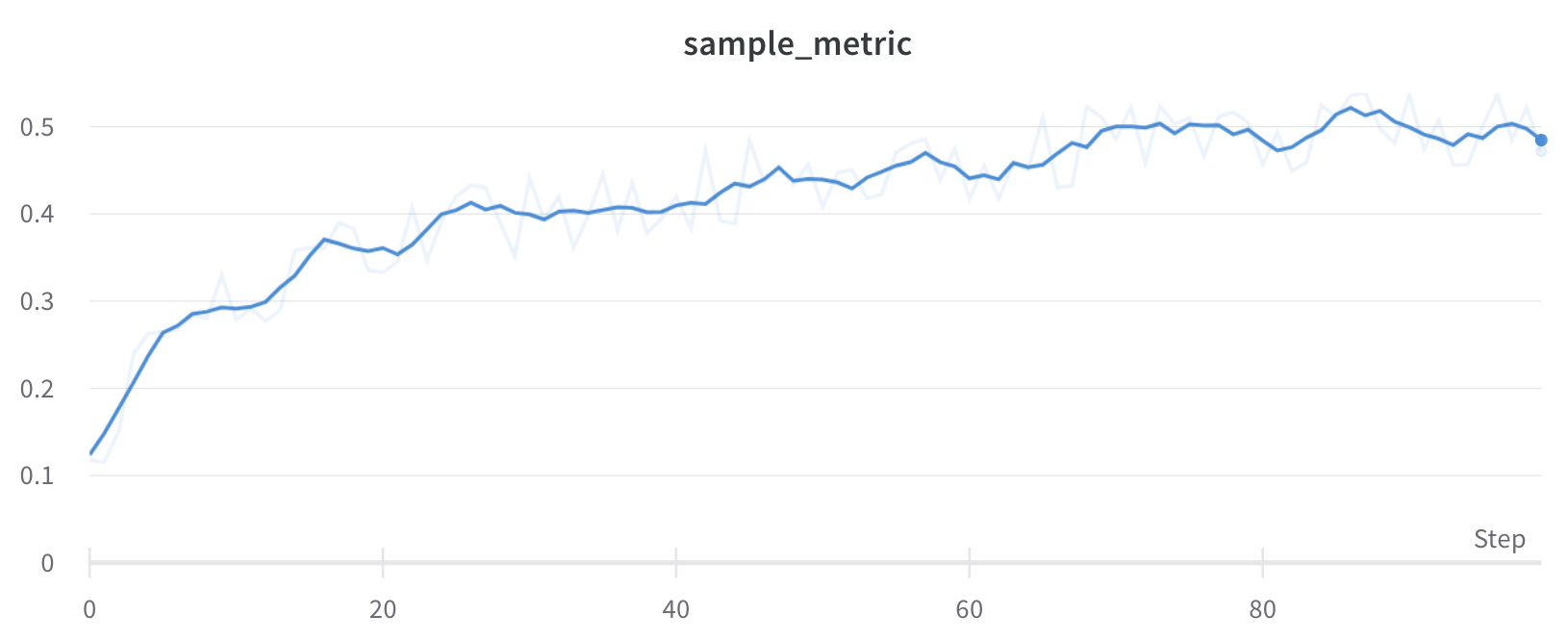
Exponential Moving Average (Deprecated)
The TensorBoard EMA algorithm has been deprecated as it cannot accurately smooth multiple lines on the same chart that do not have a consistent point density (number of points plotted per unit of x-axis).
Exponential moving average is implemented to match TensorBoard’s smoothing algorithm. The range is 0 to 1. See Exponential Smoothing for background. There is a debias term added so that early values in the time series are not biases towards zero.
Here is sample code for how this works under the hood:
data.forEach(d => {
const nextVal = d;
last = last * smoothingWeight + (1 - smoothingWeight) * nextVal;
numAccum++;
debiasWeight = 1.0 - Math.pow(smoothingWeight, numAccum);
smoothedData.push(last / debiasWeight);
Here’s what this looks like in the app:
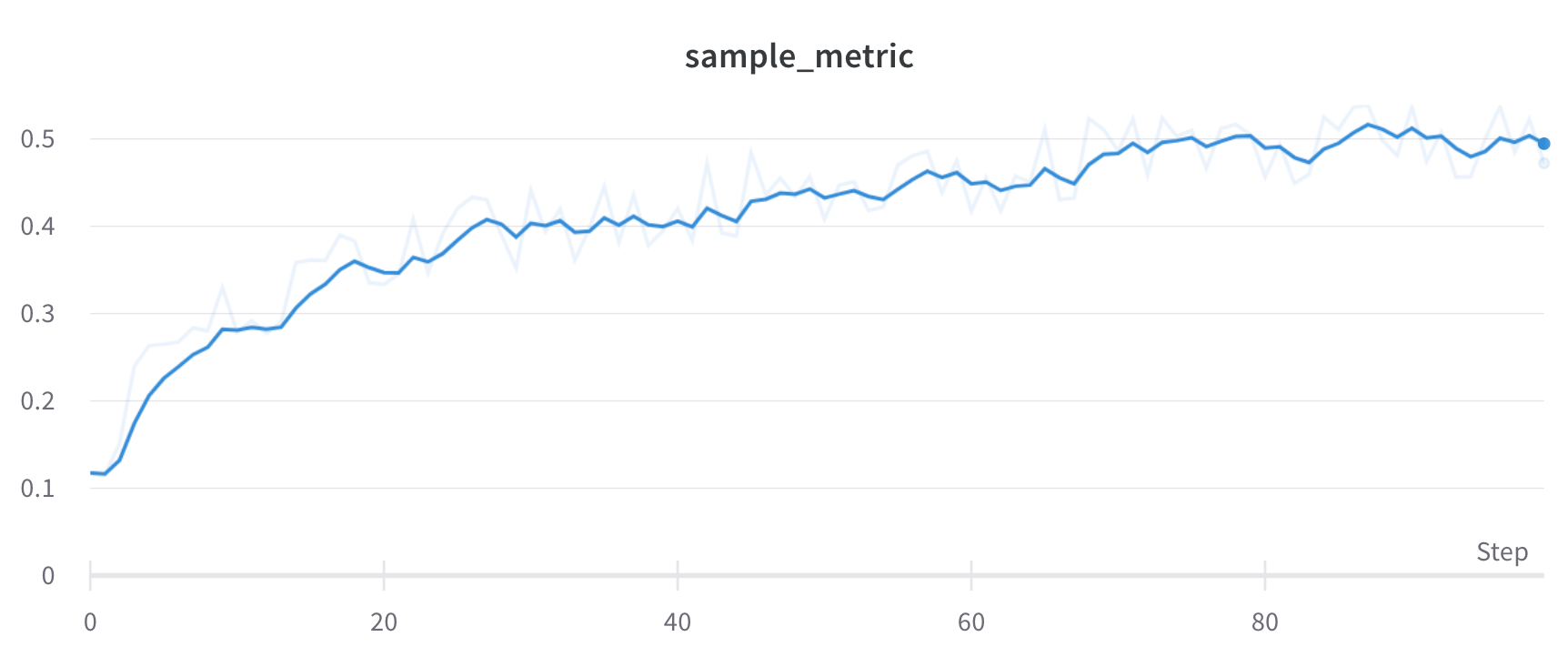
Implementation Details
All of the smoothing algorithms run on the sampled data, meaning that if you log more than 1500 points, the smoothing algorithm will run after the points are downloaded from the server. The intention of the smoothing algorithms is to help find patterns in data quickly. If you need exact smoothed values on metrics with a large number of logged points, it may be better to download your metrics through the API and run your own smoothing methods.
Hide original data
By default we show the original, unsmoothed data as a faint line in the background. Click the Show Original toggle to turn this off.
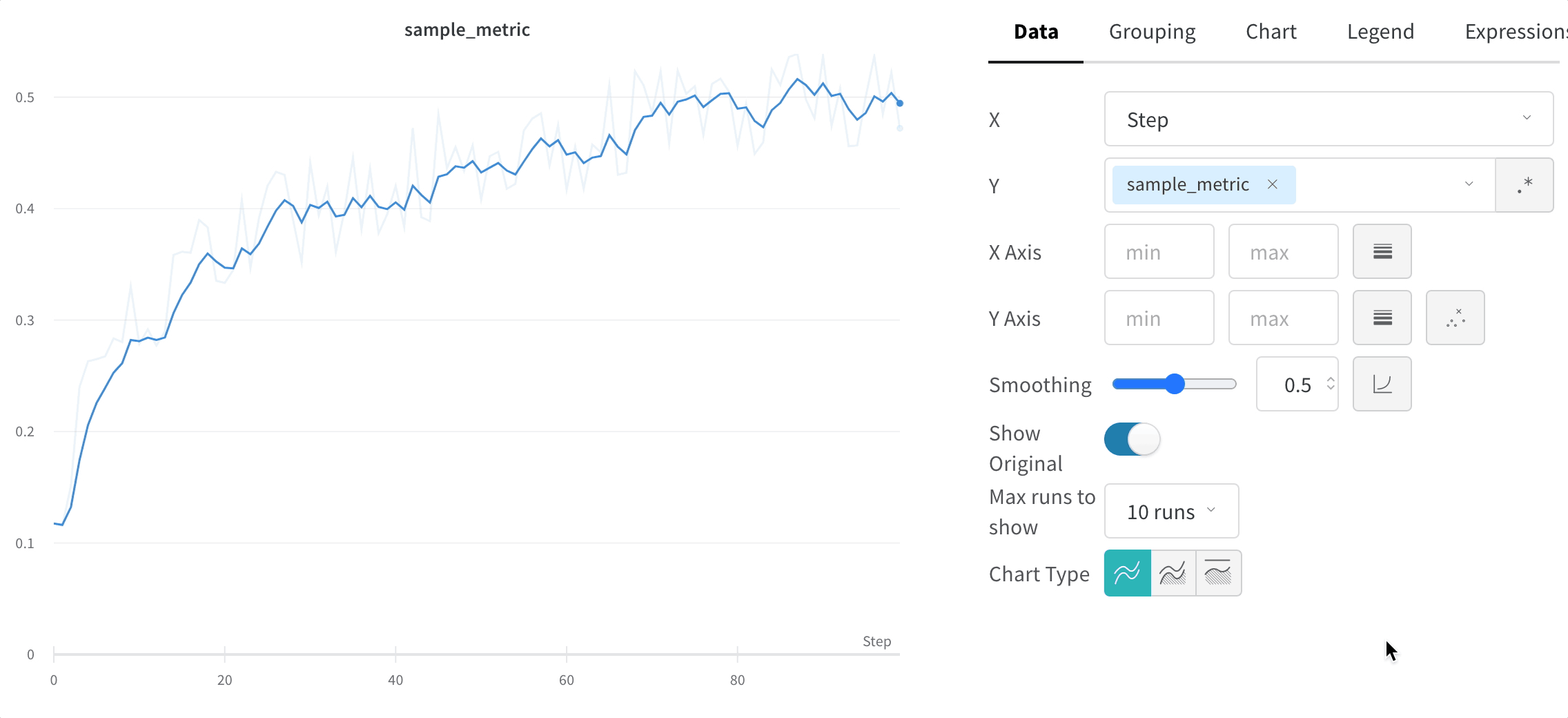
1.2 - Bar plots
A bar plot presents categorical data with rectangular bars which can be plotted vertically or horizontally. Bar plots show up by default with wandb.log() when all logged values are of length one.
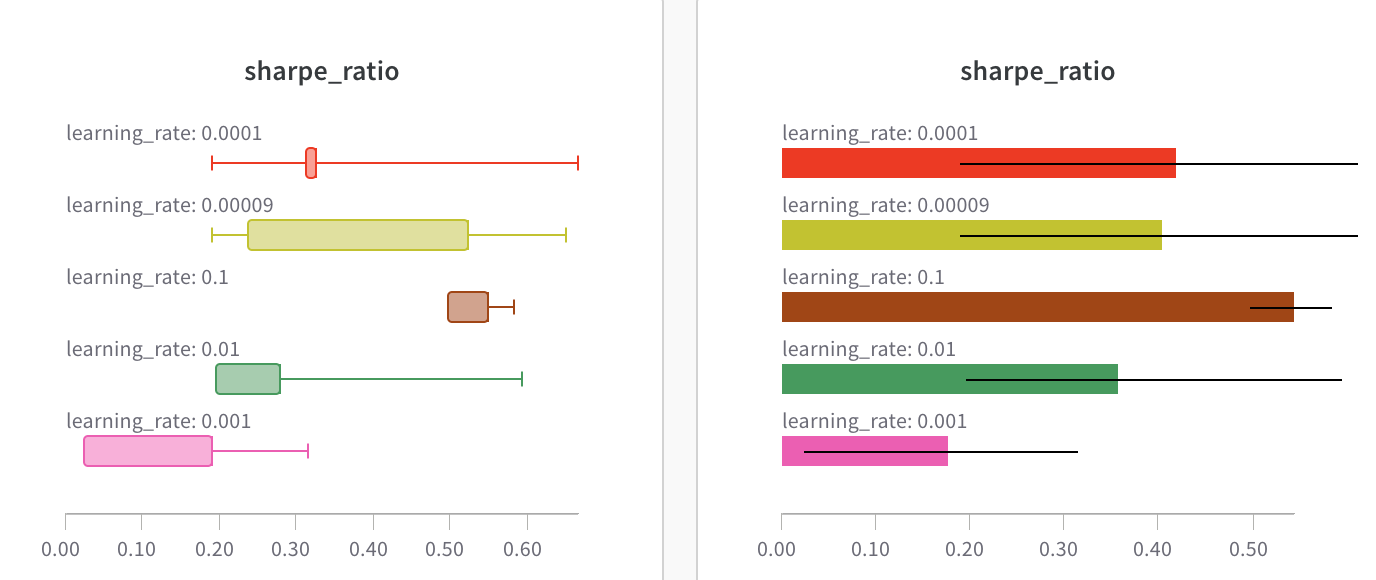
Customize with chart settings to limit max runs to show, group runs by any config and rename labels.
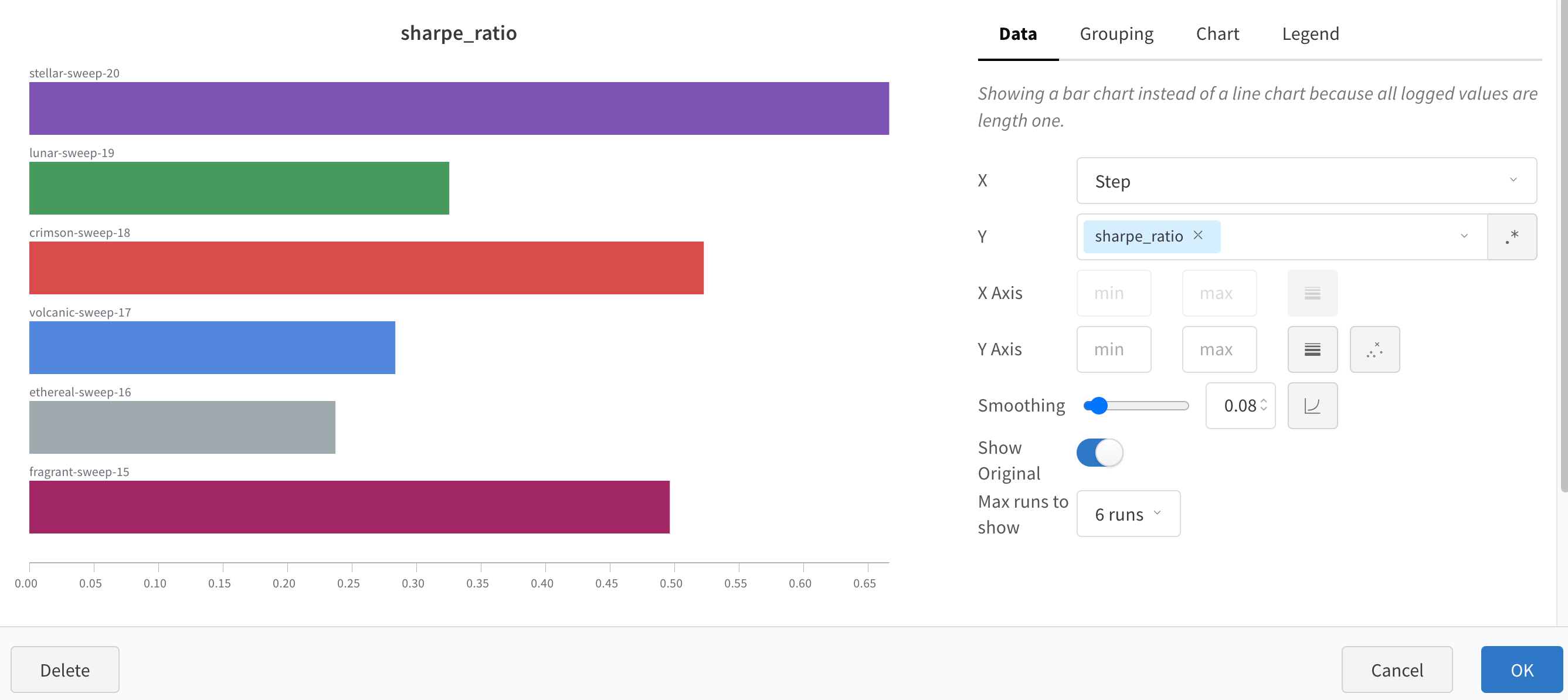
Customize bar plots
You can also create Box or Violin Plots to combine many summary statistics into one chart type**.**
- Group runs via runs table.
- Click ‘Add panel’ in the workspace.
- Add a standard ‘Bar Chart’ and select the metric to plot.
- Under the ‘Grouping’ tab, pick ‘box plot’ or ‘Violin’, etc. to plot either of these styles.
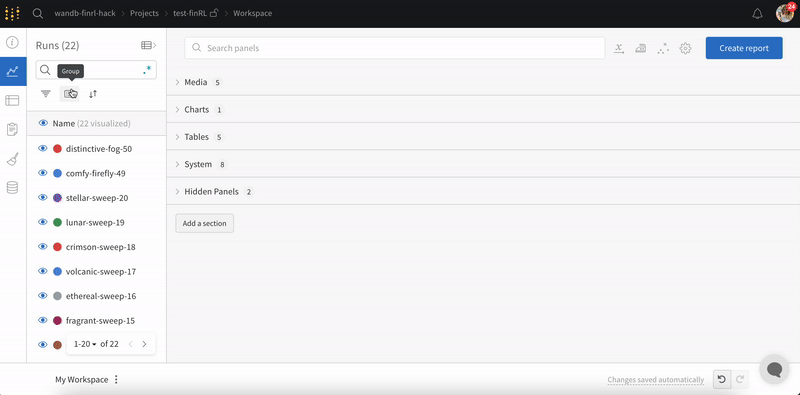
1.3 - Parallel coordinates
Parallel coordinates charts summarize the relationship between large numbers of hyperparameters and model metrics at a glance.
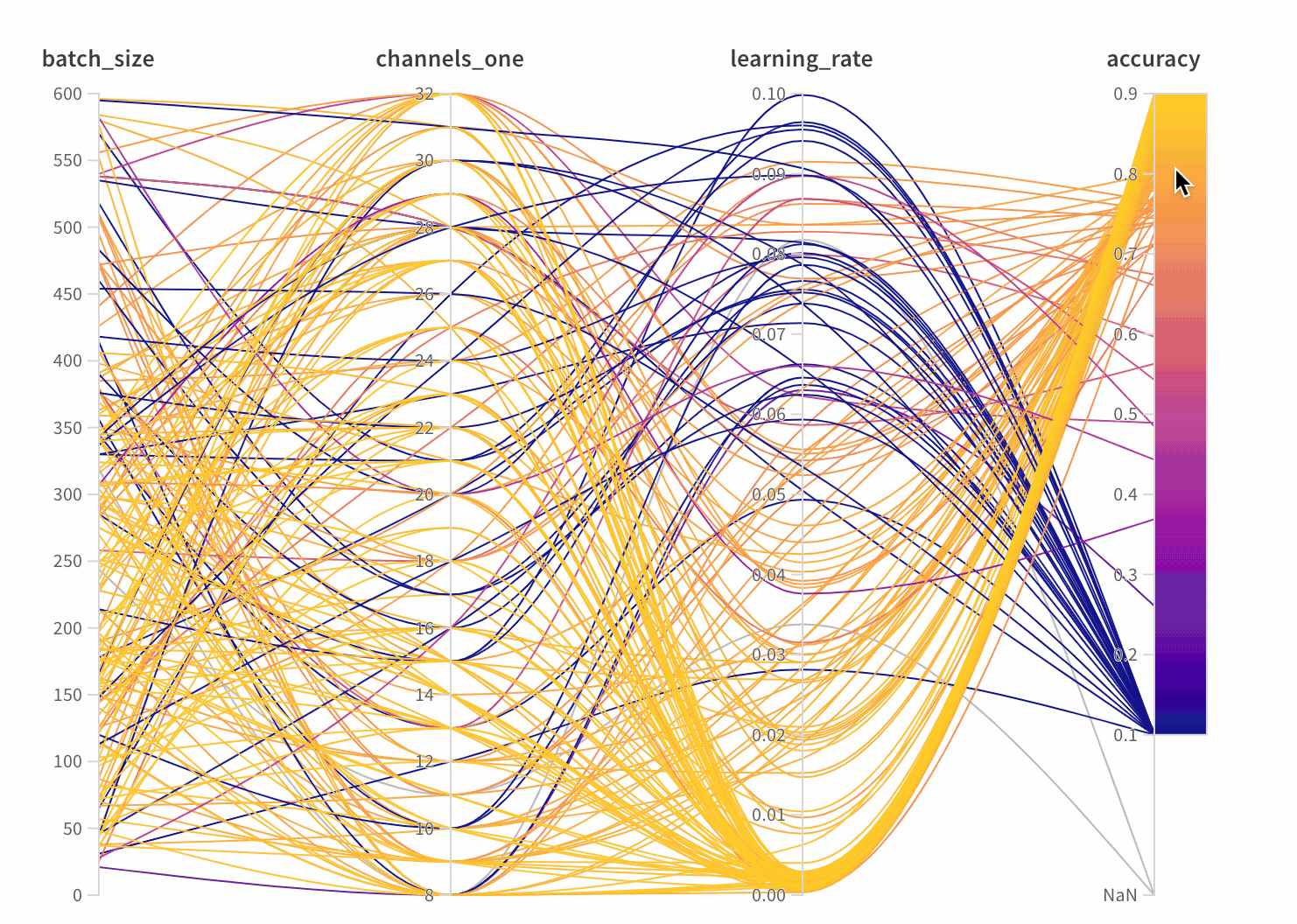
- Axes: Different hyperparameters from
wandb.config
and metrics fromwandb.log
. - Lines: Each line represents a single run. Mouse over a line to see a tooltip with details about the run. All lines that match the current filters will be shown, but if you turn off the eye, lines will be grayed out.
Panel Settings
Configure these features in the panel settings— click the edit button in the upper right corner of the panel.
- Tooltip: On hover, a legend shows up with info on each run
- Titles: Edit the axis titles to be more readable
- Gradient: Customize the gradient to be any color range you like
- Log scale: Each axis can be set to view on a log scale independently
- Flip axis: Switch the axis direction— this is useful when you have both accuracy and loss as columns
1.4 - Scatter plots
Use the scatter plot to compare multiple runs and visualize how your experiments are performing. We’ve added some customizable features:
- Plot a line along the min, max, and average
- Custom metadata tooltips
- Control point colors
- Set axes ranges
- Switch axes to log scale
Here’s an example of validation accuracy of different models over a couple of weeks of experimentation. The tooltip is customized to include the batch size and dropout as well as the values on the axes. There’s also a line plotting the running average of validation accuracy.
See a live example →
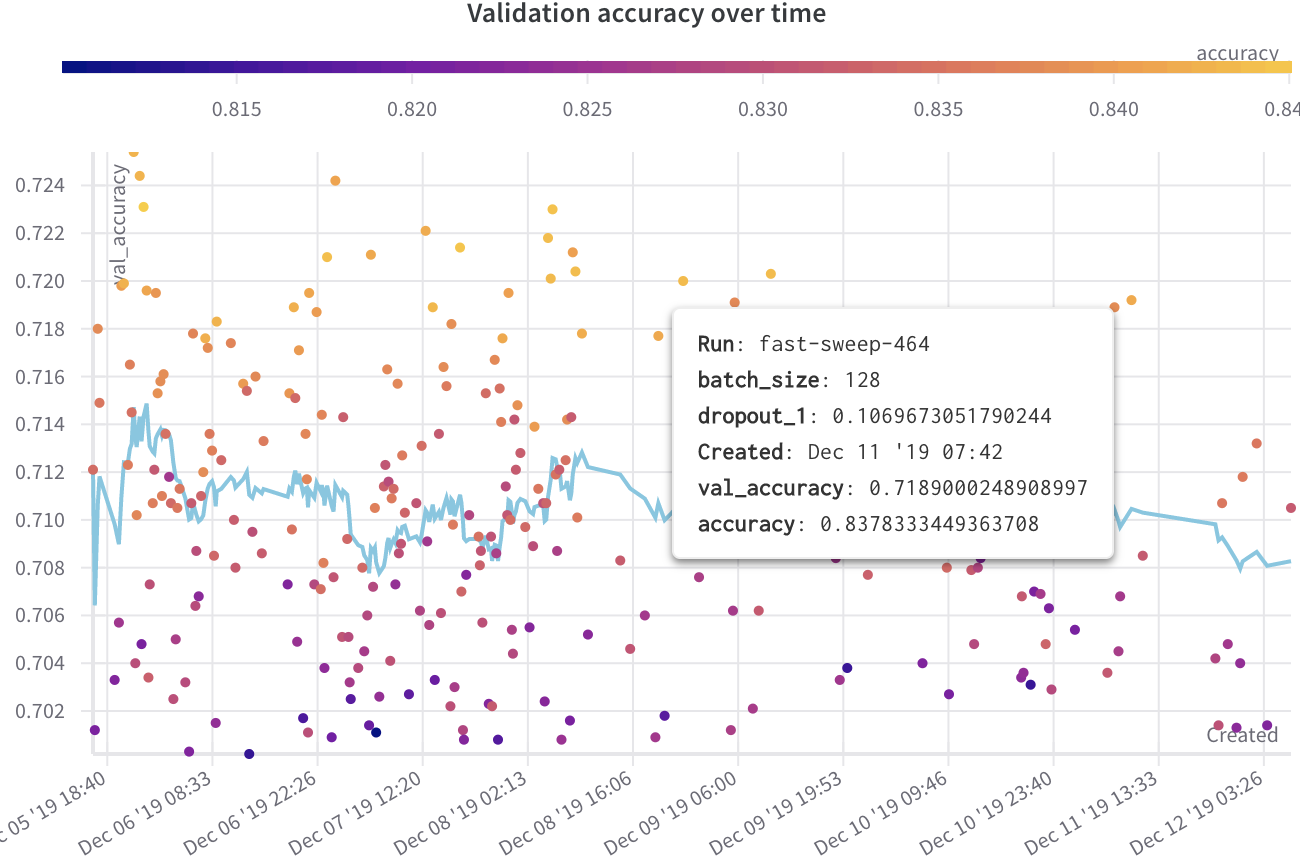
1.5 - Save and diff code
By default, W&B only saves the latest git commit hash. You can turn on more code features to compare the code between your experiments dynamically in the UI.
