Use query panels to query and interactively visualize your data.