Starting with wandb
version 0.8.28, W&B can save the code from your main training file where you call wandb.init()
.
Save library code
When you enable code saving, W&B saves the code from the file that called wandb.init()
. To save additional library code, you have three options:
Call wandb.run.log_code(".")
after calling wandb.init()
import wandb
wandb.init()
wandb.run.log_code(".")
Pass a settings object to wandb.init
with code_dir
set
import wandb
wandb.init(settings=wandb.Settings(code_dir="."))
This captures all python source code files in the current directory and all subdirectories as an artifact. For more control over the types and locations of source code files that are saved, see the reference docs.
Set code saving in the UI
In addition to setting code saving programmatically, you can also toggle this feature in your W&B account Settings. Note that this will enable code saving for all teams associated with your account.
By default, W&B disables code saving for all teams.
- Log in to your W&B account.
- Go to Settings > Privacy.
- Under Project and content security, toggle Disable default code saving on.
Code comparer
Compare code used in different W&B runs:
- Select the Add panels button in the top right corner of the page.
- Expand TEXT AND CODE dropdown and select Code.
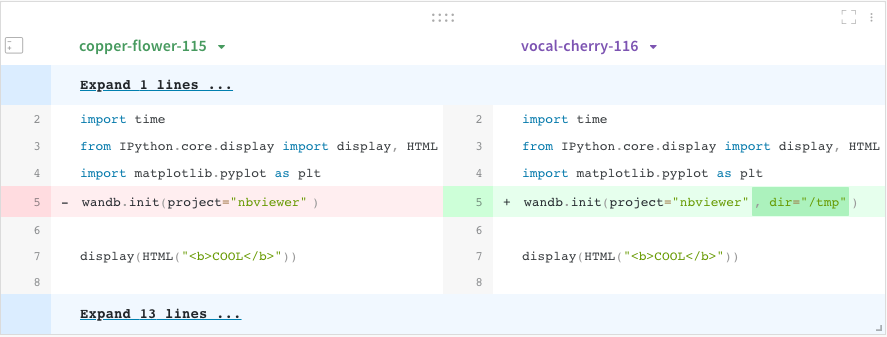
Jupyter session history
W&B saves the history of code executed in your Jupyter notebook session. When you call wandb.init() inside of Jupyter, W&B adds a hook to automatically save a Jupyter notebook containing the history of code executed in your current session.
- Navigate to your project workspaces that contains your code.
- Select the Artifacts tab in the left navigation bar.
- Expand the code artifact.
- Select the Files tab.
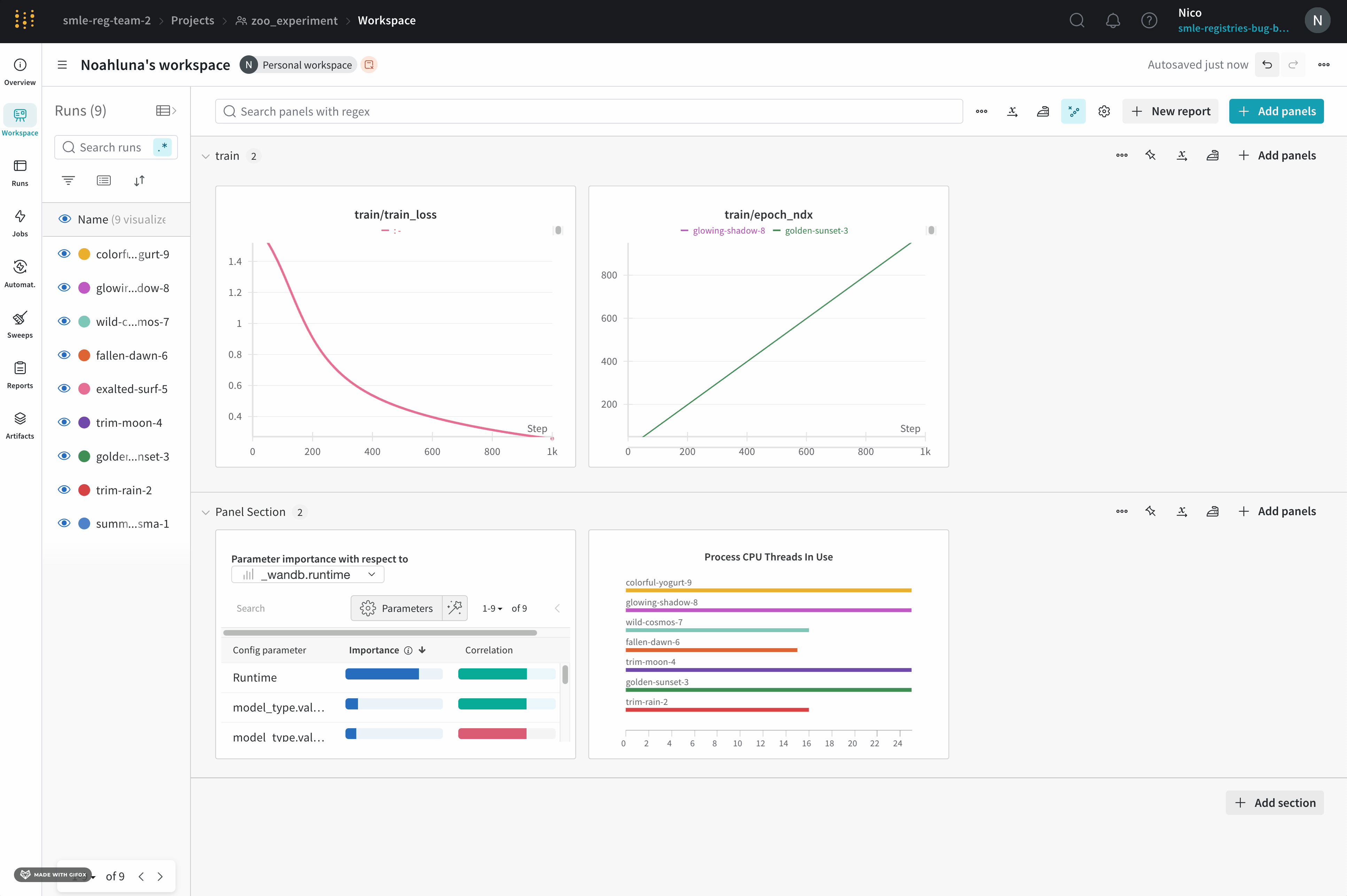
This displays the cells that were run in your session along with any outputs created by calling iPython’s display method. This enables you to see exactly what code was run within Jupyter in a given run. When possible W&B also saves the most recent version of the notebook which you would find in the code directory as well.
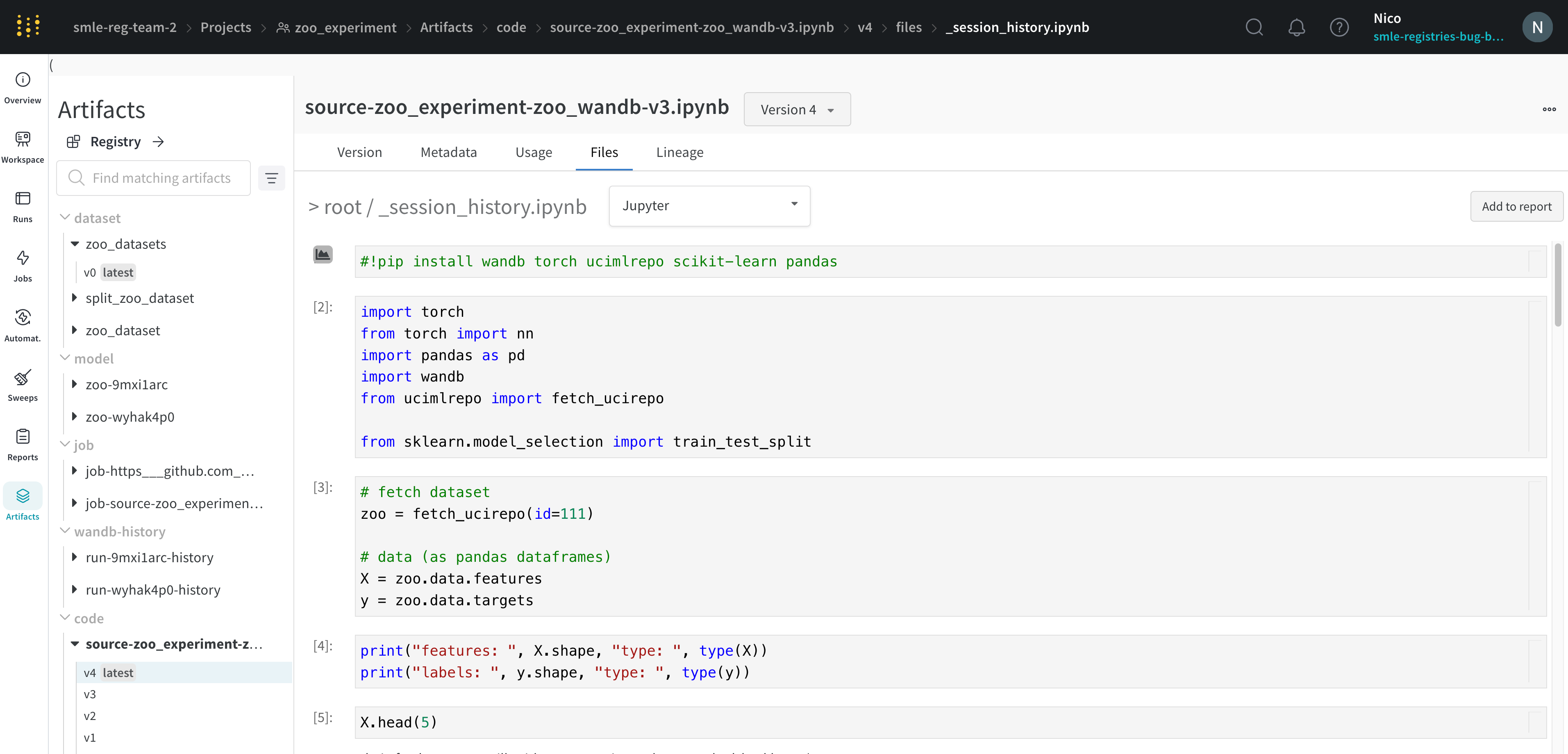
1.6 - Parameter importance
Discover which of your hyperparameters were the best predictors of, and highly correlated to desirable values of your metrics.
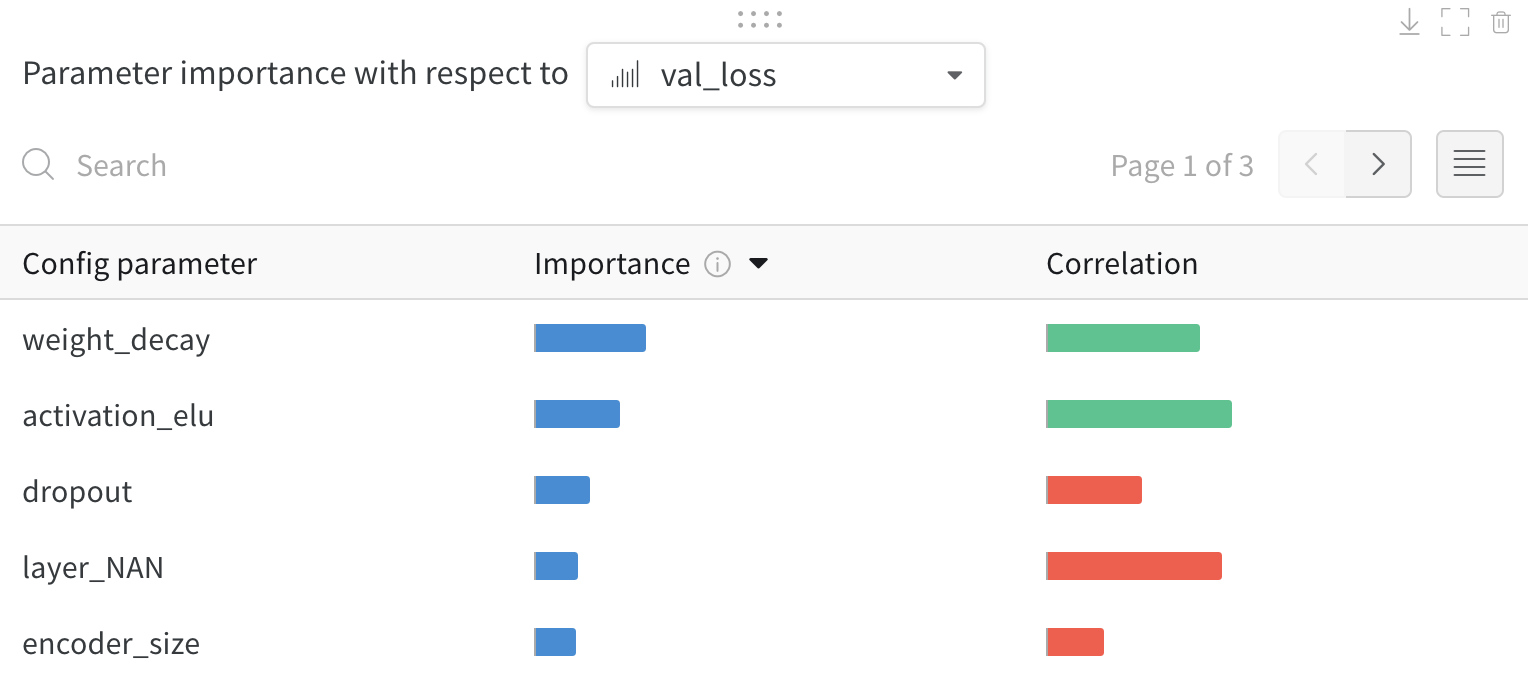
Correlation is the linear correlation between the hyperparameter and the chosen metric (in this case val_loss). So a high correlation means that when the hyperparameter has a higher value, the metric also has higher values and vice versa. Correlation is a great metric to look at but it can’t capture second order interactions between inputs and it can get messy to compare inputs with wildly different ranges.
Therefore W&B also calculates an importance metric. W&B trains a random forest with the hyperparameters as inputs and the metric as the target output and report the feature importance values for the random forest.
The idea for this technique was inspired by a conversation with Jeremy Howard who has pioneered the use of random forest feature importances to explore hyperparameter spaces at Fast.ai. W&B highly recommends you check out this lecture (and these notes) to learn more about the motivation behind this analysis.
Hyperparameter importance panel untangles the complicated interactions between highly correlated hyperparameters. In doing so, it helps you fine tune your hyperparameter searches by showing you which of your hyperparameters matter the most in terms of predicting model performance.
Creating a hyperparameter importance panel
- Navigate to your W&B project.
- Select Add panels buton.
- Expand the CHARTS dropdown, choose Parallel coordinates from the dropdown.
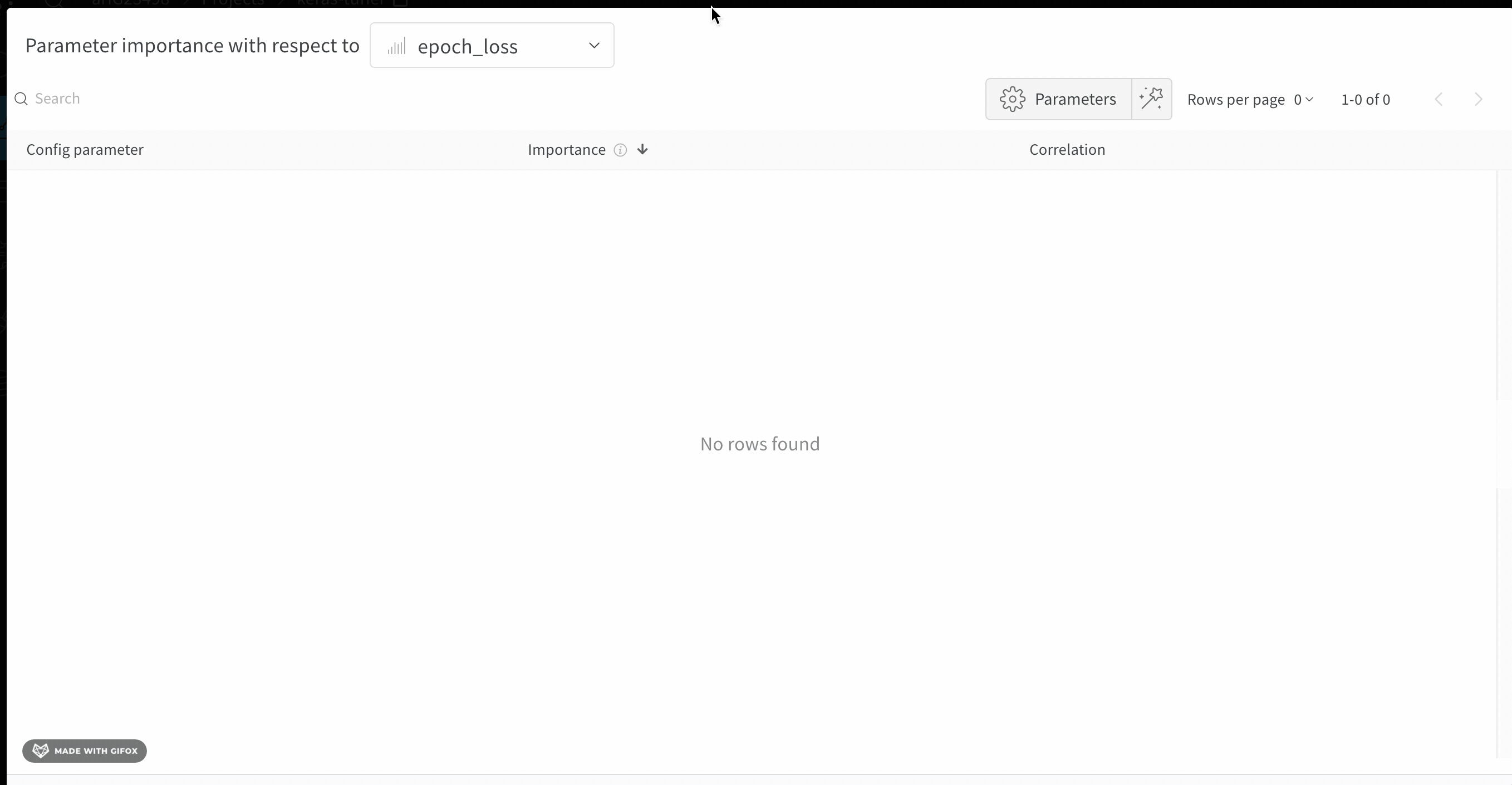
With the parameter manager, we can manually set the visible and hidden parameters.
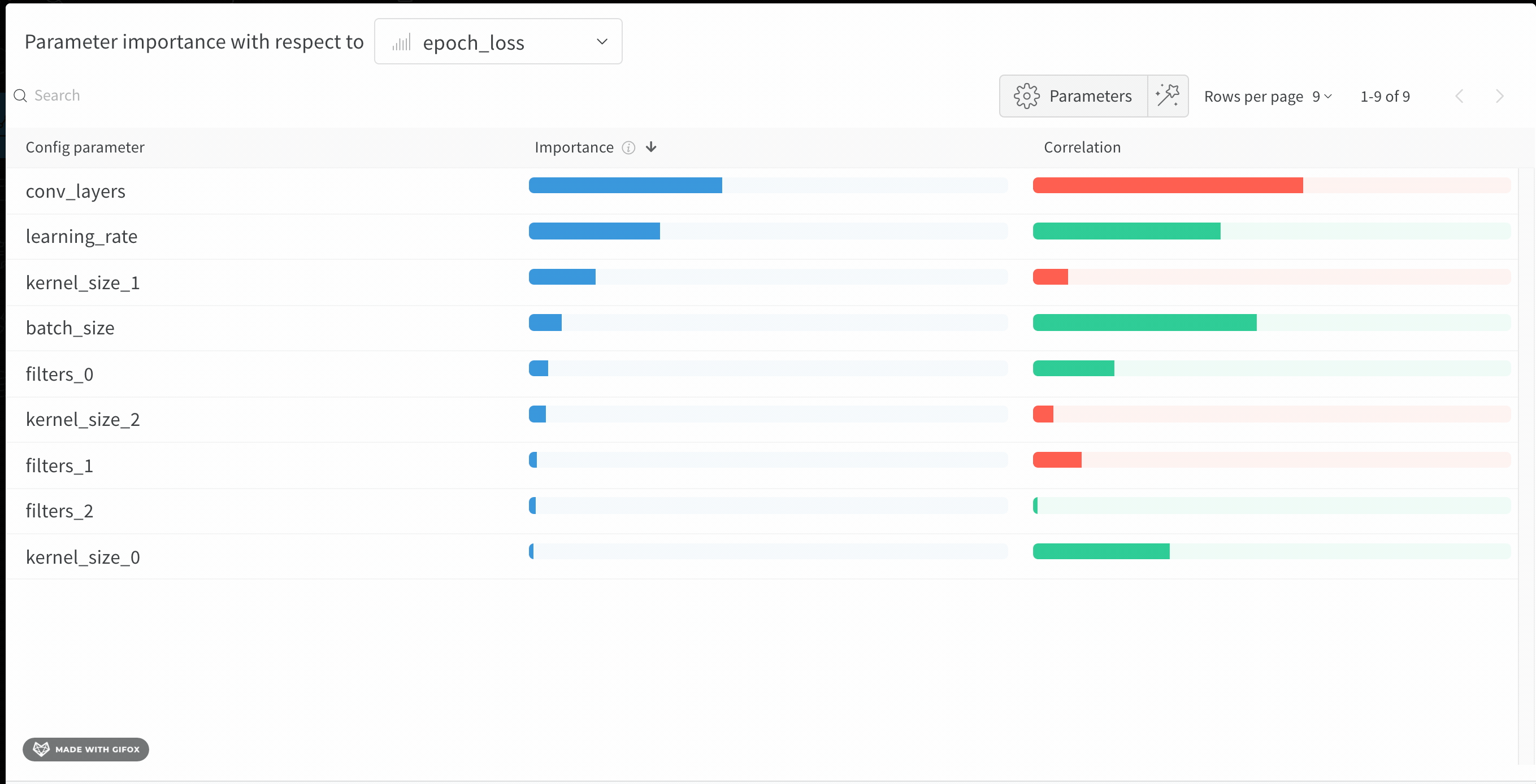
Interpreting a hyperparameter importance panel
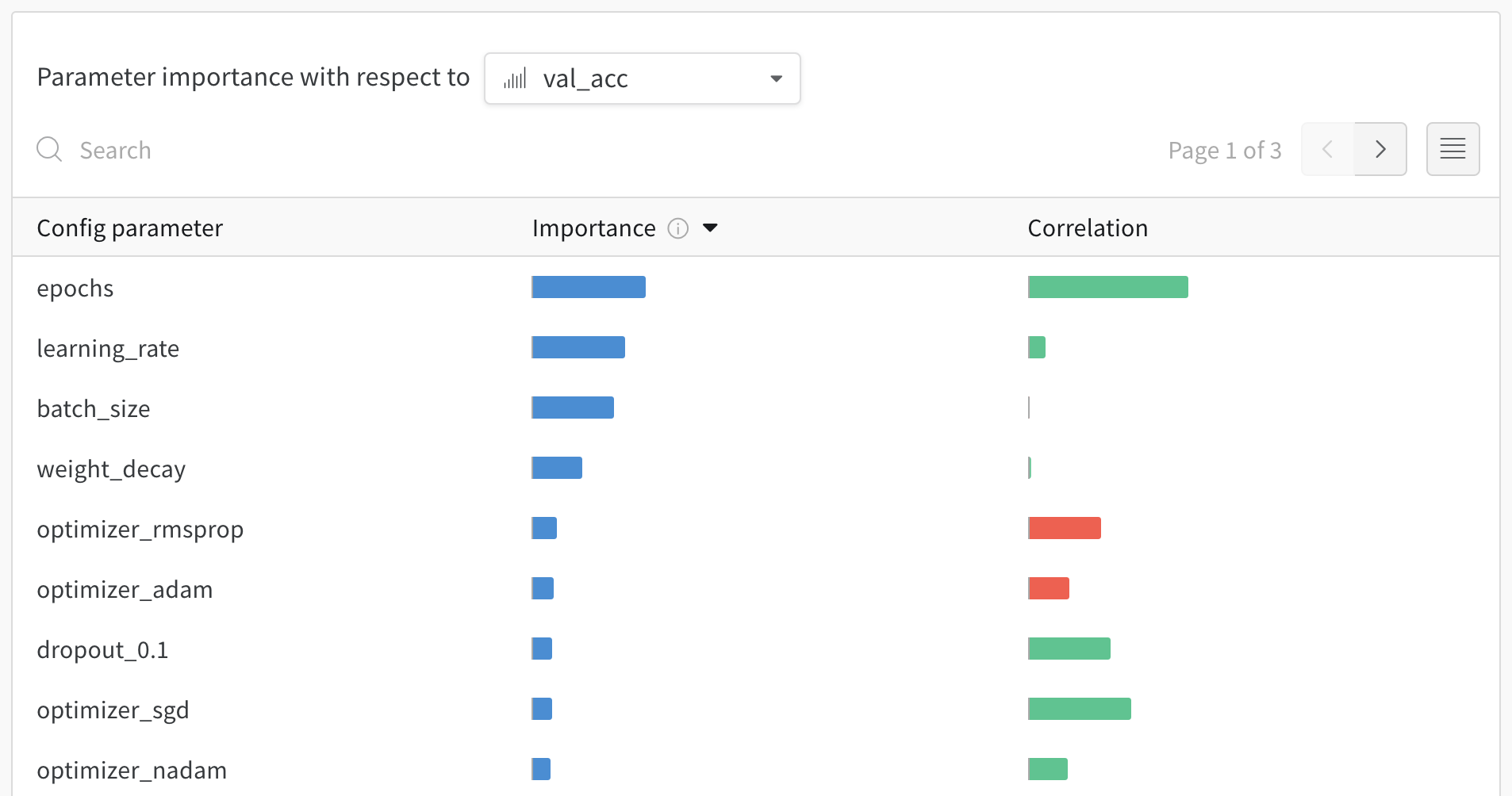
This panel shows you all the parameters passed to the wandb.config object in your training script. Next, it shows the feature importances and correlations of these config parameters with respect to the model metric you select (val_loss
in this case).
Importance
The importance column shows you the degree to which each hyperparameter was useful in predicting the chosen metric. Imagine a scenario were you start tuning a plethora of hyperparameters and using this plot to hone in on which ones merit further exploration. The subsequent sweeps can then be limited to the most important hyperparameters, thereby finding a better model faster and cheaper.
In the preceding image, you can see that epochs, learning_rate, batch_size
and weight_decay
were fairly important.
Correlations
Correlations capture linear relationships between individual hyperparameters and metric values. They answer the question of whether there a significant relationship between using a hyperparameter, such as the SGD optimizer, and the val_loss
(the answer in this case is yes). Correlation values range from -1 to 1, where positive values represent positive linear correlation, negative values represent negative linear correlation and a value of 0 represents no correlation. Generally a value greater than 0.7 in either direction represents strong correlation.
You might use this graph to further explore the values that are have a higher correlation to our metric (in this case you might pick stochastic gradient descent or adam over rmsprop or nadam) or train for more epochs.
- correlations show evidence of association, not necessarily causation.
- correlations are sensitive to outliers, which might turn a strong relationship to a moderate one, specially if the sample size of hyperparameters tried is small.
- and finally, correlations only capture linear relationships between hyperparameters and metrics. If there is a strong polynomial relationship, it won’t be captured by correlations.
The disparities between importance and correlations result from the fact that importance accounts for interactions between hyperparameters, whereas correlation only measures the affects of individual hyperparameters on metric values. Secondly, correlations capture only the linear relationships, whereas importances can capture more complex ones.
As you can see both importance and correlations are powerful tools for understanding how your hyperparameters influence model performance.
1.7 - Compare run metrics
Use the Run Comparer to see what metrics are different across your runs.
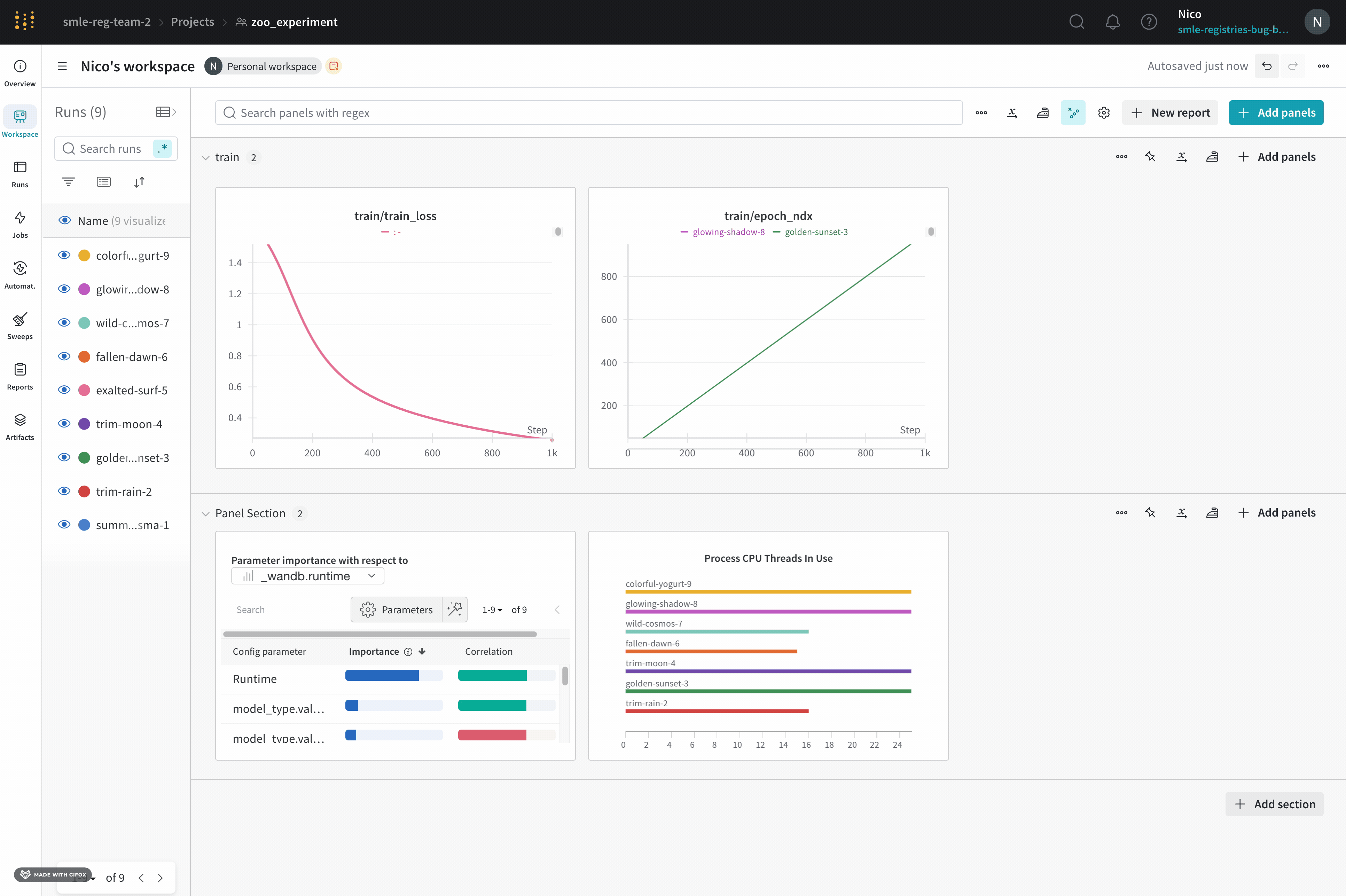
- Select the Add panels button in the top right corner of the page.
- From the left panel that appears, expand the Evaluation dropdown.
- Select Run comparer
Toggle the diff only option to hide rows where the values are the same across runs.
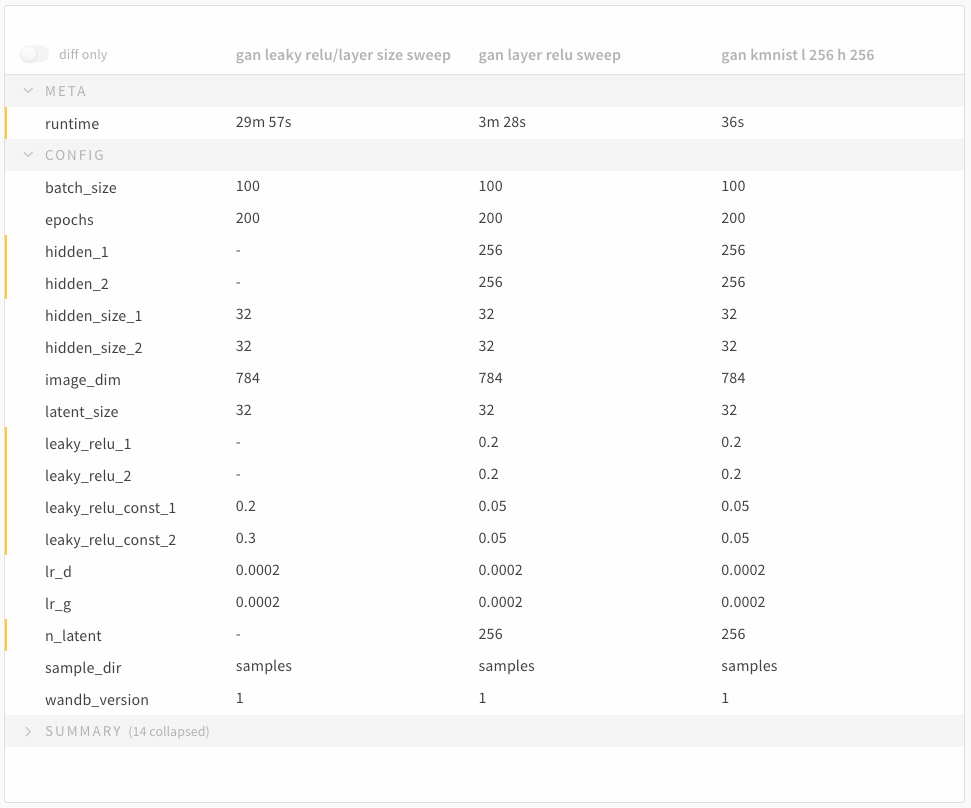
1.8 - Query panels
weave-plot
to your bio on your profile page to unlock all related features.Use query panels to query and interactively visualize your data.
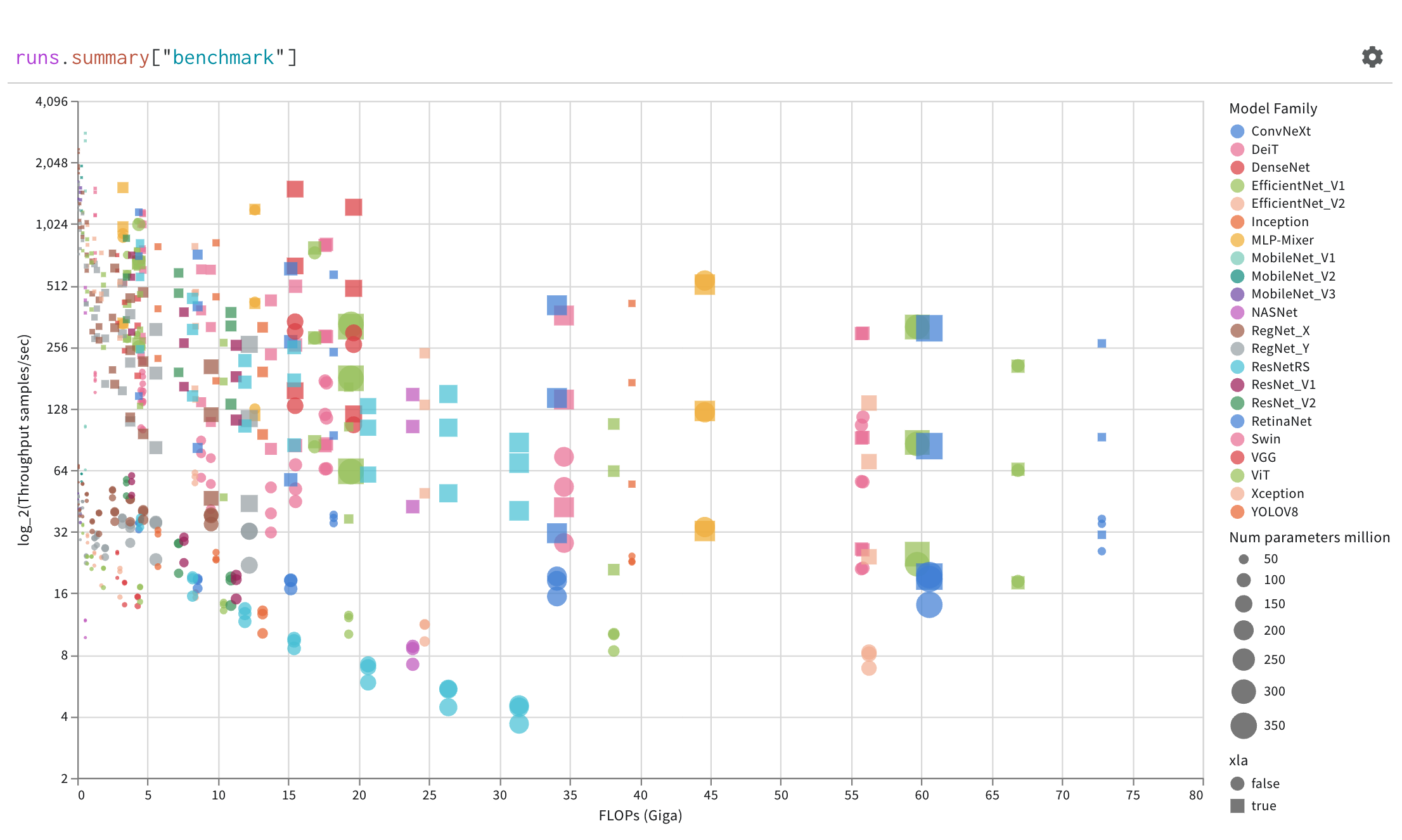
Create a query panel
Add a query to your workspace or within a report.
- Navigate to your project’s workspace.
- In the upper right hand corner, click
Add panel
. - From the dropdown, select
Query panel
.
Type and select /Query panel
.
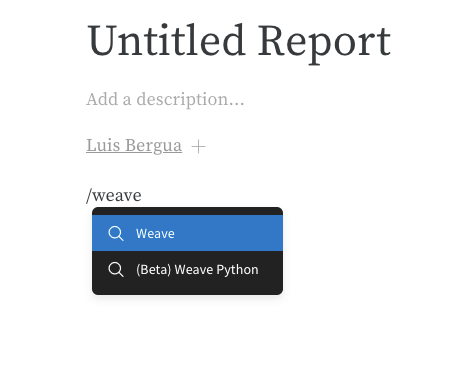
Alternatively, you can associate a query with a set of runs:
- Within your report, type and select
/Panel grid
. - Click the
Add panel
button. - From the dropdown, select
Query panel
.
Query components
Expressions
Use query expressions to query your data stored in W&B such as runs, artifacts, models, tables, and more.
Example: Query a table
Suppose you want to query a W&B Table. In your training code you log a table called "cifar10_sample_table"
:
import wandb
wandb.log({"cifar10_sample_table":<MY_TABLE>})
Within the query panel you can query your table with:
runs.summary["cifar10_sample_table"]

Breaking this down:
runs
is a variable automatically injected in Query Panel Expressions when the Query Panel is in a Workspace. Its “value” is the list of runs which are visible for that particular Workspace. Read about the different attributes available within a run here.summary
is an op which returns the Summary object for a Run. Ops are mapped, meaning this op is applied to each Run in the list, resulting in a list of Summary objects.["cifar10_sample_table"]
is a Pick op (denoted with brackets), with a parameter ofpredictions
. Since Summary objects act like dictionaries or maps, this operation picks thepredictions
field off of each Summary object.
To learn how to write your own queries interactively, see this report.
Configurations
Select the gear icon on the upper left corner of the panel to expand the query configuration. This allows the user to configure the type of panel and the parameters for the result panel.
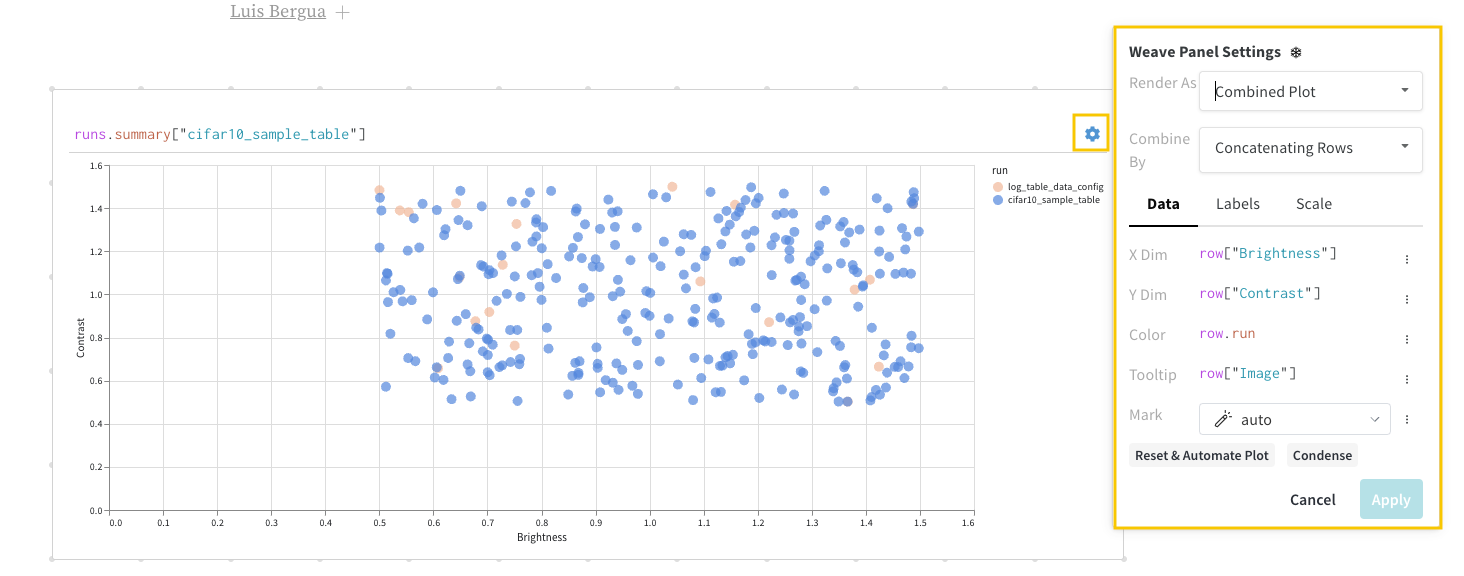
Result panels
Finally, the query result panel renders the result of the query expression, using the selected query panel, configured by the configuration to display the data in an interactive form. The following images shows a Table and a Plot of the same data.
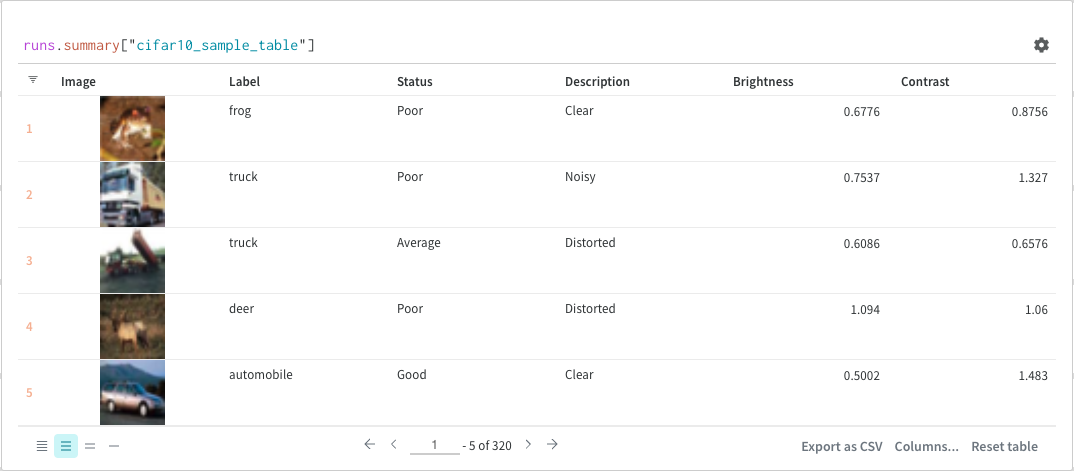
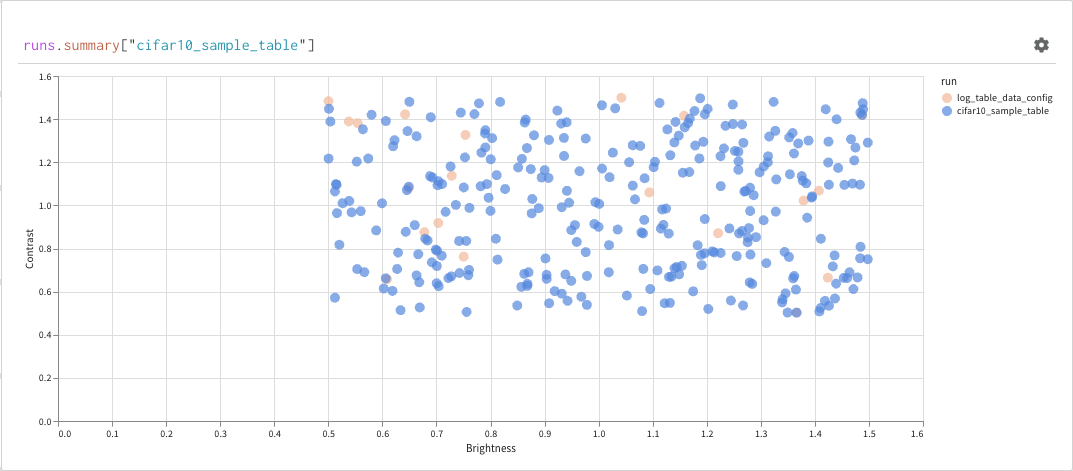
Basic operations
The following common operations you can make within your query panels.
Sort
Sort from the column options:
Filter
You can either filter directly in the query or using the filter button in the top left corner (second image)
Map
Map operations iterate over lists and apply a function to each element in the data. You can do this directly with a panel query or by inserting a new column from the column options.
Groupby
You can groupby using a query or from the column options.
Concat
The concat operation allows you to concatenate 2 tables and concatenate or join from the panel settings
Join
It is also possible to join tables directly in the query. Consider the following query expression:
project("luis_team_test", "weave_example_queries").runs.summary["short_table_0"].table.rows.concat.join(\
project("luis_team_test", "weave_example_queries").runs.summary["short_table_1"].table.rows.concat,\
(row) => row["Label"],(row) => row["Label"], "Table1", "Table2",\
"false", "false")
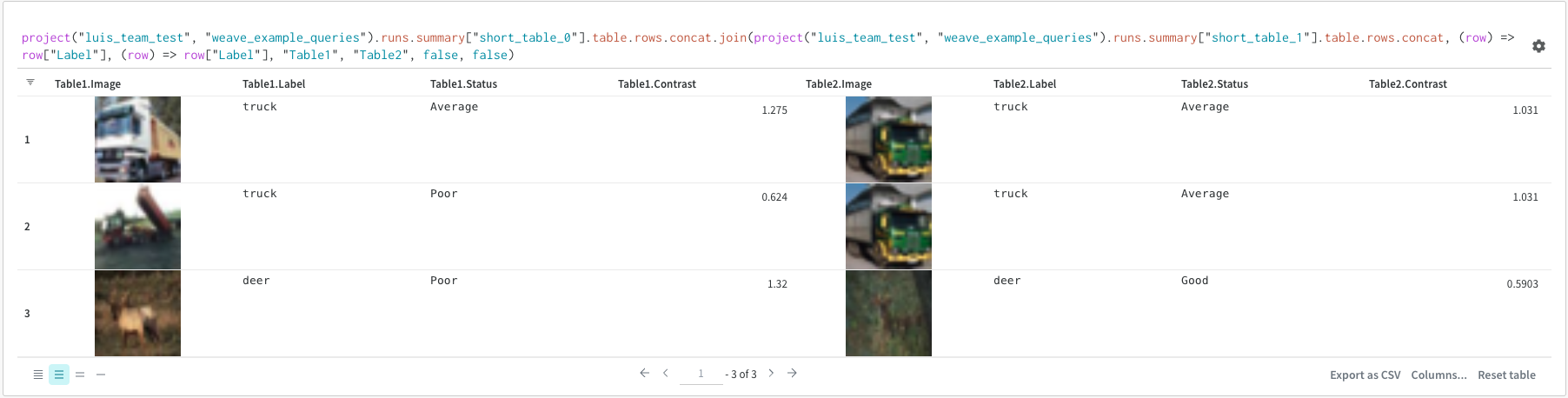
The table on the left is generated from:
project("luis_team_test", "weave_example_queries").\
runs.summary["short_table_0"].table.rows.concat.join
The table in the right is generated from:
project("luis_team_test", "weave_example_queries").\
runs.summary["short_table_1"].table.rows.concat
Where:
(row) => row["Label"]
are selectors for each table, determining which column to join on"Table1"
and"Table2"
are the names of each table when joinedtrue
andfalse
are for left and right inner/outer join settings
Runs object
Use query panels to access the runs
object. Run objects store records of your experiments. You can find more details about it in this section of the report but, as quick overview, runs
object has available:
summary
: A dictionary of information that summarizes the run’s results. This can be scalars like accuracy and loss, or large files. By default,wandb.log()
sets the summary to the final value of a logged time series. You can set the contents of the summary directly. Think of the summary as the run’s outputs.history
: A list of dictionaries meant to store values that change while the model is training such as loss. The commandwandb.log()
appends to this object.config
: A dictionary of the run’s configuration information, such as the hyperparameters for a training run or the preprocessing methods for a run that creates a dataset Artifact. Think of these as the run’s “inputs”
Access Artifacts
Artifacts are a core concept in W&B. They are a versioned, named collection of files and directories. Use Artifacts to track model weights, datasets, and any other file or directory. Artifacts are stored in W&B and can be downloaded or used in other runs. You can find more details and examples in this section of the report. Artifacts are normally accessed from the project
object:
project.artifactVersion()
: returns the specific artifact version for a given name and version within a projectproject.artifact("")
: returns the artifact for a given name within a project. You can then use.versions
to get a list of all versions of this artifactproject.artifactType()
: returns theartifactType
for a given name within a project. You can then use.artifacts
to get a list of all artifacts with this typeproject.artifactTypes
: returns a list of all artifact types under the project
1.8.1 - Embed objects
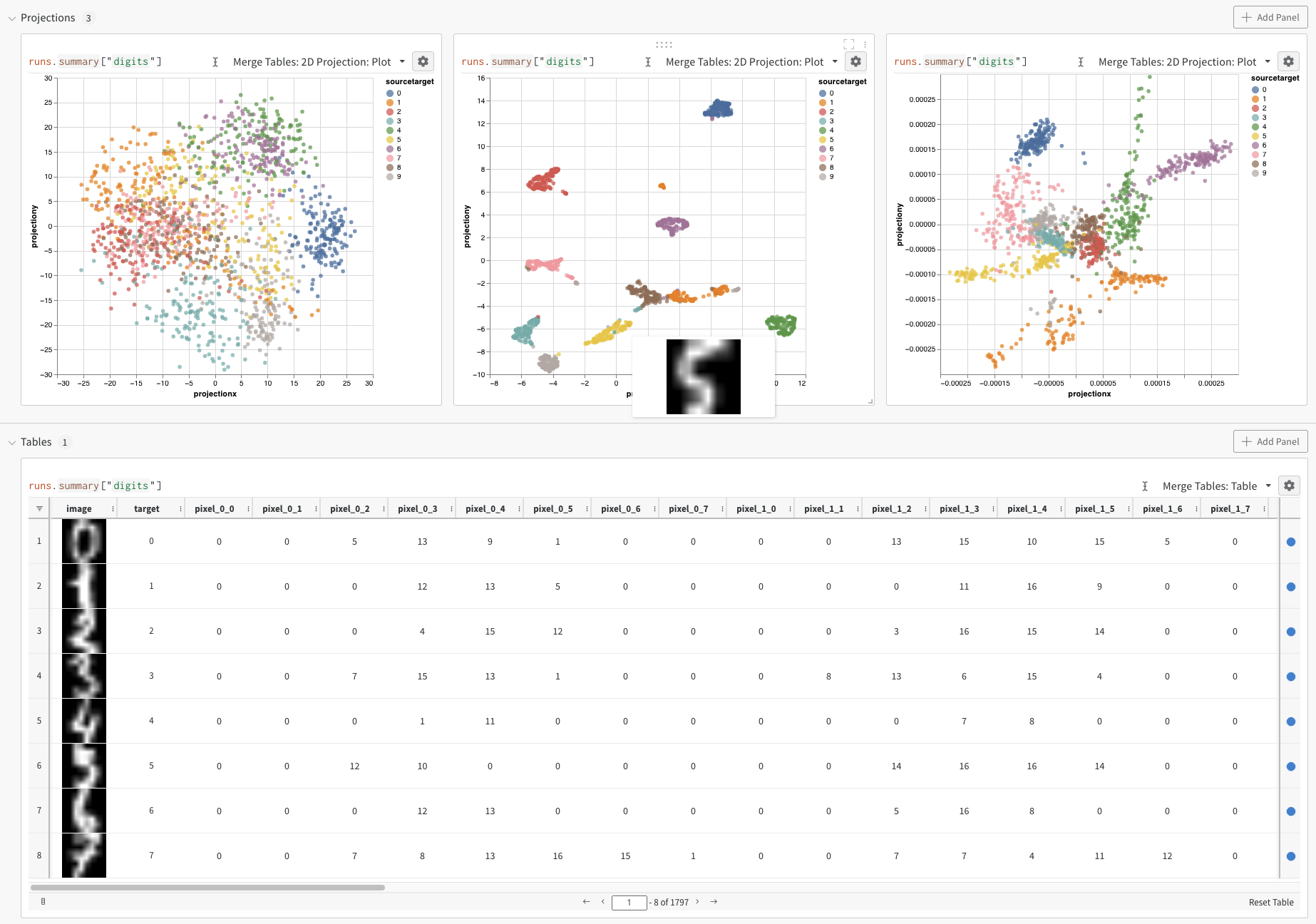
Embeddings are used to represent objects (people, images, posts, words, etc…) with a list of numbers - sometimes referred to as a vector. In machine learning and data science use cases, embeddings can be generated using a variety of approaches across a range of applications. This page assumes the reader is familiar with embeddings and is interested in visually analyzing them inside of W&B.
Embedding Examples
Hello World
W&B allows you to log embeddings using the wandb.Table
class. Consider the following example of 3 embeddings, each consisting of 5 dimensions:
import wandb
wandb.init(project="embedding_tutorial")
embeddings = [
# D1 D2 D3 D4 D5
[0.2, 0.4, 0.1, 0.7, 0.5], # embedding 1
[0.3, 0.1, 0.9, 0.2, 0.7], # embedding 2
[0.4, 0.5, 0.2, 0.2, 0.1], # embedding 3
]
wandb.log(
{"embeddings": wandb.Table(columns=["D1", "D2", "D3", "D4", "D5"], data=embeddings)}
)
wandb.finish()
After running the above code, the W&B dashboard will have a new Table containing your data. You can select 2D Projection
from the upper right panel selector to plot the embeddings in 2 dimensions. Smart default will be automatically selected, which can be easily overridden in the configuration menu accessed by clicking the gear icon. In this example, we automatically use all 5 available numeric dimensions.
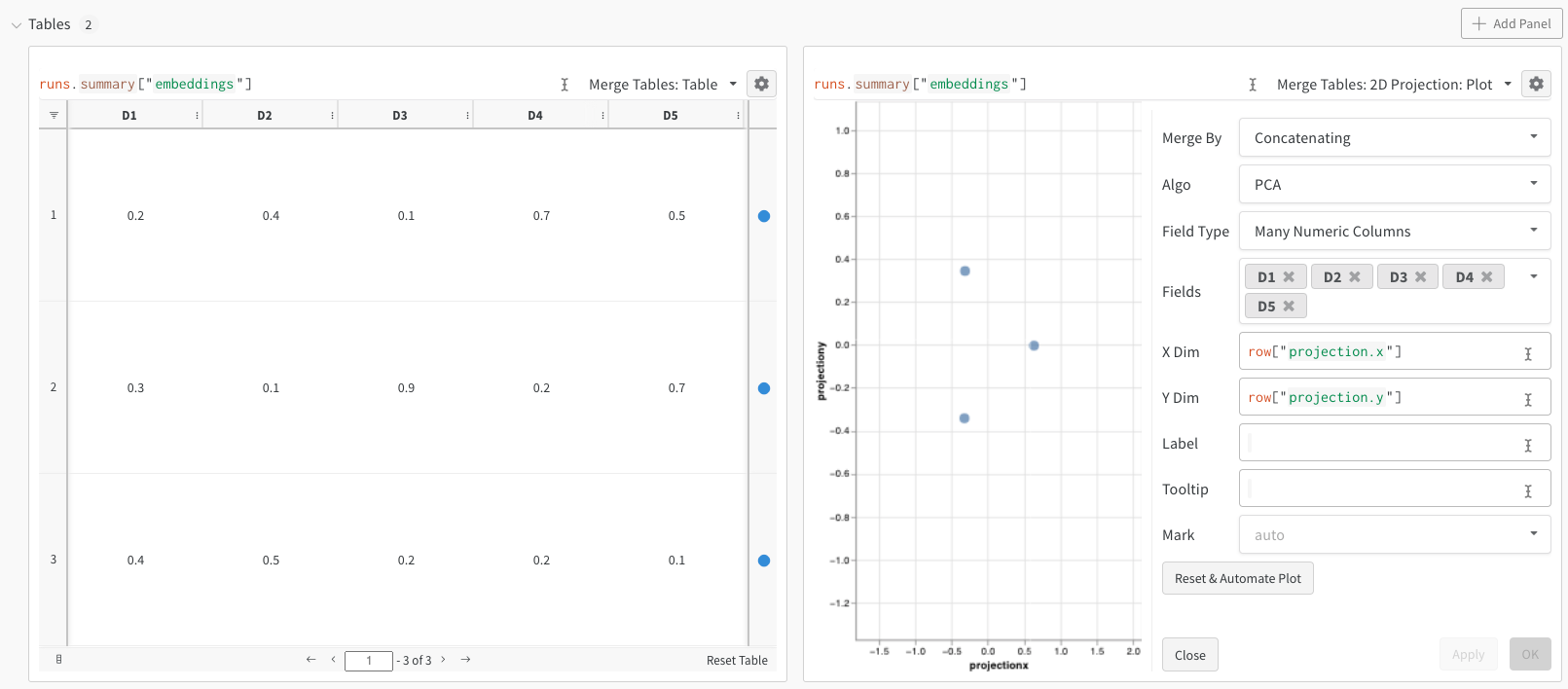
Digits MNIST
While the above example shows the basic mechanics of logging embeddings, typically you are working with many more dimensions and samples. Let’s consider the MNIST Digits dataset (UCI ML hand-written digits datasets) made available via SciKit-Learn. This dataset has 1797 records, each with 64 dimensions. The problem is a 10 class classification use case. We can convert the input data to an image for visualization as well.
import wandb
from sklearn.datasets import load_digits
wandb.init(project="embedding_tutorial")
# Load the dataset
ds = load_digits(as_frame=True)
df = ds.data
# Create a "target" column
df["target"] = ds.target.astype(str)
cols = df.columns.tolist()
df = df[cols[-1:] + cols[:-1]]
# Create an "image" column
df["image"] = df.apply(
lambda row: wandb.Image(row[1:].values.reshape(8, 8) / 16.0), axis=1
)
cols = df.columns.tolist()
df = df[cols[-1:] + cols[:-1]]
wandb.log({"digits": df})
wandb.finish()
After running the above code, again we are presented with a Table in the UI. By selecting 2D Projection
we can configure the definition of the embedding, coloring, algorithm (PCA, UMAP, t-SNE), algorithm parameters, and even overlay (in this case we show the image when hovering over a point). In this particular case, these are all “smart defaults” and you should see something very similar with a single click on 2D Projection
. (Click here to interact with this example).
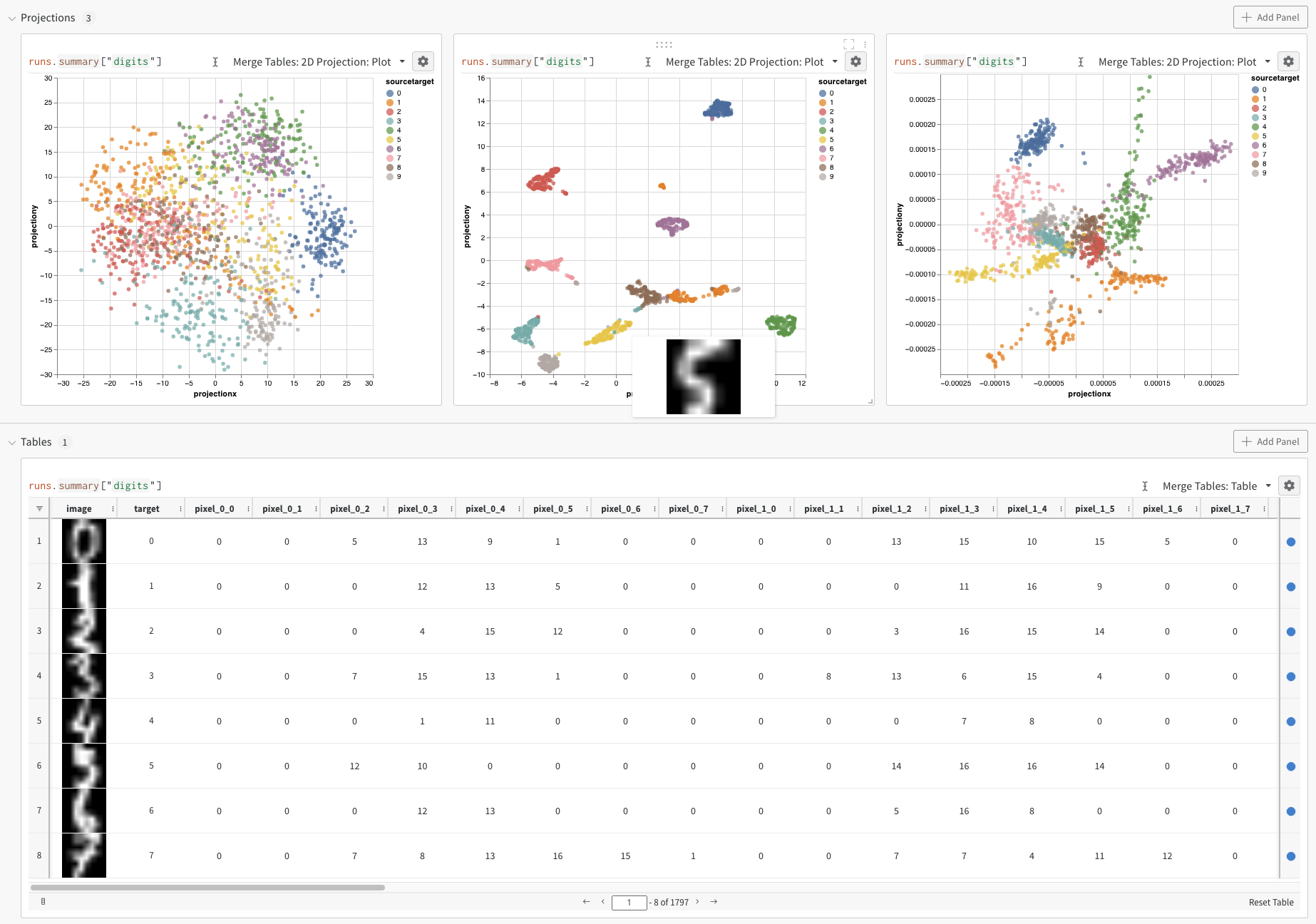
Logging Options
You can log embeddings in a number of different formats:
- Single Embedding Column: Often your data is already in a “matrix”-like format. In this case, you can create a single embedding column - where the data type of the cell values can be
list[int]
,list[float]
, ornp.ndarray
. - Multiple Numeric Columns: In the above two examples, we use this approach and create a column for each dimension. We currently accept python
int
orfloat
for the cells.
Furthermore, just like all tables, you have many options regarding how to construct the table:
- Directly from a dataframe using
wandb.Table(dataframe=df)
- Directly from a list of data using
wandb.Table(data=[...], columns=[...])
- Build the table incrementally row-by-row (great if you have a loop in your code). Add rows to your table using
table.add_data(...)
- Add an embedding column to your table (great if you have a list of predictions in the form of embeddings):
table.add_col("col_name", ...)
- Add a computed column (great if you have a function or model you want to map over your table):
table.add_computed_columns(lambda row, ndx: {"embedding": model.predict(row)})
Plotting Options
After selecting 2D Projection
, you can click the gear icon to edit the rendering settings. In addition to selecting the intended columns (see above), you can select an algorithm of interest (along with the desired parameters). Below you can see the parameters for UMAP and t-SNE respectively.
2 - Custom charts
Use Custom Charts to create charts that aren’t possible right now in the default UI. Log arbitrary tables of data and visualize them exactly how you want. Control details of fonts, colors, and tooltips with the power of Vega.
- What’s possible: Read the launch announcement
- Code: Try a live example in a hosted notebook
- Video: Watch a quick walkthrough video
- Example: Quick Keras and Sklearn demo notebook
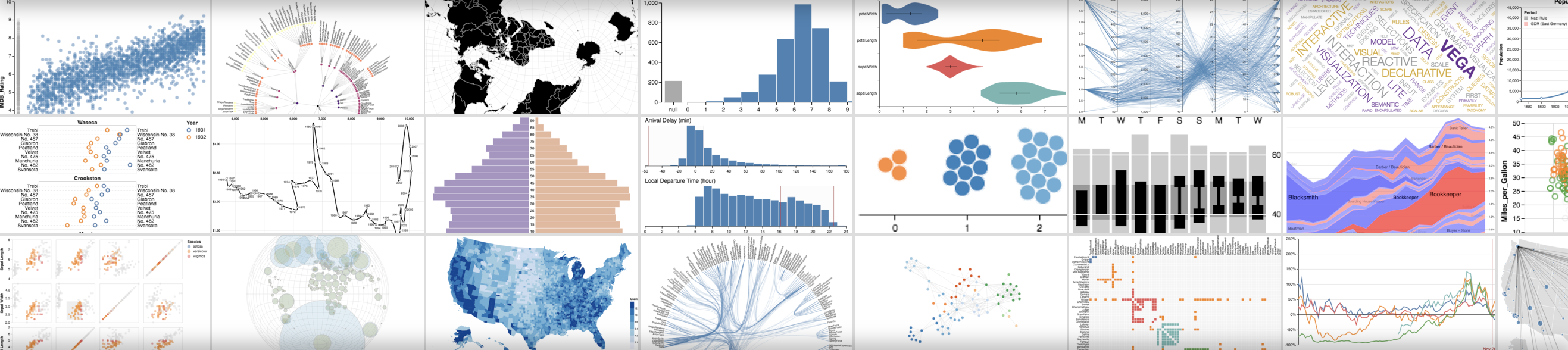
How it works
- Log data: From your script, log config and summary data as you normally would when running with W&B. To visualize a list of multiple values logged at one specific time, use a custom
wandb.Table
- Customize the chart: Pull in any of this logged data with a GraphQL query. Visualize the results of your query with Vega, a powerful visualization grammar.
- Log the chart: Call your own preset from your script with
wandb.plot_table()
.
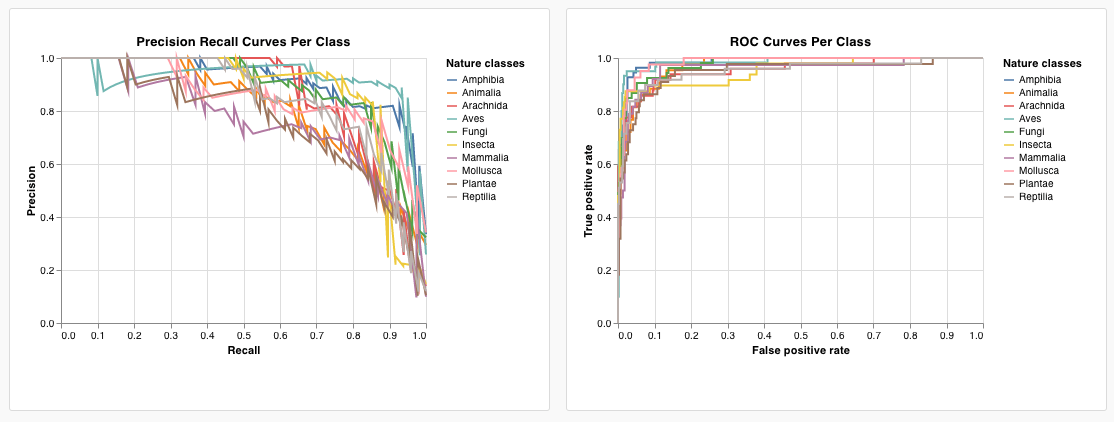
Log charts from a script
Builtin presets
These presets have builtin wandb.plot
methods that make it fast to log charts directly from your script and see the exact visualizations you’re looking for in the UI.
wandb.plot.line()
Log a custom line plot—a list of connected and ordered points (x,y) on arbitrary axes x and y.
data = [[x, y] for (x, y) in zip(x_values, y_values)]
table = wandb.Table(data=data, columns=["x", "y"])
wandb.log(
{
"my_custom_plot_id": wandb.plot.line(
table, "x", "y", title="Custom Y vs X Line Plot"
)
}
)
You can use this to log curves on any two dimensions. Note that if you’re plotting two lists of values against each other, the number of values in the lists must match exactly (for example, each point must have an x and a y).
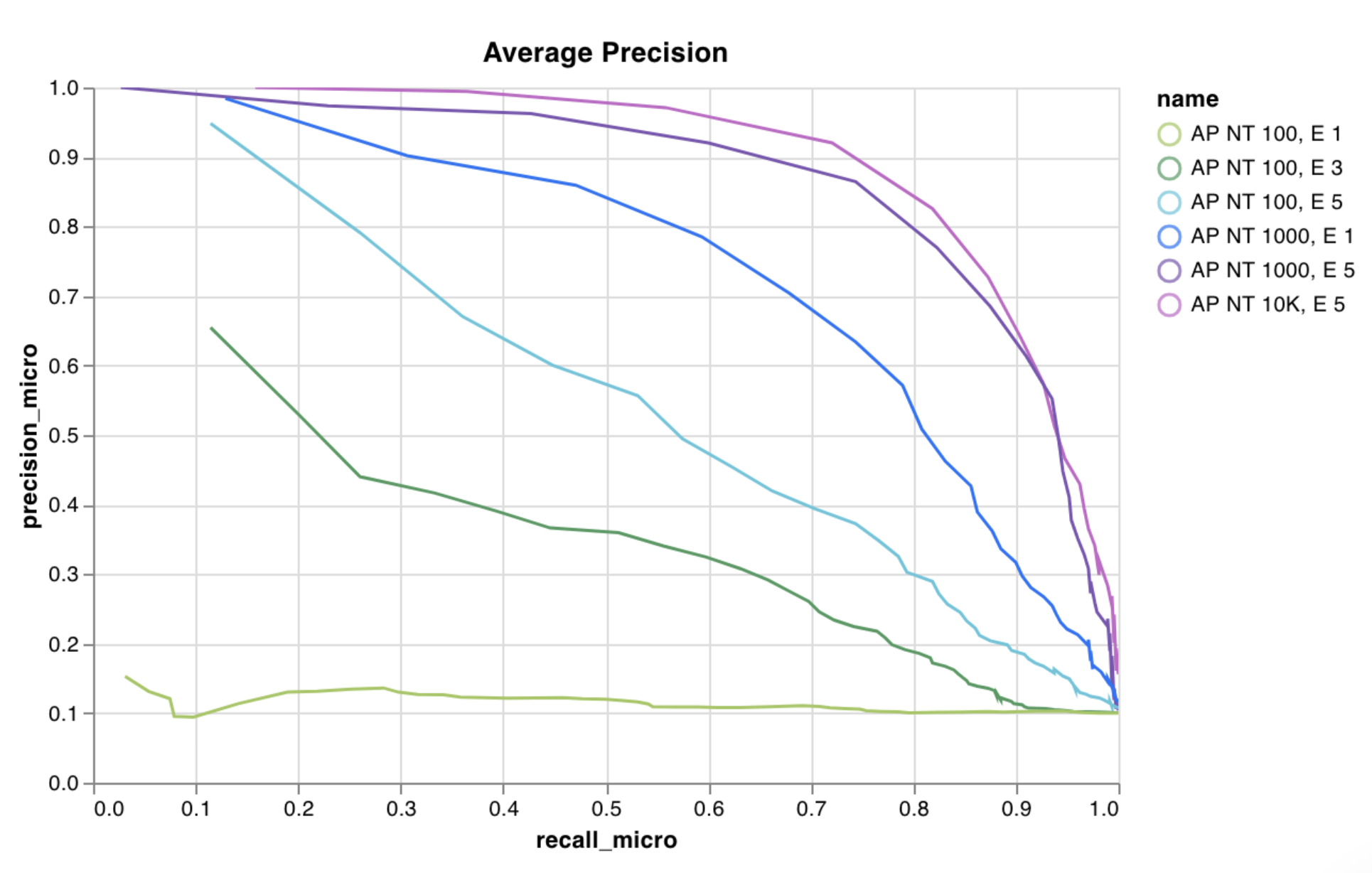
wandb.plot.scatter()
Log a custom scatter plot—a list of points (x, y) on a pair of arbitrary axes x and y.
data = [[x, y] for (x, y) in zip(class_x_prediction_scores, class_y_prediction_scores)]
table = wandb.Table(data=data, columns=["class_x", "class_y"])
wandb.log({"my_custom_id": wandb.plot.scatter(table, "class_x", "class_y")})
You can use this to log scatter points on any two dimensions. Note that if you’re plotting two lists of values against each other, the number of values in the lists must match exactly (for example, each point must have an x and a y).
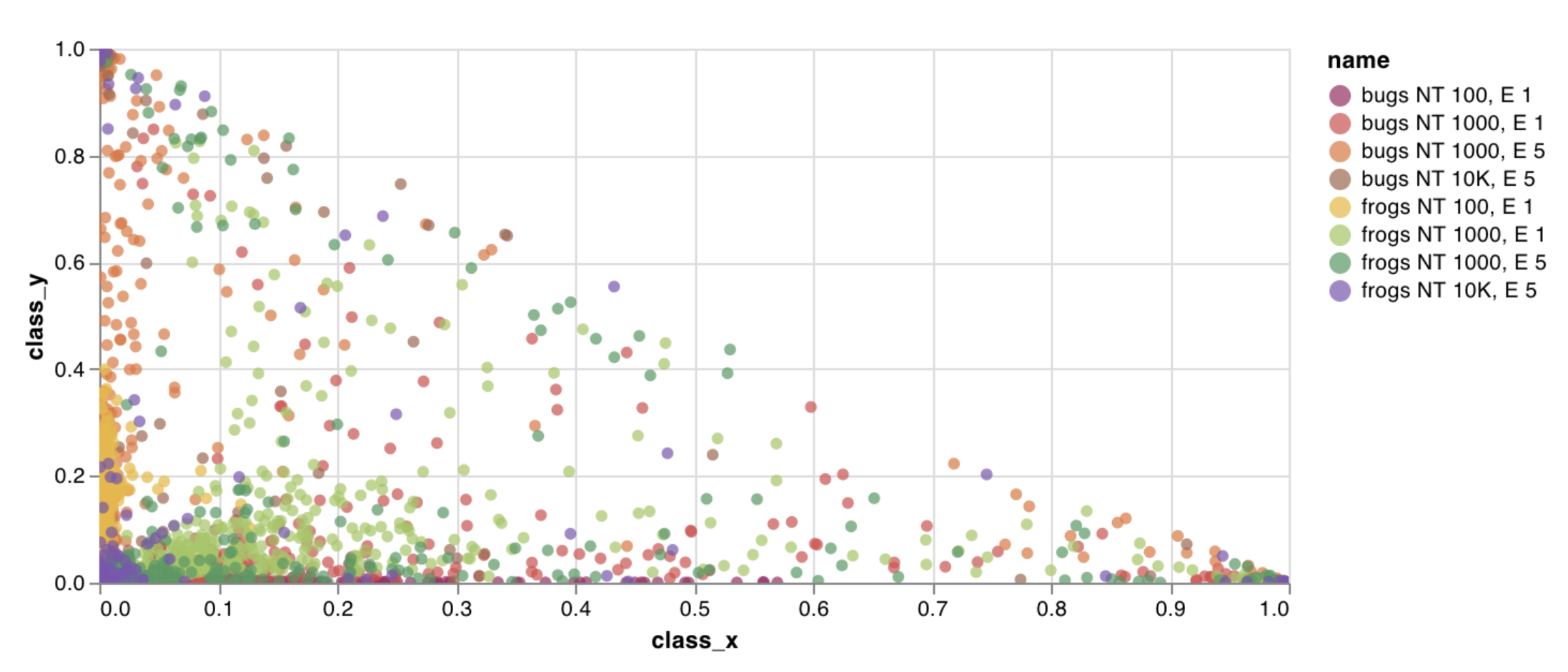
wandb.plot.bar()
Log a custom bar chart—a list of labeled values as bars—natively in a few lines:
data = [[label, val] for (label, val) in zip(labels, values)]
table = wandb.Table(data=data, columns=["label", "value"])
wandb.log(
{
"my_bar_chart_id": wandb.plot.bar(
table, "label", "value", title="Custom Bar Chart"
)
}
)
You can use this to log arbitrary bar charts. Note that the number of labels and values in the lists must match exactly (for example, each data point must have both).
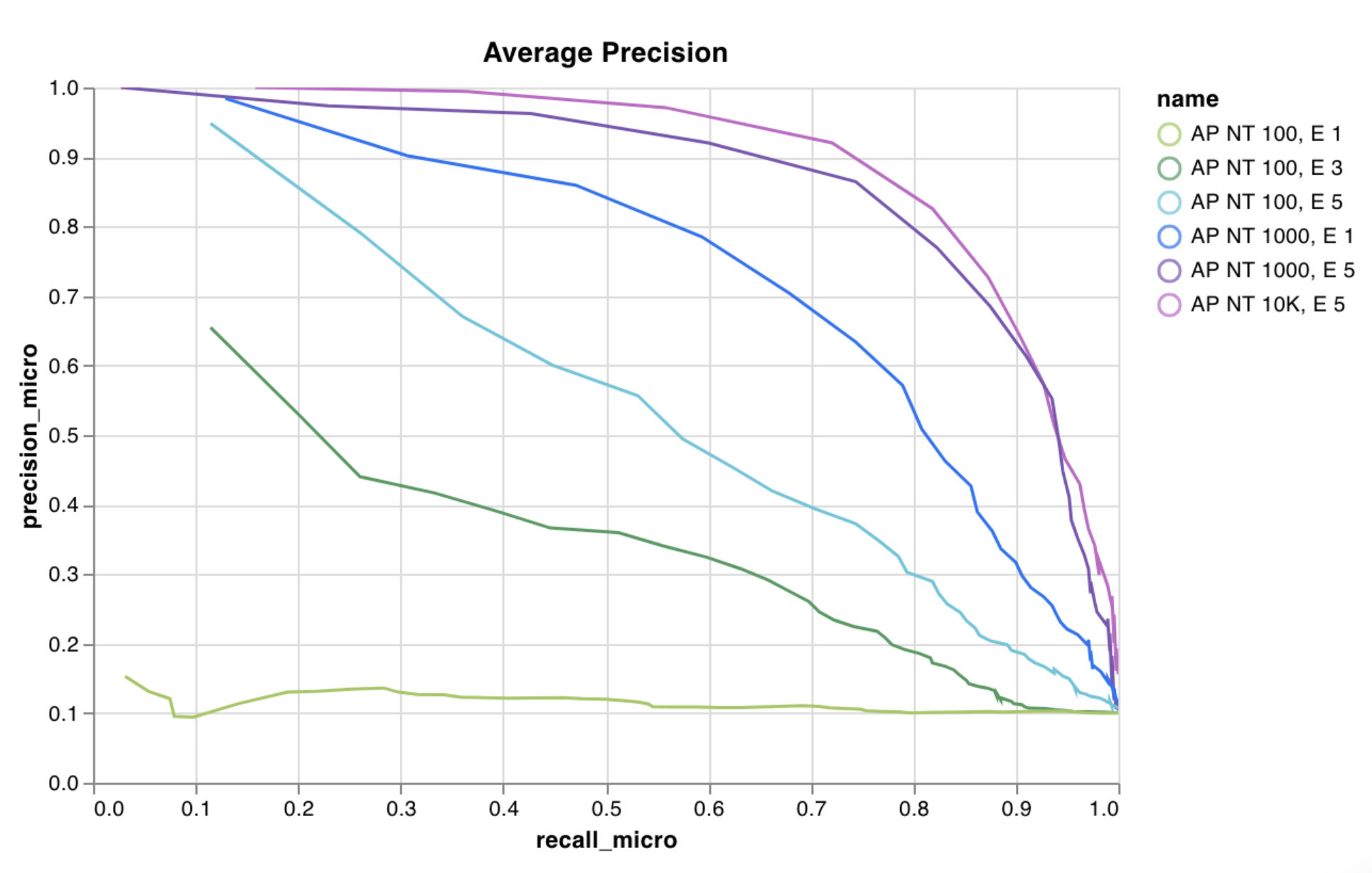
wandb.plot.histogram()
Log a custom histogram—sort list of values into bins by count/frequency of occurrence—natively in a few lines. Let’s say I have a list of prediction confidence scores (scores
) and want to visualize their distribution:
data = [[s] for s in scores]
table = wandb.Table(data=data, columns=["scores"])
wandb.log({"my_histogram": wandb.plot.histogram(table, "scores", title=None)})
You can use this to log arbitrary histograms. Note that data
is a list of lists, intended to support a 2D array of rows and columns.
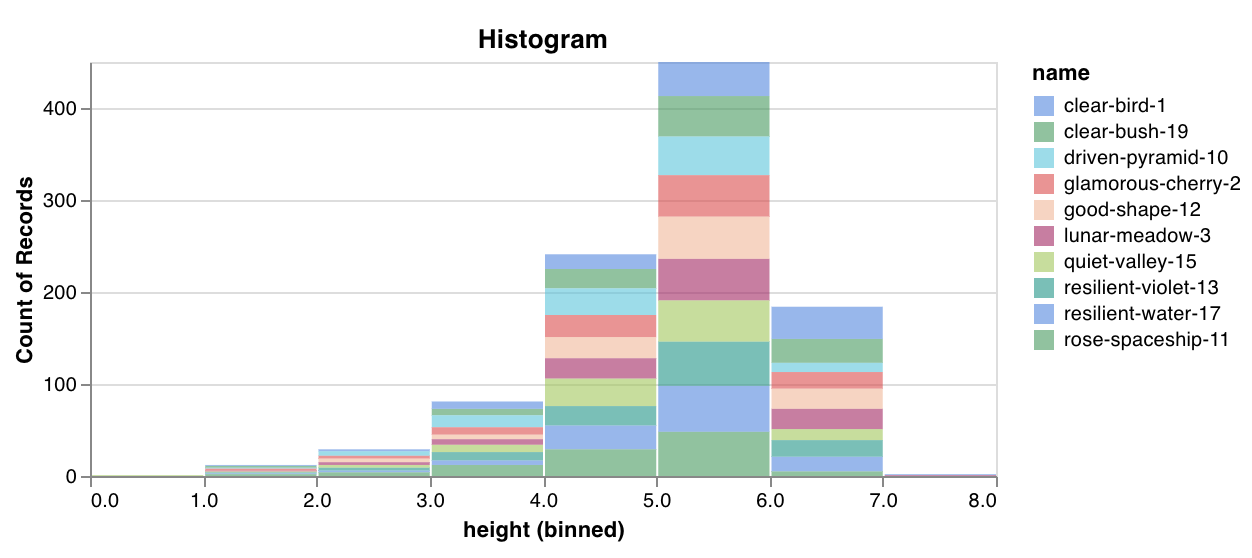
wandb.plot.pr_curve()
Create a Precision-Recall curve in one line:
plot = wandb.plot.pr_curve(ground_truth, predictions, labels=None, classes_to_plot=None)
wandb.log({"pr": plot})
You can log this whenever your code has access to:
- a model’s predicted scores (
predictions
) on a set of examples - the corresponding ground truth labels (
ground_truth
) for those examples - (optionally) a list of the labels/class names (
labels=["cat", "dog", "bird"...]
if label index 0 means cat, 1 = dog, 2 = bird, etc.) - (optionally) a subset (still in list format) of the labels to visualize in the plot
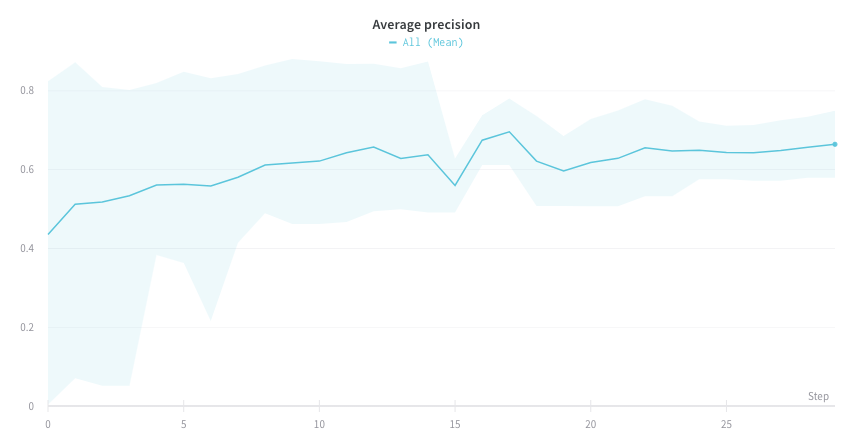
wandb.plot.roc_curve()
Create an ROC curve in one line:
plot = wandb.plot.roc_curve(
ground_truth, predictions, labels=None, classes_to_plot=None
)
wandb.log({"roc": plot})
You can log this whenever your code has access to:
- a model’s predicted scores (
predictions
) on a set of examples - the corresponding ground truth labels (
ground_truth
) for those examples - (optionally) a list of the labels/ class names (
labels=["cat", "dog", "bird"...]
if label index 0 means cat, 1 = dog, 2 = bird, etc.) - (optionally) a subset (still in list format) of these labels to visualize on the plot
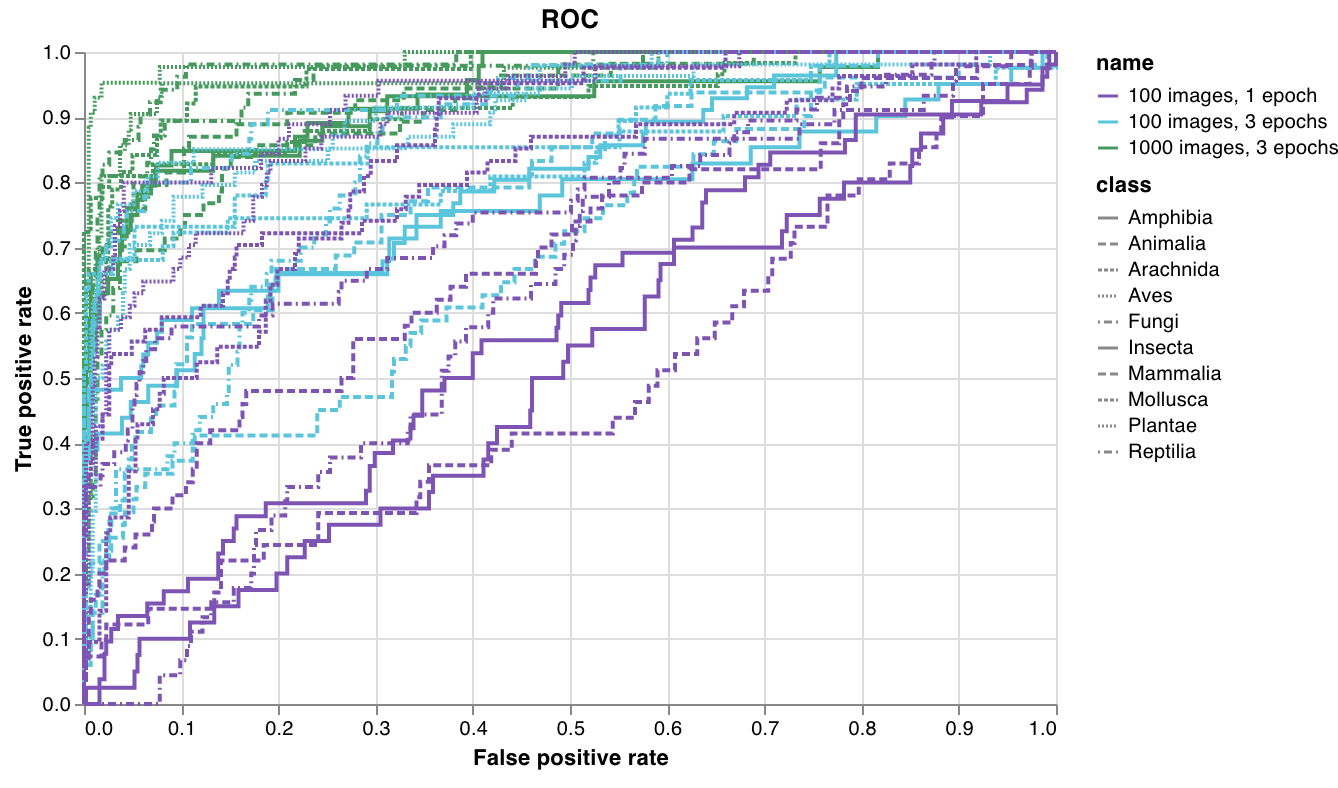
Custom presets
Tweak a builtin preset, or create a new preset, then save the chart. Use the chart ID to log data to that custom preset directly from your script.
# Create a table with the columns to plot
table = wandb.Table(data=data, columns=["step", "height"])
# Map from the table's columns to the chart's fields
fields = {"x": "step", "value": "height"}
# Use the table to populate the new custom chart preset
# To use your own saved chart preset, change the vega_spec_name
my_custom_chart = wandb.plot_table(
vega_spec_name="carey/new_chart",
data_table=table,
fields=fields,
)
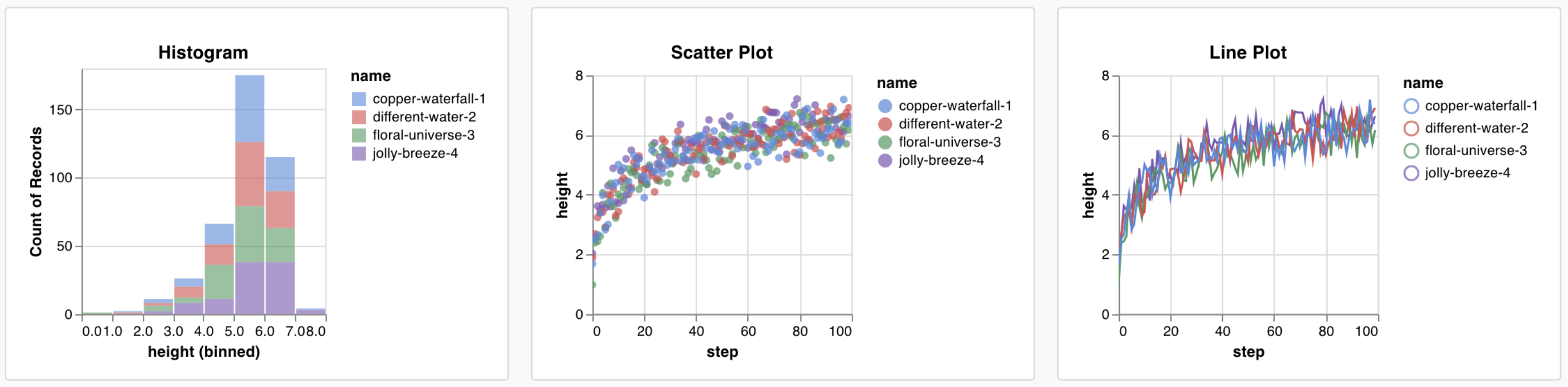
Log data
Here are the data types you can log from your script and use in a custom chart:
- Config: Initial settings of your experiment (your independent variables). This includes any named fields you’ve logged as keys to
wandb.config
at the start of your training. For example:wandb.config.learning_rate = 0.0001
- Summary: Single values logged during training (your results or dependent variables). For example,
wandb.log({"val_acc" : 0.8})
. If you write to this key multiple times during training viawandb.log()
, the summary is set to the final value of that key. - History: The full time series of the logged scalar is available to the query via the
history
field - summaryTable: If you need to log a list of multiple values, use a
wandb.Table()
to save that data, then query it in your custom panel. - historyTable: If you need to see the history data, then query
historyTable
in your custom chart panel. Each time you callwandb.Table()
or log a custom chart, you’re creating a new table in history for that step.
How to log a custom table
Use wandb.Table()
to log your data as a 2D array. Typically each row of this table represents one data point, and each column denotes the relevant fields/dimensions for each data point which you’d like to plot. As you configure a custom panel, the whole table will be accessible via the named key passed to wandb.log()
(custom_data_table
below), and the individual fields will be accessible via the column names (x
, y
, and z
). You can log tables at multiple time steps throughout your experiment. The maximum size of each table is 10,000 rows.
# Logging a custom table of data
my_custom_data = [[x1, y1, z1], [x2, y2, z2]]
wandb.log(
{"custom_data_table": wandb.Table(data=my_custom_data, columns=["x", "y", "z"])}
)
Customize the chart
Add a new custom chart to get started, then edit the query to select data from your visible runs. The query uses GraphQL to fetch data from the config, summary, and history fields in your runs.
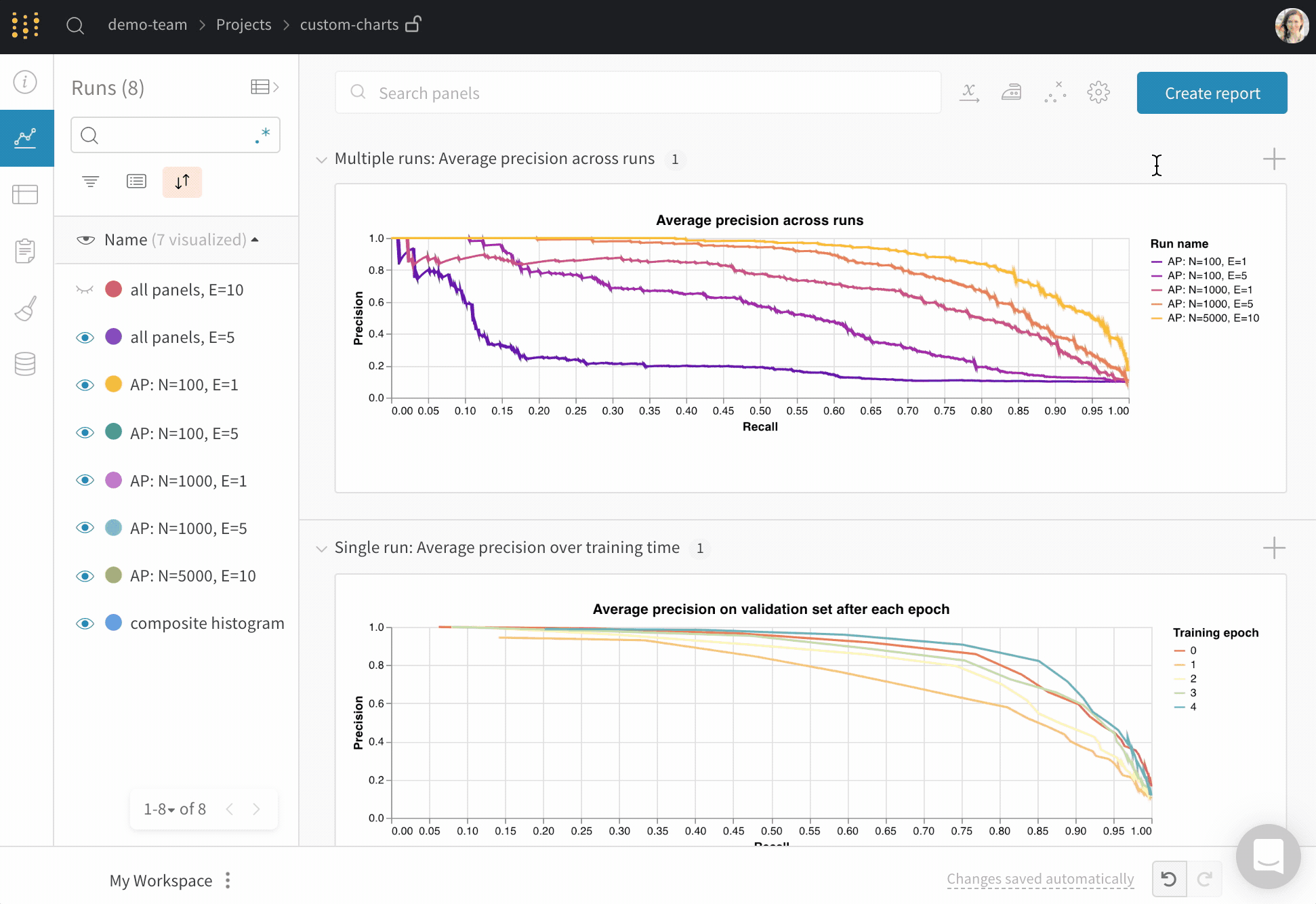
Custom visualizations
Select a Chart in the upper right corner to start with a default preset. Next, pick Chart fields to map the data you’re pulling in from the query to the corresponding fields in your chart. Here’s an example of selecting a metric to get from the query, then mapping that into the bar chart fields below.
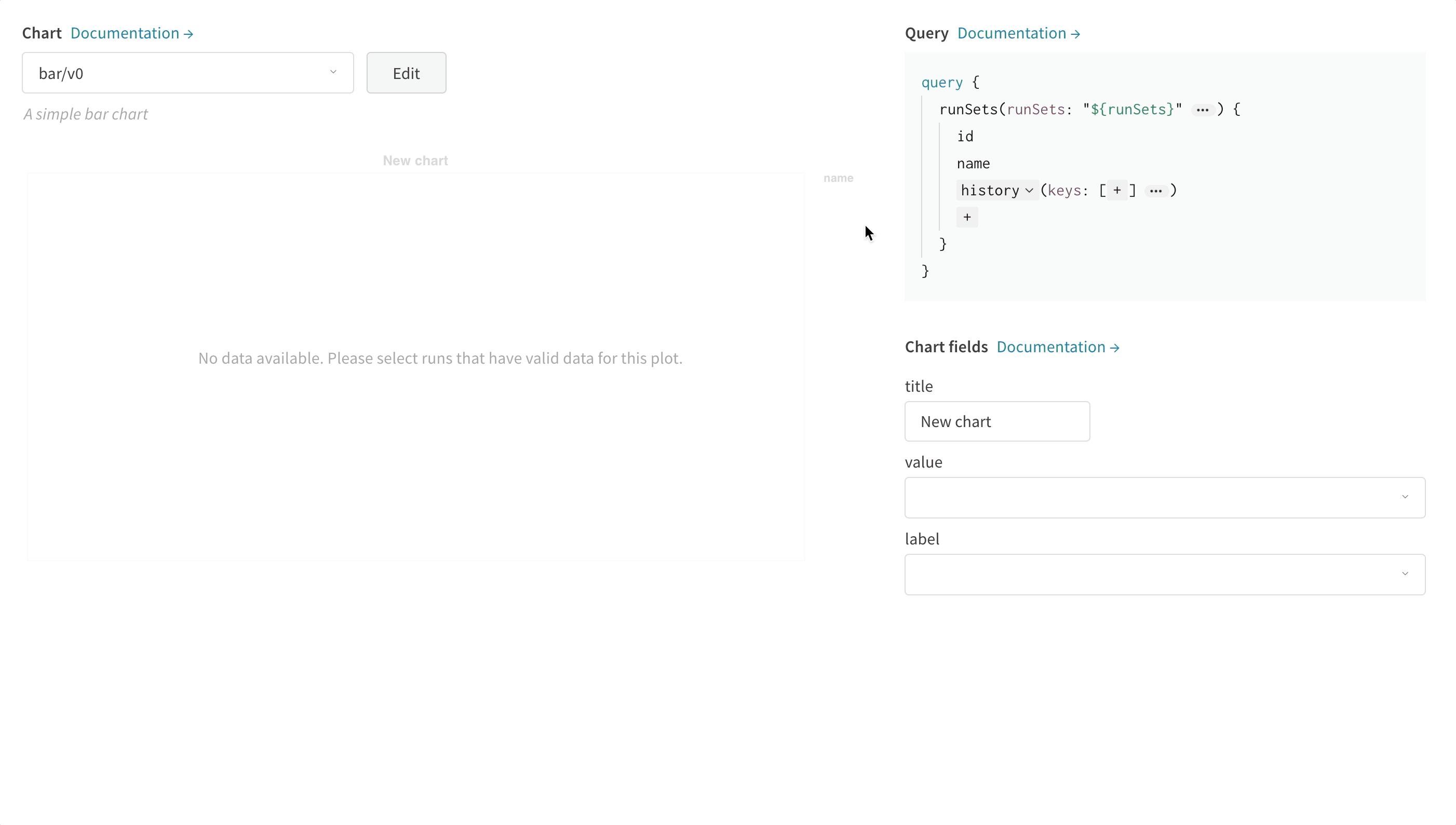
How to edit Vega
Click Edit at the top of the panel to go into Vega edit mode. Here you can define a Vega specification that creates an interactive chart in the UI. You can change any aspect of the chart. For example, you can change the title, pick a different color scheme, show curves as a series of points instead of as connected lines. You can also make changes to the data itself, such as using a Vega transform to bin an array of values into a histogram. The panel preview will update interactively, so you can see the effect of your changes as you edit the Vega spec or query. Refer to the Vega documentation and tutorials .
Field references
To pull data into your chart from W&B, add template strings of the form "${field:<field-name>}"
anywhere in your Vega spec. This will create a dropdown in the Chart Fields area on the right side, which users can use to select a query result column to map into Vega.
To set a default value for a field, use this syntax: "${field:<field-name>:<placeholder text>}"
Saving chart presets
Apply any changes to a specific visualization panel with the button at the bottom of the modal. Alternatively, you can save the Vega spec to use elsewhere in your project. To save the reusable chart definition, click Save as at the top of the Vega editor and give your preset a name.
Articles and guides
- The W&B Machine Learning Visualization IDE
- Visualizing NLP Attention Based Models
- Visualizing The Effect of Attention on Gradient Flow
- Logging arbitrary curves
Frequently asked questions
Coming soon
- Polling: Auto-refresh of data in the chart
- Sampling: Dynamically adjust the total number of points loaded into the panel for efficiency
Gotchas
- Not seeing the data you’re expecting in the query as you’re editing your chart? It might be because the column you’re looking for is not logged in the runs you have selected. Save your chart and go back out to the runs table, and select the runs you’d like to visualize with the eye icon.
Common use cases
- Customize bar plots with error bars
- Show model validation metrics which require custom x-y coordinates (like precision-recall curves)
- Overlay data distributions from two different models/experiments as histograms
- Show changes in a metric via snapshots at multiple points during training
- Create a unique visualization not yet available in W&B (and hopefully share it with the world)
2.1 - Tutorial: Use custom charts
Use custom charts to control the data you’re loading in to a panel and its visualization.
1. Log data to W&B
First, log data in your script. Use wandb.config for single points set at the beginning of training, like hyperparameters. Use wandb.log() for multiple points over time, and log custom 2D arrays with wandb.Table()
. We recommend logging up to 10,000 data points per logged key.
# Logging a custom table of data
my_custom_data = [[x1, y1, z1], [x2, y2, z2]]
wandb.log(
{"custom_data_table": wandb.Table(data=my_custom_data, columns=["x", "y", "z"])}
)
Try a quick example notebook to log the data tables, and in the next step we’ll set up custom charts. See what the resulting charts look like in the live report.
2. Create a query
Once you’ve logged data to visualize, go to your project page and click the +
button to add a new panel, then select Custom Chart. You can follow along in this workspace.
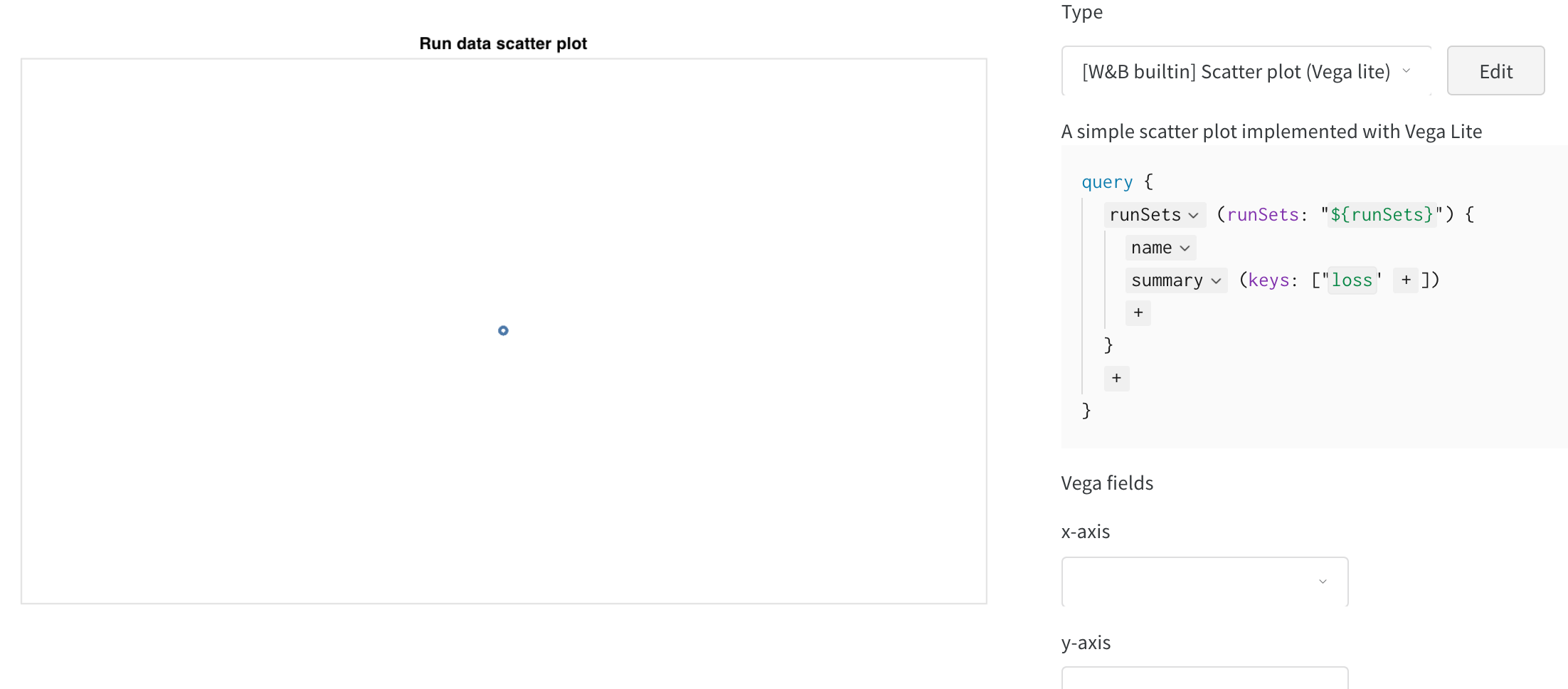
Add a query
- Click
summary
and selecthistoryTable
to set up a new query pulling data from the run history. - Type in the key where you logged the
wandb.Table()
. In the code snippet above, it wasmy_custom_table
. In the example notebook, the keys arepr_curve
androc_curve
.
Set Vega fields
Now that the query is loading in these columns, they’re available as options to select in the Vega fields dropdown menus:
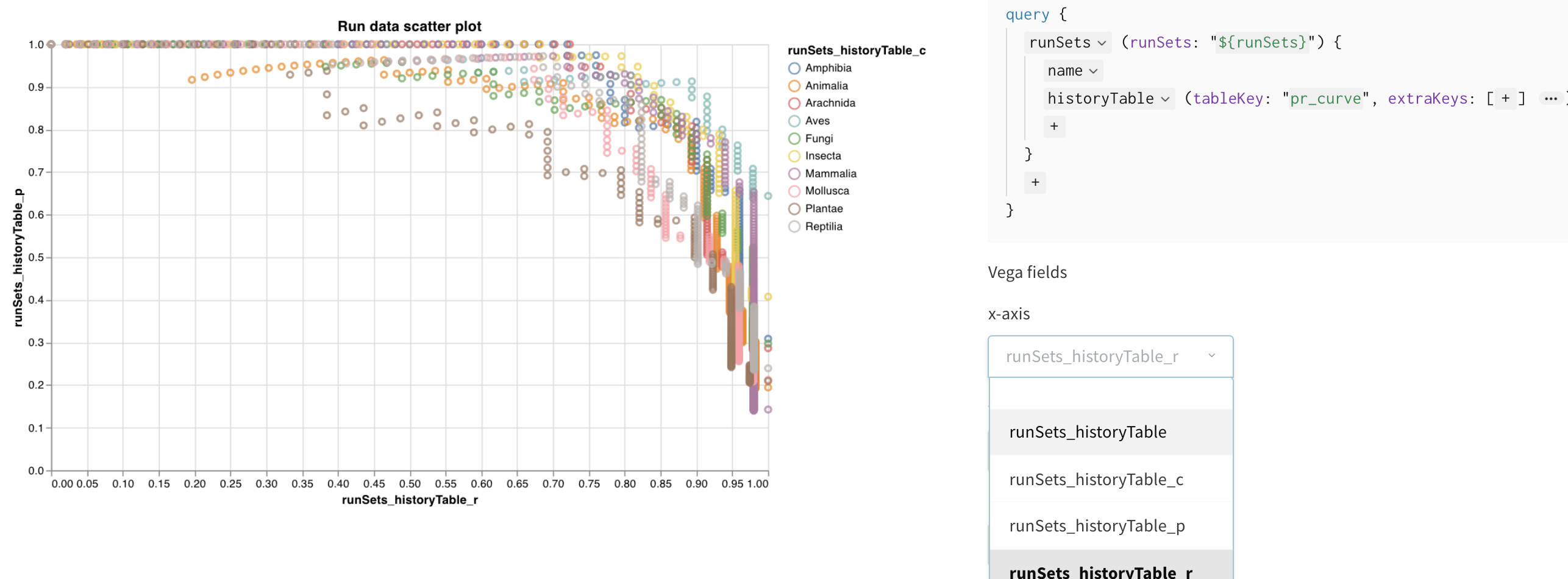
- x-axis: runSets_historyTable_r (recall)
- y-axis: runSets_historyTable_p (precision)
- color: runSets_historyTable_c (class label)
3. Customize the chart
Now that looks pretty good, but I’d like to switch from a scatter plot to a line plot. Click Edit to change the Vega spec for this built in chart. Follow along in this workspace.

I updated the Vega spec to customize the visualization:
- add titles for the plot, legend, x-axis, and y-axis (set “title” for each field)
- change the value of “mark” from “point” to “line”
- remove the unused “size” field
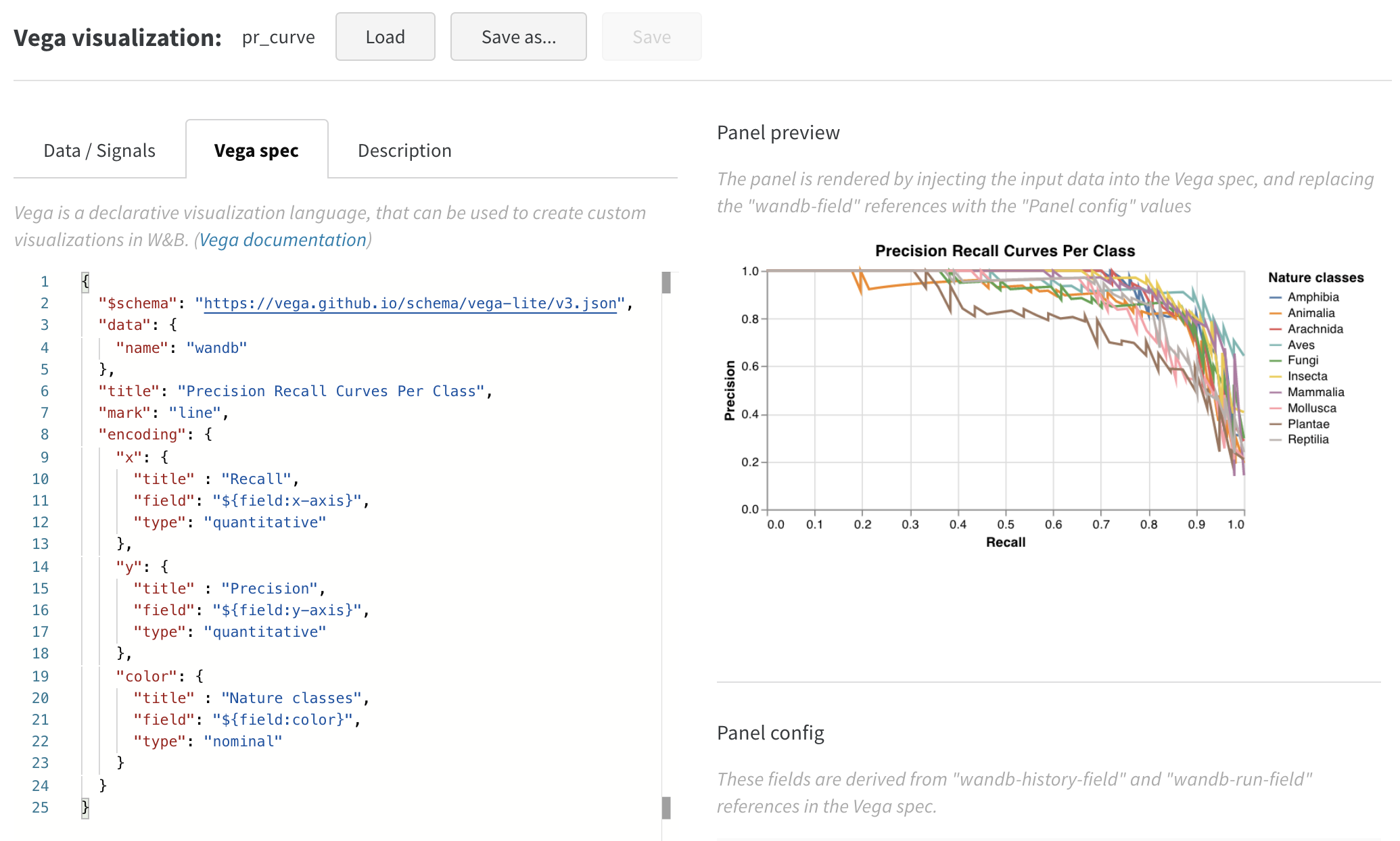
To save this as a preset that you can use elsewhere in this project, click Save as at the top of the page. Here’s what the result looks like, along with an ROC curve:
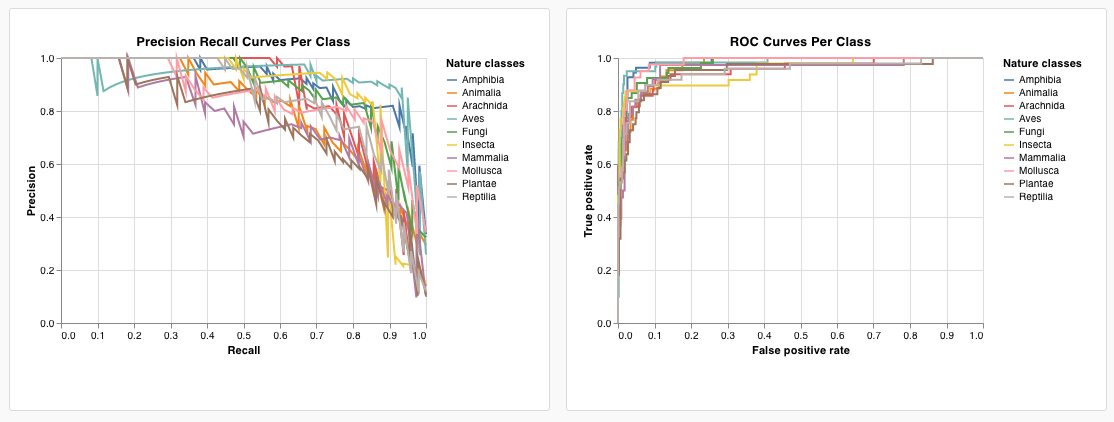
Bonus: Composite Histograms
Histograms can visualize numerical distributions to help us understand larger datasets. Composite histograms show multiple distributions across the same bins, letting us compare two or more metrics across different models or across different classes within our model. For a semantic segmentation model detecting objects in driving scenes, we might compare the effectiveness of optimizing for accuracy versus intersection over union (IOU), or we might want to know how well different models detect cars (large, common regions in the data) versus traffic signs (much smaller, less common regions). In the demo Colab, you can compare the confidence scores for two of the ten classes of living things.
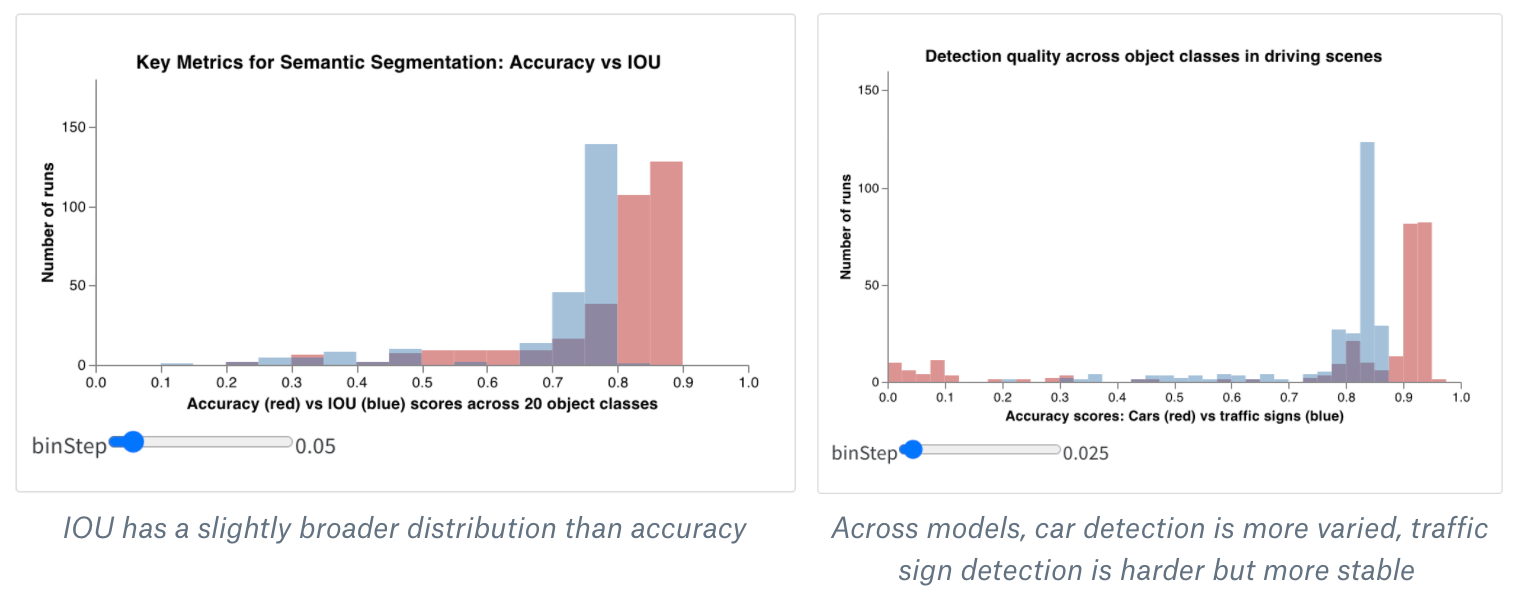
To create your own version of the custom composite histogram panel:
- Create a new Custom Chart panel in your Workspace or Report (by adding a “Custom Chart” visualization). Hit the “Edit” button in the top right to modify the Vega spec starting from any built-in panel type.
- Replace that built-in Vega spec with my MVP code for a composite histogram in Vega. You can modify the main title, axis titles, input domain, and any other details directly in this Vega spec using Vega syntax (you could change the colors or even add a third histogram :)
- Modify the query in the right hand side to load the correct data from your wandb logs. Add the field
summaryTable
and set the correspondingtableKey
toclass_scores
to fetch thewandb.Table
logged by your run. This will let you populate the two histogram bin sets (red_bins
andblue_bins
) via the dropdown menus with the columns of thewandb.Table
logged asclass_scores
. For my example, I chose theanimal
class prediction scores for the red bins andplant
for the blue bins. - You can keep making changes to the Vega spec and query until you’re happy with the plot you see in the preview rendering. Once you’re done, click Save as in the top and give your custom plot a name so you can reuse it. Then click Apply from panel library to finish your plot.
Here’s what my results look like from a very brief experiment: training on only 1000 examples for one epoch yields a model that’s very confident that most images are not plants and very uncertain about which images might be animals.
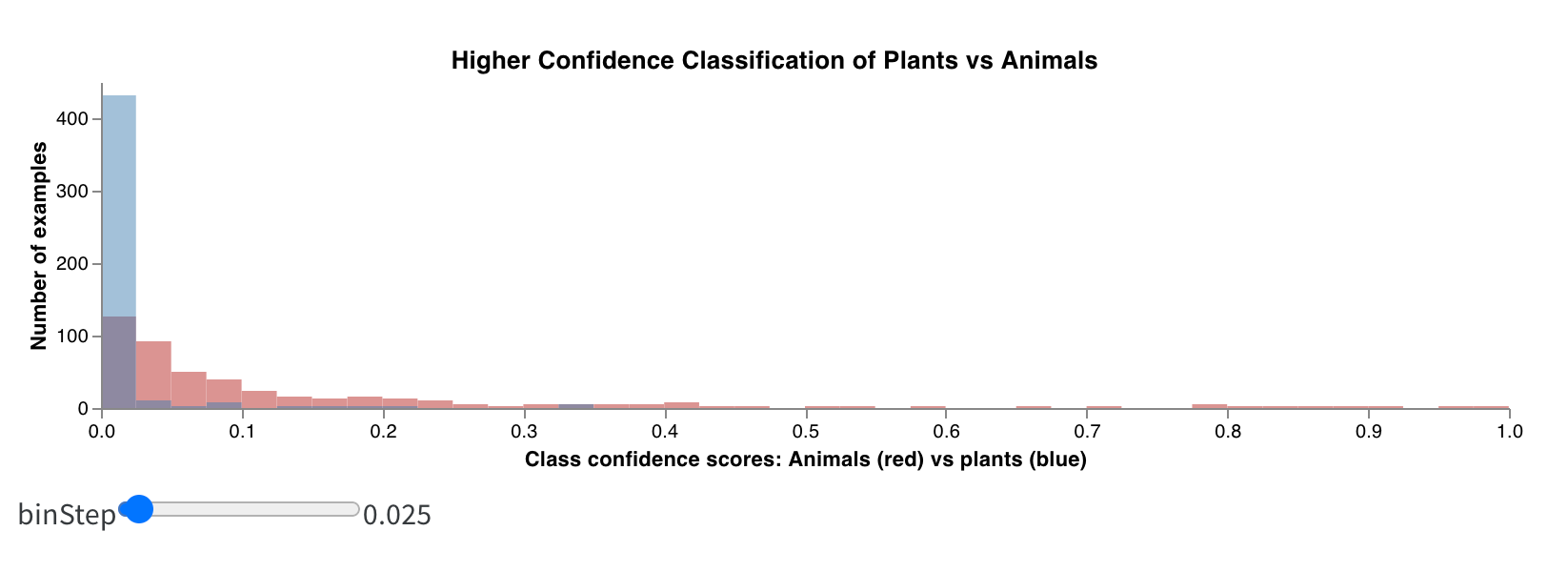
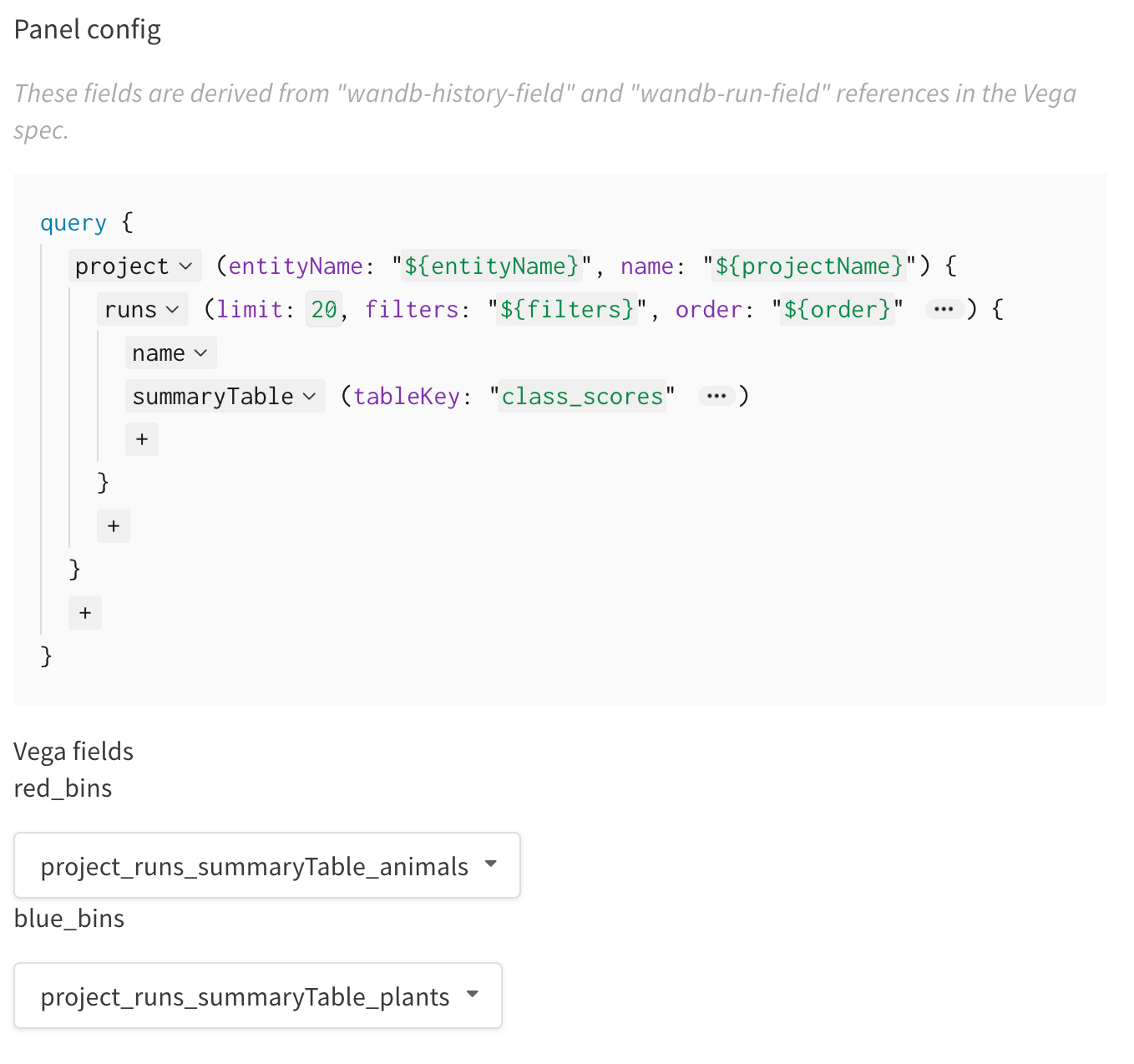
3 - Manage workspace, section, and panel settings
Within a given workspace page there are three different setting levels: workspaces, sections, and panels. Workspace settings apply to the entire workspace. Section settings apply to all panels within a section. Panel settings apply to individual panels.
Workspace settings
Workspace settings apply to all sections and all panels within those sections. You can edit two types of workspace settings: Workspace layout and Line plots. Workspace layouts determine the structure of the workspace, while Line plots settings control the default settings for line plots in the workspace.
To edit settings that apply to the overall structure of this workspace:
- Navigate to your project workspace.
- Click the gear icon next to the New report button to view the workspace settings.
- Choose Workspace layout to change the workspace’s layout, or choose Line plots to configure default settings for line plots in the workspace.
Workspace layout options
Configure a workspaces layout to define the overall structure of the workspace. This includes sectioning logic and panel organization.
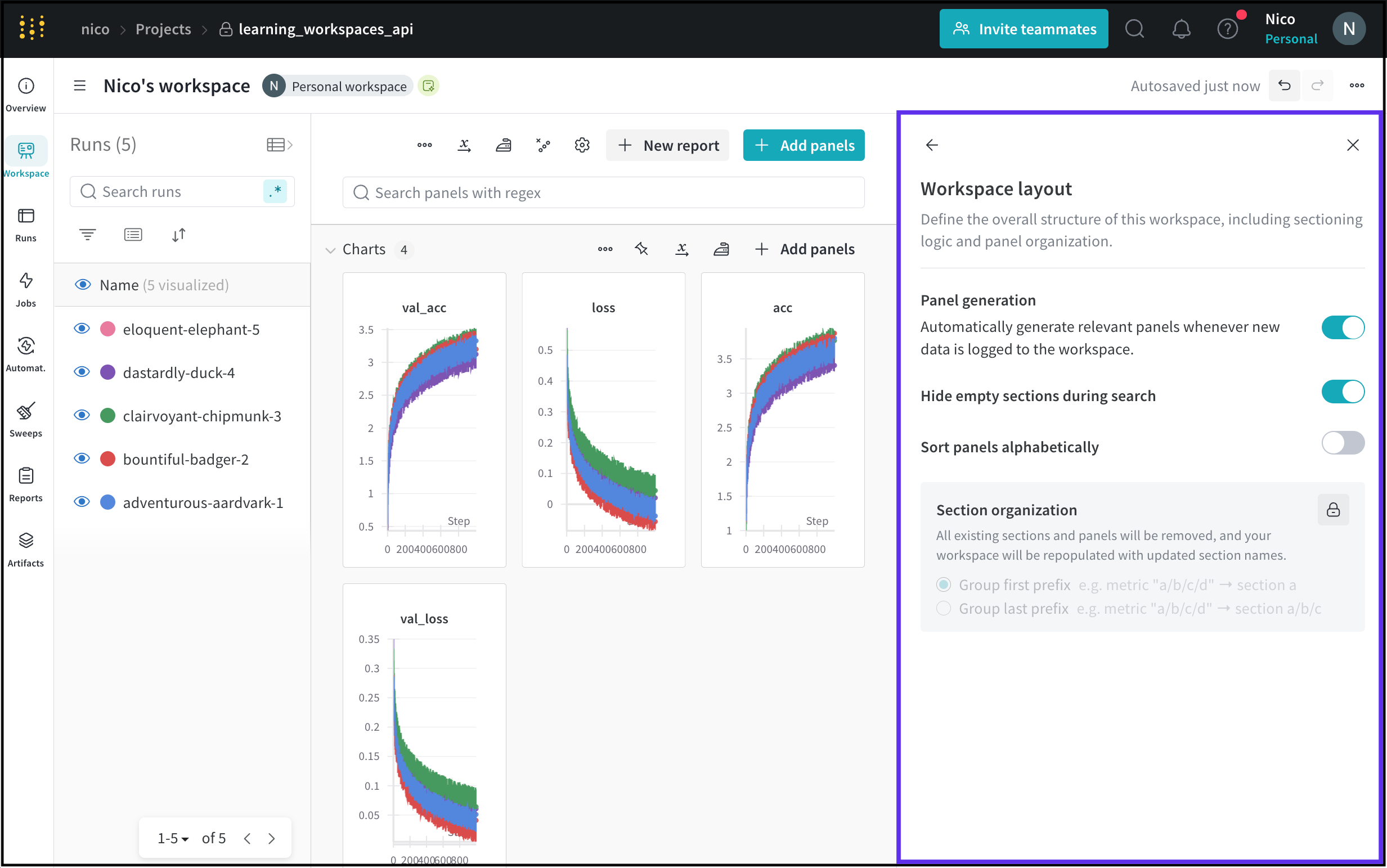
The workspace layout options page shows whether the workspace generates panels automatically or manually. To adjust a workspace’s panel generation mode, refer to Panels.
This table describes each workspace layout option.
Workspace setting | Description |
---|---|
Hide empty sections during search | Hide sections that do not contain any panels when searching for a panel. |
Sort panels alphabetically | Sort panels in your workspaces alphabetically. |
Section organization | Remove all existing sections and panels and repopulate them with new section names. Groups the newly populated sections either by first or last prefix. |
Line plots options
Set global defaults and custom rules for line plots in a workspace by modifying the Line plots workspace settings.
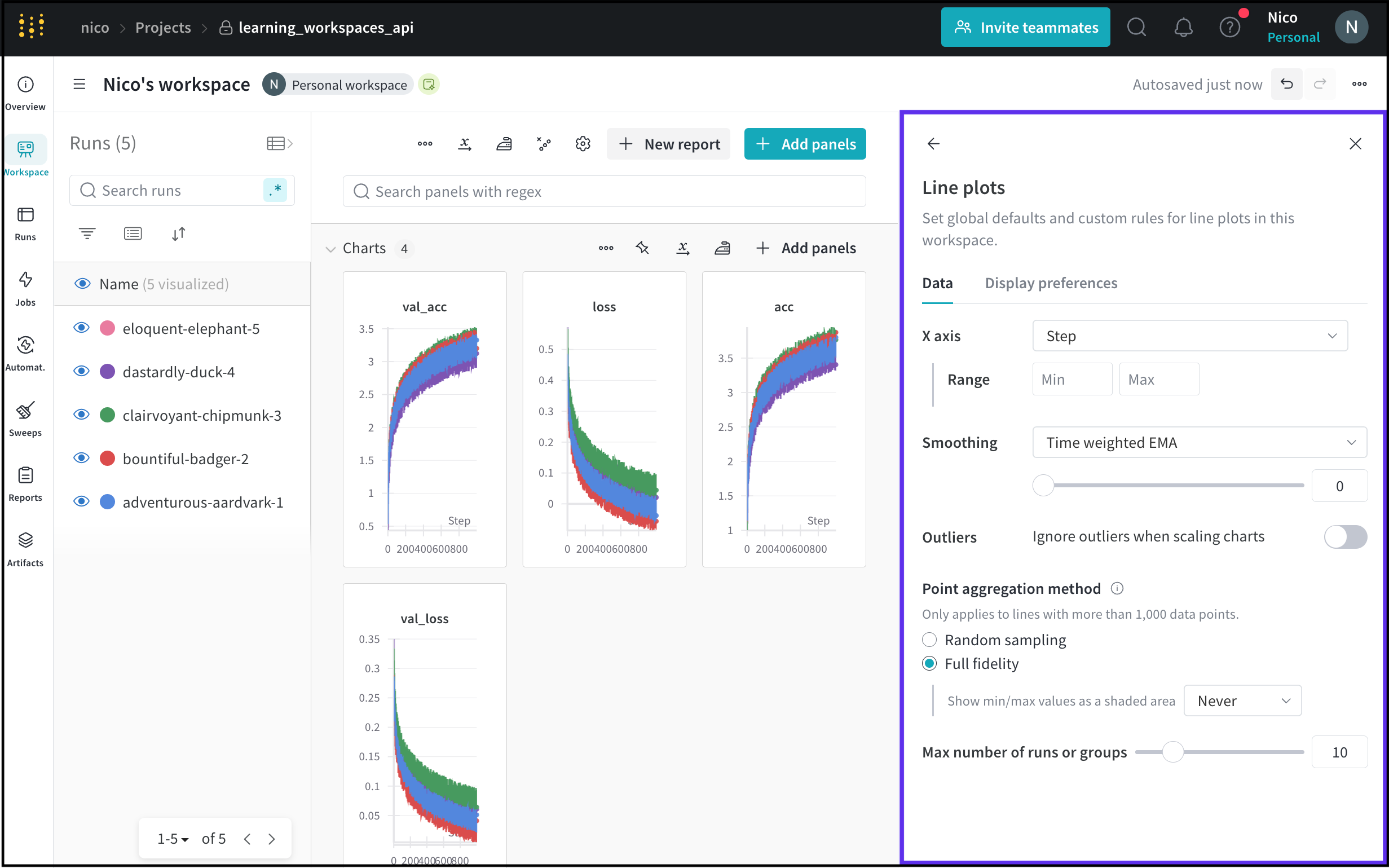
You can edit two main settings within Line plots settings: Data and Display preferences. The Data tab contains the following settings:
Line plot setting | Description |
---|---|
X axis | The scale of the x-axis in line plots. The x-axis is set to Step by default. See the proceeding table for the list of x-axis options. |
Range | Minimum and maximum settings to display for x axis. |
Smoothing | Change the smoothing on the line plot. For more information about smoothing, see Smooth line plots. |
Outliers | Rescale to exclude outliers from the default plot min and max scale. |
Point aggregation method | Improve data visualization accuracy and performance. See Point aggregation for more information. |
Max number of runs or groups | Limit the number of runs or groups displayed on the line plot. |
In addition to Step, there are other options for the x-axis:
X axis option | Description |
---|---|
Relative Time (Wall) | Timestamp since the process starts. For example, suppose start a run and resume that run the next day. If you then log something, the recorded point is 24 hours. |
Relative Time (Process) | Timestamp inside the running process. For example, suppose you start a run and let it continue for 10 seconds. The next day you resume that run. The point is recorded as 10 seconds. |
Wall Time | Minutes elapsed since the start of the first run on the graph. |
Step | Increments each time you call wandb.log() . |
Within the Display preferences tab, you can toggle the proceeding settings:
Display preference | Description |
---|---|
Remove legends from all panels | Remove the panel’s legend |
Display colored run names in tooltips | Show the runs as colored text within the tooltip |
Only show highlighted run in companion chart tooltip | Display only highlighted runs in chart tooltip |
Number of runs shown in tooltips | Display the number of runs in the tooltip |
Display full run names on the primary chart tooltip | Display the full name of the run in the chart tooltip |
Section settings
Section settings apply to all panels within that section. Within a workspace section you can sort panels, rearrange panels, and rename the section name.
Modify section settings by selecting the three horizontal dots (…) in the upper right corner of a section.
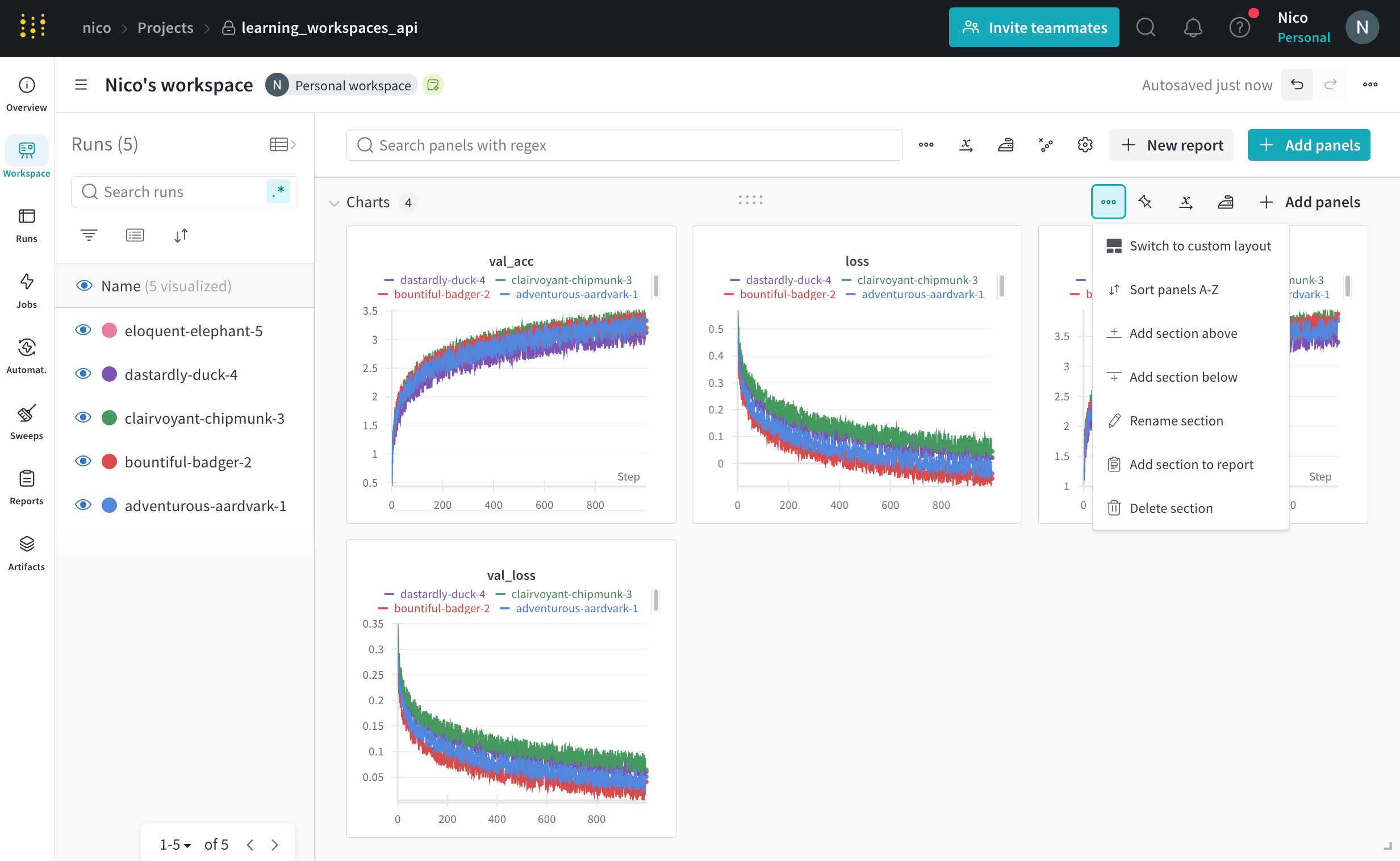
From the dropdown, you can edit the following settings that apply to the entire section:
Section setting | Description |
---|---|
Rename a section | Rename the name of the section |
Sort panels A-Z | Sort panels within a section alphabetically |
Rearrange panels | Select and drag a panel within a section to manually order your panels |
The proceeding animation demonstrates how to rearrange panels within a section:
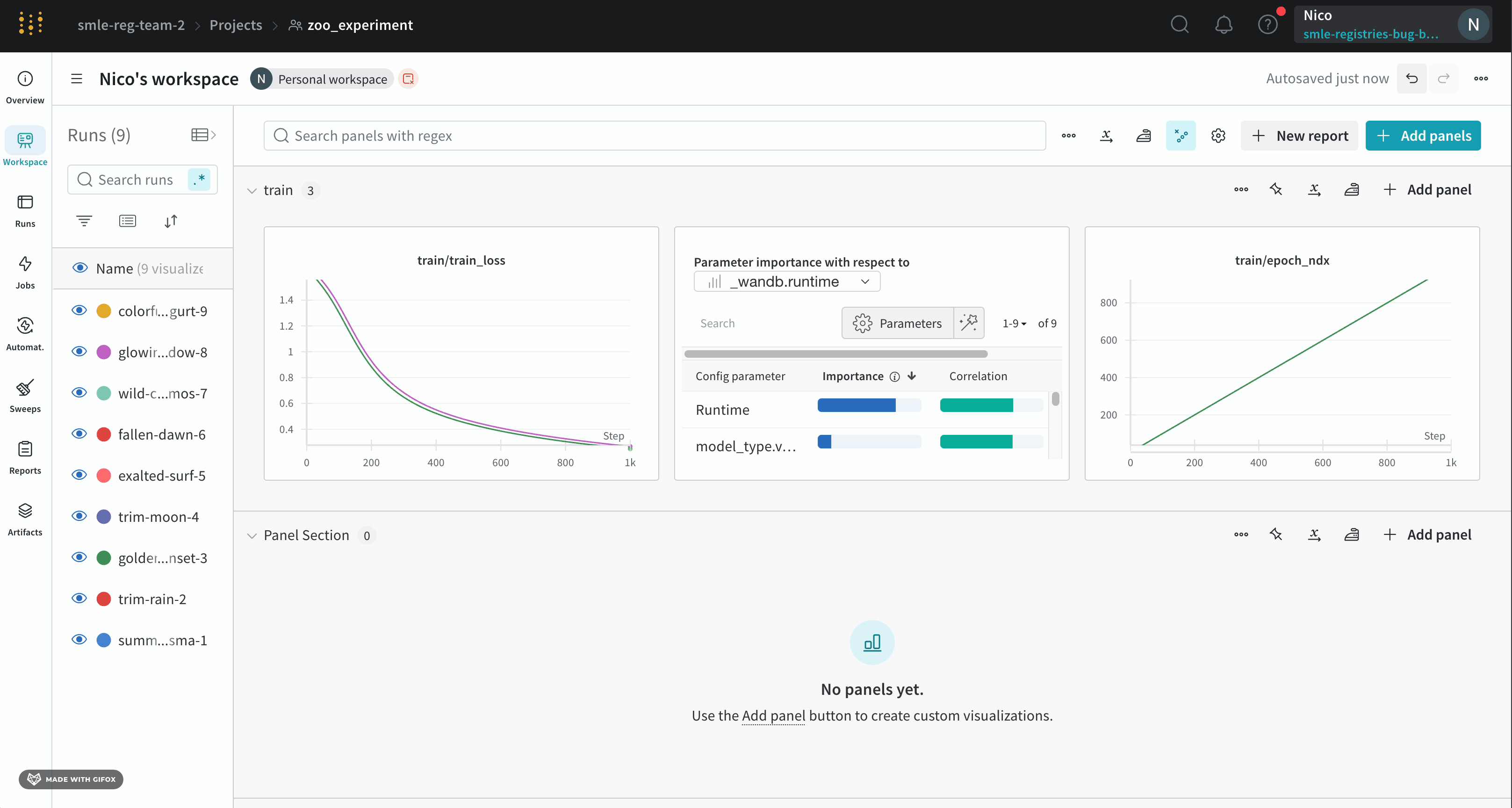
Panel settings
Customize an individual panel’s settings to compare multiple lines on the same plot, calculate custom axes, rename labels, and more. To edit a panel’s settings:
- Hover your mouse over the panel you want to edit.
- Select the pencil icon that appears.
- Within the modal that appears, you can edit settings related to the panel’s data, display preferences, and more.
For a complete list of settings you can apply to a panel, see Edit line panel settings.
4 - Settings
Within your individual user account you can edit: your profile picture, display name, geography location, biography information, emails associated to your account, and manage alerts for runs. You can also use the settings page to link your GitHub repository and delete your account. For more information, see User settings.
Use the team settings page to invite or remove new members to a team, manage alerts for team runs, change privacy settings, and view and manage storage usage. For more information about team settings, see Team settings.
4.1 - Manage user settings
Navigate to your user profile page and select your user icon on the top right corner. From the dropdown, choose Settings.
Profile
Within the Profile section you can manage and modify your account name and institution. You can optionally add a biography, location, link to a personal or your institution’s website, and upload a profile image.
Teams
Create a new team in the Team section. To create a new team, select the New team button and provide the following:
- Team name - the name of your team. The team mane must be unique. Team names can not be changed.
- Team type - Select either the Work or Academic button.
- Company/Organization - Provide the name of the team’s company or organization. Choose the dropdown menu to select a company or organization. You can optionally provide a new organization.
Beta features
Within the Beta Features section you can optionally enable fun add-ons and sneak previews of new products in development. Select the toggle switch next to the beta feature you want to enable.
Alerts
Get notified when your runs crash, finish, or set custom alerts with wandb.alert(). Receive notifications either through Email or Slack. Toggle the switch next to the event type you want to receive alerts from.
- Runs finished: whether a Weights and Biases run successfully finished.
- Run crashed: notification if a run has failed to finish.
For more information about how to set up and manage alerts, see Send alerts with wandb.alert.
Personal GitHub integration
Connect a personal Github account. To connect a Github account:
- Select the Connect Github button. This will redirect you to an open authorization (OAuth) page.
- Select the organization to grant access in the Organization access section.
- Select Authorize wandb.
Delete your account
Select the Delete Account button to delete your account.
Storage
The Storage section describes the total memory usage the your account has consumed on the Weights and Biases servers. The default storage plan is 100GB. For more information about storage and pricing, see the Pricing page.
4.2 - Manage billing settings
Navigate to your user profile page and select your user icon on the top right corner. From the dropdown, choose Billing, or choose Settings and then select the Billing tab.
Plan details
The Plan details section summarizes your organization’s current plan, charges, limits, and usage.
- For details and a list of users, click Manage users.
- For details about usage, click View usage.
- Amount of storage your organization uses, both free and paid. From here, you can purchase additional storage and manage storage that is currently in use. Learn more about storage settings.
From here, you can compare plans or talk to Sales.
Plan usage
This section visually summarizes current usage and displays upcoming usage charges. For detailed insights into usage by month, click View usage on an individual tile. To export usage by calendar month, team, or project, click Export CSV.
Usage alerts
For organizations on paid plans, admins receive alerts via email once per billing period when certain thresholds are met, along with details about how to increase your organization’s limits if you are a billing admin and how to contact a billing admin otherwise. On the Pro plan, only the billing admin receives usage alerts.
These alerts are not configurable, and are sent when:
- Your organization is approaching a monthly limit of a category of usage (85% of hours used) and when it reaches 100% of the limit, according to your plan.
- Your organization’s accumulated average charges for a billing period exceed these thresholds: $200, $450, $700, and $1000. These overage charges are incurred when your organization accumulates more usage than your plan includes for tracked hours, storage, or Weave data ingestion.
For questions about usage or billing, contact your account team or Support.
Payment methods
This section shows the payment methods on file for your organization. If you have not added a payment method, you will be prompted to do so when you upgrade your plan or add paid storage.
Billing admin
This section shows the current billing admin. The billing admin is an organization admin, receives all billing-related emails, and can view and manage payment methods.
To change the billing admin or assign the role to additional users:
- Click Manage roles.
- Search for a user.
- Click the Billing admin field in that user’s row.
- Read the summary, then click Change billing user.
Invoices
If you pay using a credit card, this section allows you to view monthly invoices.
- For Enterprise accounts that pay via wire transfer, this section is blank. For questions, contact your account team.
- If your organization incurs no charges, no invoice is generated.
4.3 - Manage team settings
Team settings
Change your team’s settings, including members, avatar, alerts, privacy, and usage. Organization admins and team admins can view and edit a team’s settings.
Members
The Members section shows a list of all pending invitations and the members that have either accepted the invitation to join the team. Each member listed displays a member’s name, username, email, team role, as well as their access privileges to Models and Weave, which is inherited by from the Organization. You can choose from the standard team roles Admin, Member, and View-only. If your organization has created custom roles, you can assign a custom role instead.
See Add and Manage teams for information on how to create a team, manage teams, and manage team membership and roles.
Avatar
Set an avatar by navigating to the Avatar section and uploading an image.
- Select the Update Avatar to prompt a file dialog to appear.
- From the file dialog, choose the image you want to use.
Alerts
Notify your team when runs crash, finish, or set custom alerts. Your team can receive alerts either through email or Slack.
Toggle the switch next to the event type you want to receive alerts from. Weights and Biases provides the following event type options be default:
- Runs finished: whether a Weights and Biases run successfully finished.
- Run crashed: if a run has failed to finish.
For more information about how to set up and manage alerts, see Send alerts with wandb.alert.
Privacy
Navigate to the Privacy section to change privacy settings. Only organization admins can modify privacy setting, including:
- Force projects in the team to be private.
- Enable code saving by default.
Usage
The Usage section describes the total memory usage the team has consumed on the Weights and Biases servers. The default storage plan is 100GB. For more information about storage and pricing, see the Pricing page.
Storage
The Storage section describes the cloud storage bucket configuration that is being used for the team’s data. For more information, see Secure Storage Connector or check out our W&B Server docs if you are self-hosting.
4.4 - Manage email settings
Add, delete, manage email types and primary email addresses in your W&B Profile Settings page. Select your profile icon in the upper right corner of the W&B dashboard. From the dropdown, select Settings. Within the Settings page, scroll down to the Emails dashboard:
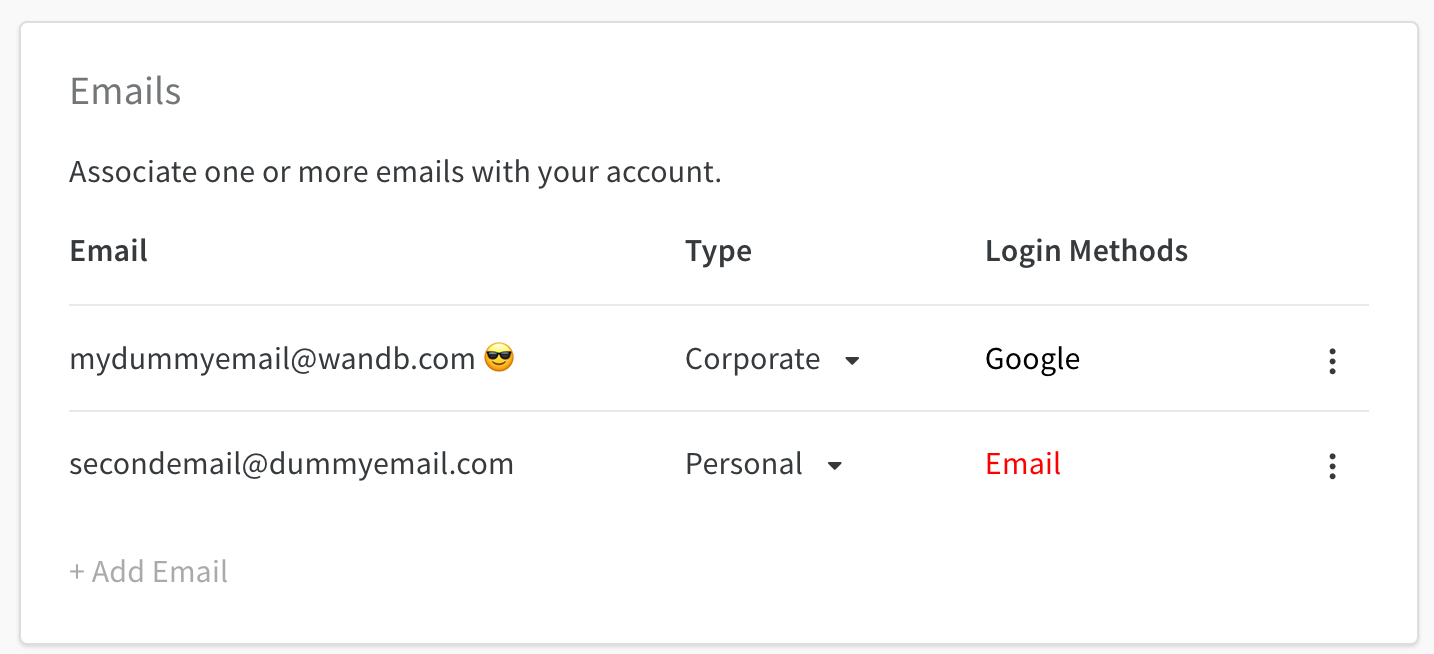
Manage primary email
The primary email is marked with a 😎 emoji. The primary email is automatically defined with the email you provided when you created a W&B account.
Select the kebab dropdown to change the primary email associated with your Weights And Biases account:
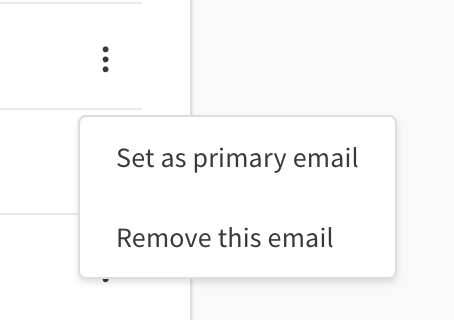
Add emails
Select + Add Email to add an email. This will take you to an Auth0 page. You can enter in the credentials for the new email or connect using single sign-on (SSO).
Delete emails
Select the kebab dropdown and choose Delete Emails to delete an email that is registered to your W&B account
Log in methods
The Log in Methods column displays the log in methods that are associated with your account.
A verification email is sent to your email account when you create a W&B account. Your email account is considered unverified until you verify your email address. Unverified emails are displayed in red.
Attempt to log in with your email address again to retrieve a second verification email if you no longer have the original verification email that was sent to your email account.
Contact support@wandb.com for account log in issues.
4.5 - Manage teams
Use W&B Teams as a central workspace for your ML team to build better models faster.
- Track all the experiments your team has tried so you never duplicate work.
- Save and reproduce previously trained models.
- Share progress and results with your boss and collaborators.
- Catch regressions and immediately get alerted when performance drops.
- Benchmark model performance and compare model versions.
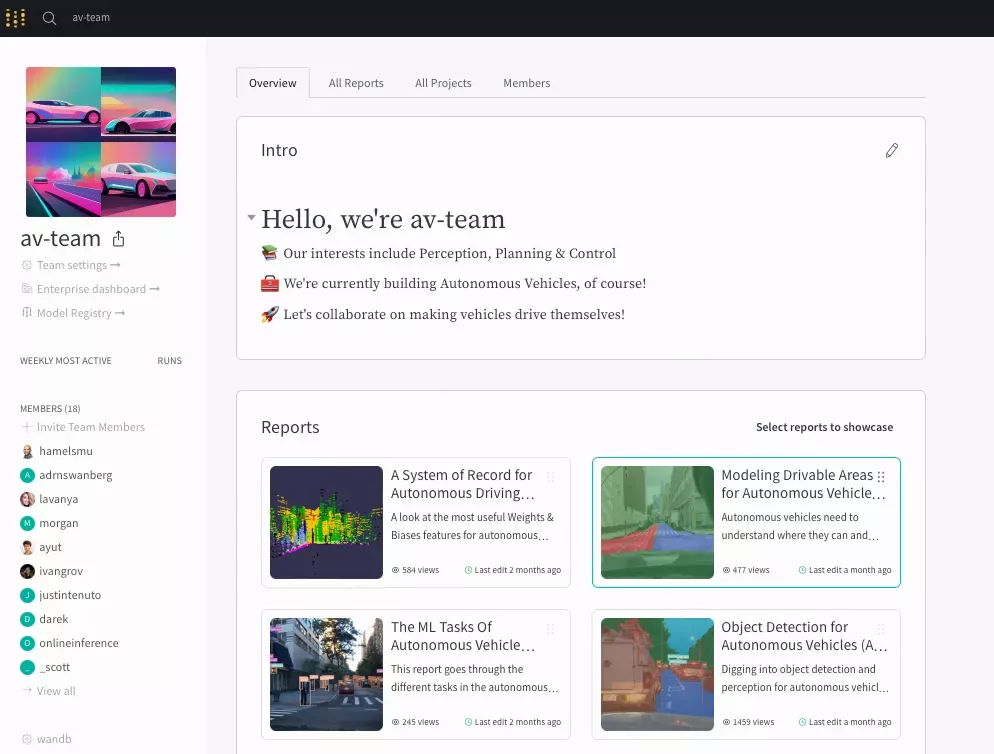
Create a collaborative team
- Sign up or log in to your free W&B account.
- Click Invite Team in the navigation bar.
- Create your team and invite collaborators.
Create a team profile
You can customize your team’s profile page to show an introduction and showcase reports and projects that are visible to the public or team members. Present reports, projects, and external links.
- Highlight your best research to visitors by showcasing your best public reports
- Showcase the most active projects to make it easier for teammates to find them
- Find collaborators by adding external links to your company or research lab’s website and any papers you’ve published
Remove team members
Team admins can open the team settings page and click the delete button next to the departing member’s name. Any runs logged to the team remain after a user leaves.
Manage team roles and permissions
Select a team role when you invite colleagues to join a team. There are following team role options:
- Admin: Team admins can add and remove other admins or team members. They have permissions to modify all projects and full deletion permissions. This includes, but is not limited to, deleting runs, projects, artifacts, and sweeps.
- Member: A regular member of the team. An admin invites a team member by email. A team member cannot invite other members. Team members can only delete runs and sweep runs created by that member. Suppose you have two members A and B. Member B moves a Run from team B’s project to a different project owned by Member A. Member A can not delete the Run Member B moved to Member A’s project. Only the member that creates the Run, or the team admin, can delete the run.
- View-Only (Enterprise-only feature): View-Only members can view assets within the team such as runs, reports, and workspaces. They can follow and comment on reports, but they can not create, edit, or delete project overview, reports, or runs.
- Custom roles (Enterprise-only feature): Custom roles allow organization admins to compose new roles based on either of the View-Only or Member roles, together with additional permissions to achieve fine-grained access control. Team admins can then assign any of those custom roles to users in their respective teams. Refer to Introducing Custom Roles for W&B Teams for details.
- Service accounts (Enterprise-only feature): Refer to Use service accounts to automate workflows.
Team settings
Team settings allow you to manage the settings for your team and its members. With these privileges, you can effectively oversee and organize your team within W&B.
Permissions | View-Only | Team Member | Team Admin |
---|---|---|---|
Add team members | X | ||
Remove team members | X | ||
Manage team settings | X |
Model Registry
The proceeding table lists permissions that apply to all projects across a given team.
Permissions | View-Only | Team Member | Model Registry Admin | Team Admin |
---|---|---|---|---|
Add aliases | X | X | X | |
Add models to the registry | X | X | X | |
View models in the registry | X | X | X | X |
Download models | X | X | X | X |
Add/Remove Registry Admins | X | X | ||
Add/Remove Protected Aliases | X |
See the Model Registry chapter for more information about protected aliases.
Reports
Report permissions grant access to create, view, and edit reports. The proceeding table lists permissions that apply to all reports across a given team.
Permissions | View-Only | Team Member | Team Admin |
---|---|---|---|
View reports | X | X | X |
Create reports | X | X | |
Edit reports | X (team members can only edit their own reports) | X | |
Delete reports | X (team members can only edit their own reports) | X |
Experiments
The proceeding table lists permissions that apply to all experiments across a given team.
Permissions | View-Only | Team Member | Team Admin |
---|---|---|---|
View experiment metadata (includes history metrics, system metrics, files, and logs) | X | X | X |
Edit experiment panels and workspaces | X | X | |
Log experiments | X | X | |
Delete experiments | X (team members can only delete experiments they created) | X | |
Stop experiments | X (team members can only stop experiments they created) | X |
Artifacts
The proceeding table lists permissions that apply to all artifacts across a given team.
Permissions | View-Only | Team Member | Team Admin |
---|---|---|---|
View artifacts | X | X | X |
Create artifacts | X | X | |
Delete artifacts | X | X | |
Edit metadata | X | X | |
Edit aliases | X | X | |
Delete aliases | X | X | |
Download artifact | X | X |
System settings (W&B Server only)
Use system permissions to create and manage teams and their members and to adjust system settings. These privileges enable you to effectively administer and maintain the W&B instance.
Permissions | View-Only | Team Member | Team Admin | System Admin |
---|---|---|---|---|
Configure system settings | X | |||
Create/delete teams | X |
Team service account behavior
- When you configure a team in your training environment, you can use a service account from that team to log runs in either of private or public projects within that team. Additionally, you can attribute those runs to a user if WANDB_USERNAME or WANDB_USER_EMAIL variable exists in your environment and the referenced user is part of that team.
- When you do not configure a team in your training environment and use a service account, the runs log to the named project within that service account’s parent team. In this case as well, you can attribute the runs to a user if WANDB_USERNAME or WANDB_USER_EMAIL variable exists in your environment and the referenced user is part of the service account’s parent team.
- A service account can not log runs to a private project in a team different from its parent team. A service account can log to runs to project only if the project is set to
Open
project visibility.
Add social badges to your intro
In your Intro, type /
and choose Markdown and paste the markdown snippet that renders your badge. Once you convert it to WYSIWYG, you can resize it.
For example, to add a Twitter follow badge, add [
replacing ](https://twitter.com/intent/follow?screen_name=weights_biases
weights_biases
with your Twitter username.
Team trials
See the pricing page for more information on W&B plans. You can download all your data at any time, either using the dashboard UI or the Export API.
Privacy settings
You can see the privacy settings of all team projects on the team settings page:
app.wandb.ai/teams/your-team-name
Advanced configuration
Secure storage connector
The team-level secure storage connector allows teams to use their own cloud storage bucket with W&B. This provides greater data access control and data isolation for teams with highly sensitive data or strict compliance requirements. Refer to Secure Storage Connector for more information.
4.6 - Manage storage
If you are approaching or exceeding your storage limit, there are multiple paths forward to manage your data. The path that’s best for you will depend on your account type and your current project setup.
Manage storage consumption
W&B offers different methods of optimizing your storage consumption:
- Use reference artifacts to track files saved outside the W&B system, instead of uploading them to W&B storage.
- Use an external cloud storage bucket for storage. (Enterprise only)
Delete data
You can also choose to delete data to remain under your storage limit. There are several ways to do this:
- Delete data interactively with the app UI.
- Set a TTL policy on Artifacts so they are automatically deleted.
4.7 - System metrics
This page provides detailed information about the system metrics that are tracked by the W&B SDK.
wandb
automatically logs system metrics every 10 seconds.CPU
Process CPU Percent (CPU)
Percentage of CPU usage by the process, normalized by the number of available CPUs.
W&B assigns a cpu
tag to this metric.
CPU Percent
CPU usage of the system on a per-core basis.
W&B assigns a cpu.{i}.cpu_percent
tag to this metric.
Process CPU Threads
The number of threads utilized by the process.
W&B assigns a proc.cpu.threads
tag to this metric.
Disk
By default, the usage metrics are collected for the /
path. To configure the paths to be monitored, use the following setting:
run = wandb.init(
settings=wandb.Settings(
_stats_disk_paths=("/System/Volumes/Data", "/home", "/mnt/data"),
),
)
Disk Usage Percent
Represents the total system disk usage in percentage for specified paths.
W&B assigns a disk.{path}.usagePercen
tag to this metric.
Disk Usage
Represents the total system disk usage in gigabytes (GB) for specified paths. The paths that are accessible are sampled, and the disk usage (in GB) for each path is appended to the samples.
W&B assigns a disk.{path}.usageGB)
tag to this metric.
Disk In
Indicates the total system disk read in megabytes (MB). The initial disk read bytes are recorded when the first sample is taken. Subsequent samples calculate the difference between the current read bytes and the initial value.
W&B assigns a disk.in
tag to this metric.
Disk Out
Represents the total system disk write in megabytes (MB). Similar to Disk In, the initial disk write bytes are recorded when the first sample is taken. Subsequent samples calculate the difference between the current write bytes and the initial value.
W&B assigns a disk.out
tag to this metric.
Memory
Process Memory RSS
Represents the Memory Resident Set Size (RSS) in megabytes (MB) for the process. RSS is the portion of memory occupied by a process that is held in main memory (RAM).
W&B assigns a proc.memory.rssMB
tag to this metric.
Process Memory Percent
Indicates the memory usage of the process as a percentage of the total available memory.
W&B assigns a proc.memory.percent
tag to this metric.
Memory Percent
Represents the total system memory usage as a percentage of the total available memory.
W&B assigns a memory
tag to this metric.
Memory Available
Indicates the total available system memory in megabytes (MB).
W&B assigns a proc.memory.availableMB
tag to this metric.
Network
Network Sent
Represents the total bytes sent over the network. The initial bytes sent are recorded when the metric is first initialized. Subsequent samples calculate the difference between the current bytes sent and the initial value.
W&B assigns a network.sent
tag to this metric.
Network Received
Indicates the total bytes received over the network. Similar to Network Sent, the initial bytes received are recorded when the metric is first initialized. Subsequent samples calculate the difference between the current bytes received and the initial value.
W&B assigns a network.recv
tag to this metric.
NVIDIA GPU
In addition to the metrics described below, if the process and/or its children use a particular GPU, W&B captures the corresponding metrics as gpu.process.{gpu_index}...
GPU Memory Utilization
Represents the GPU memory utilization in percent for each GPU.
W&B assigns a gpu.{gpu_index}.memory
tag to this metric.
GPU Memory Allocated
Indicates the GPU memory allocated as a percentage of the total available memory for each GPU.
W&B assigns a gpu.{gpu_index}.memoryAllocated
tag to this metric.
GPU Memory Allocated Bytes
Specifies the GPU memory allocated in bytes for each GPU.
W&B assigns a gpu.{gpu_index}.memoryAllocatedBytes
tag to this metric.
GPU Utilization
Reflects the GPU utilization in percent for each GPU.
W&B assigns a gpu.{gpu_index}.gpu
tag to this metric.
GPU Temperature
The GPU temperature in Celsius for each GPU.
W&B assigns a gpu.{gpu_index}.temp
tag to this metric.
GPU Power Usage Watts
Indicates the GPU power usage in Watts for each GPU.
W&B assigns a gpu.{gpu_index}.powerWatts
tag to this metric.
GPU Power Usage Percent
Reflects the GPU power usage as a percentage of its power capacity for each GPU.
W&B assigns a gpu.{gpu_index}.powerPercent
tag to this metric.
GPU SM Clock Speed
Represents the clock speed of the Streaming Multiprocessor (SM) on the GPU in MHz. This metric is indicative of the processing speed within the GPU cores responsible for computation tasks.
W&B assigns a gpu.{gpu_index}.smClock
tag to this metric.
GPU Memory Clock Speed
Represents the clock speed of the GPU memory in MHz, which influences the rate of data transfer between the GPU memory and processing cores.
W&B assigns a gpu.{gpu_index}.memoryClock
tag to this metric.
GPU Graphics Clock Speed
Represents the base clock speed for graphics rendering operations on the GPU, expressed in MHz. This metric often reflects performance during visualization or rendering tasks.
W&B assigns a gpu.{gpu_index}.graphicsClock
tag to this metric.
GPU Corrected Memory Errors
Tracks the count of memory errors on the GPU that W&B automatically corrects by error-checking protocols, indicating recoverable hardware issues.
W&B assigns a gpu.{gpu_index}.correctedMemoryErrors
tag to this metric.
GPU Uncorrected Memory Errors
Tracks the count of memory errors on the GPU that W&B uncorrected, indicating non-recoverable errors which can impact processing reliability.
W&B assigns a gpu.{gpu_index}.unCorrectedMemoryErrors
tag to this metric.
GPU Encoder Utilization
Represents the percentage utilization of the GPU’s video encoder, indicating its load when encoding tasks (for example, video rendering) are running.
W&B assigns a gpu.{gpu_index}.encoderUtilization
tag to this metric.
AMD GPU
W&B extracts metrics from the output of the rocm-smi
tool supplied by AMD (rocm-smi -a --json
).
AMD GPU Utilization
Represents the GPU utilization in percent for each AMD GPU device.
W&B assigns a gpu.{gpu_index}.gpu
tag to this metric.
AMD GPU Memory Allocated
Indicates the GPU memory allocated as a percentage of the total available memory for each AMD GPU device.
W&B assigns a gpu.{gpu_index}.memoryAllocated
tag to this metric.
AMD GPU Temperature
The GPU temperature in Celsius for each AMD GPU device.
W&B assigns a gpu.{gpu_index}.temp
tag to this metric.
AMD GPU Power Usage Watts
The GPU power usage in Watts for each AMD GPU device.
W&B assigns a gpu.{gpu_index}.powerWatts
tag to this metric.
AMD GPU Power Usage Percent
Reflects the GPU power usage as a percentage of its power capacity for each AMD GPU device.
W&B assigns a gpu.{gpu_index}.powerPercent
to this metric.
Apple ARM Mac GPU
Apple GPU Utilization
Indicates the GPU utilization in percent for Apple GPU devices, specifically on ARM Macs.
W&B assigns a gpu.0.gpu
tag to this metric.
Apple GPU Memory Allocated
The GPU memory allocated as a percentage of the total available memory for Apple GPU devices on ARM Macs.
W&B assigns a gpu.0.memoryAllocated
tag to this metric.
Apple GPU Temperature
The GPU temperature in Celsius for Apple GPU devices on ARM Macs.
W&B assigns a gpu.0.temp
tag to this metric.
Apple GPU Power Usage Watts
The GPU power usage in Watts for Apple GPU devices on ARM Macs.
W&B assigns a gpu.0.powerWatts
tag to this metric.
Apple GPU Power Usage Percent
The GPU power usage as a percentage of its power capacity for Apple GPU devices on ARM Macs.
W&B assigns a gpu.0.powerPercent
tag to this metric.
Graphcore IPU
Graphcore IPUs (Intelligence Processing Units) are unique hardware accelerators designed specifically for machine intelligence tasks.
IPU Device Metrics
These metrics represent various statistics for a specific IPU device. Each metric has a device ID (device_id
) and a metric key (metric_key
) to identify it. W&B assigns a ipu.{device_id}.{metric_key}
tag to this metric.
Metrics are extracted using the proprietary gcipuinfo
library, which interacts with Graphcore’s gcipuinfo
binary. The sample
method fetches these metrics for each IPU device associated with the process ID (pid
). Only the metrics that change over time, or the first time a device’s metrics are fetched, are logged to avoid logging redundant data.
For each metric, the method parse_metric
is used to extract the metric’s value from its raw string representation. The metrics are then aggregated across multiple samples using the aggregate
method.
The following lists available metrics and their units:
- Average Board Temperature (
average board temp (C)
): Temperature of the IPU board in Celsius. - Average Die Temperature (
average die temp (C)
): Temperature of the IPU die in Celsius. - Clock Speed (
clock (MHz)
): The clock speed of the IPU in MHz. - IPU Power (
ipu power (W)
): Power consumption of the IPU in Watts. - IPU Utilization (
ipu utilisation (%)
): Percentage of IPU utilization. - IPU Session Utilization (
ipu utilisation (session) (%)
): IPU utilization percentage specific to the current session. - Data Link Speed (
speed (GT/s)
): Speed of data transmission in Giga-transfers per second.
Google Cloud TPU
Tensor Processing Units (TPUs) are Google’s custom-developed ASICs (Application Specific Integrated Circuits) used to accelerate machine learning workloads.
TPU Memory usage
The current High Bandwidth Memory usage in bytes per TPU core.
W&B assigns a tpu.{tpu_index}.memoryUsageBytes
tag to this metric.
TPU Memory usage percentage
The current High Bandwidth Memory usage in percent per TPU core.
W&B assigns a tpu.{tpu_index}.memoryUsageBytes
tag to this metric.
TPU Duty cycle
TensorCore duty cycle percentage per TPU device. Tracks the percentage of time over the sample period during which the accelerator TensorCore was actively processing. A larger value means better TensorCore utilization.
W&B assigns a tpu.{tpu_index}.dutyCycle
tag to this metric.
AWS Trainium
AWS Trainium is a specialized hardware platform offered by AWS that focuses on accelerating machine learning workloads. The neuron-monitor
tool from AWS is used to capture the AWS Trainium metrics.
Trainium Neuron Core Utilization
The utilization percentage of each NeuronCore, reported on a per-core basis.
W&B assigns a trn.{core_index}.neuroncore_utilization
tag to this metric.
Trainium Host Memory Usage, Total
The total memory consumption on the host in bytes.
W&B assigns a trn.host_total_memory_usage
tag to this metric.
Trainium Neuron Device Total Memory Usage
The total memory usage on the Neuron device in bytes.
W&B assigns a trn.neuron_device_total_memory_usage)
tag to this metric.
Trainium Host Memory Usage Breakdown:
The following is a breakdown of memory usage on the host:
- Application Memory (
trn.host_total_memory_usage.application_memory
): Memory used by the application. - Constants (
trn.host_total_memory_usage.constants
): Memory used for constants. - DMA Buffers (
trn.host_total_memory_usage.dma_buffers
): Memory used for Direct Memory Access buffers. - Tensors (
trn.host_total_memory_usage.tensors
): Memory used for tensors.
Trainium Neuron Core Memory Usage Breakdown
Detailed memory usage information for each NeuronCore:
- Constants (
trn.{core_index}.neuroncore_memory_usage.constants
) - Model Code (
trn.{core_index}.neuroncore_memory_usage.model_code
) - Model Shared Scratchpad (
trn.{core_index}.neuroncore_memory_usage.model_shared_scratchpad
) - Runtime Memory (
trn.{core_index}.neuroncore_memory_usage.runtime_memory
) - Tensors (
trn.{core_index}.neuroncore_memory_usage.tensors
)
OpenMetrics
Capture and log metrics from external endpoints that expose OpenMetrics / Prometheus-compatible data with support for custom regex-based metric filters to be applied to the consumed endpoints.
Refer to this report for a detailed example of how to use this feature in a particular case of monitoring GPU cluster performance with the NVIDIA DCGM-Exporter.
4.8 - Anonymous mode
Are you publishing code that you want anyone to be able to run easily? Use anonymous mode to let someone run your code, see a W&B dashboard, and visualize results without needing to create a W&B account first.
Allow results to be logged in anonymous mode with:
import wandb
wandb.init(anonymous="allow")
For example, the proceeding code snippet shows how to create and log an artifact with W&B:
import wandb
run = wandb.init(anonymous="allow")
artifact = wandb.Artifact(name="art1", type="foo")
artifact.add_file(local_path="path/to/file")
run.log_artifact(artifact)
run.finish()
Try the example notebook to see how anonymous mode works.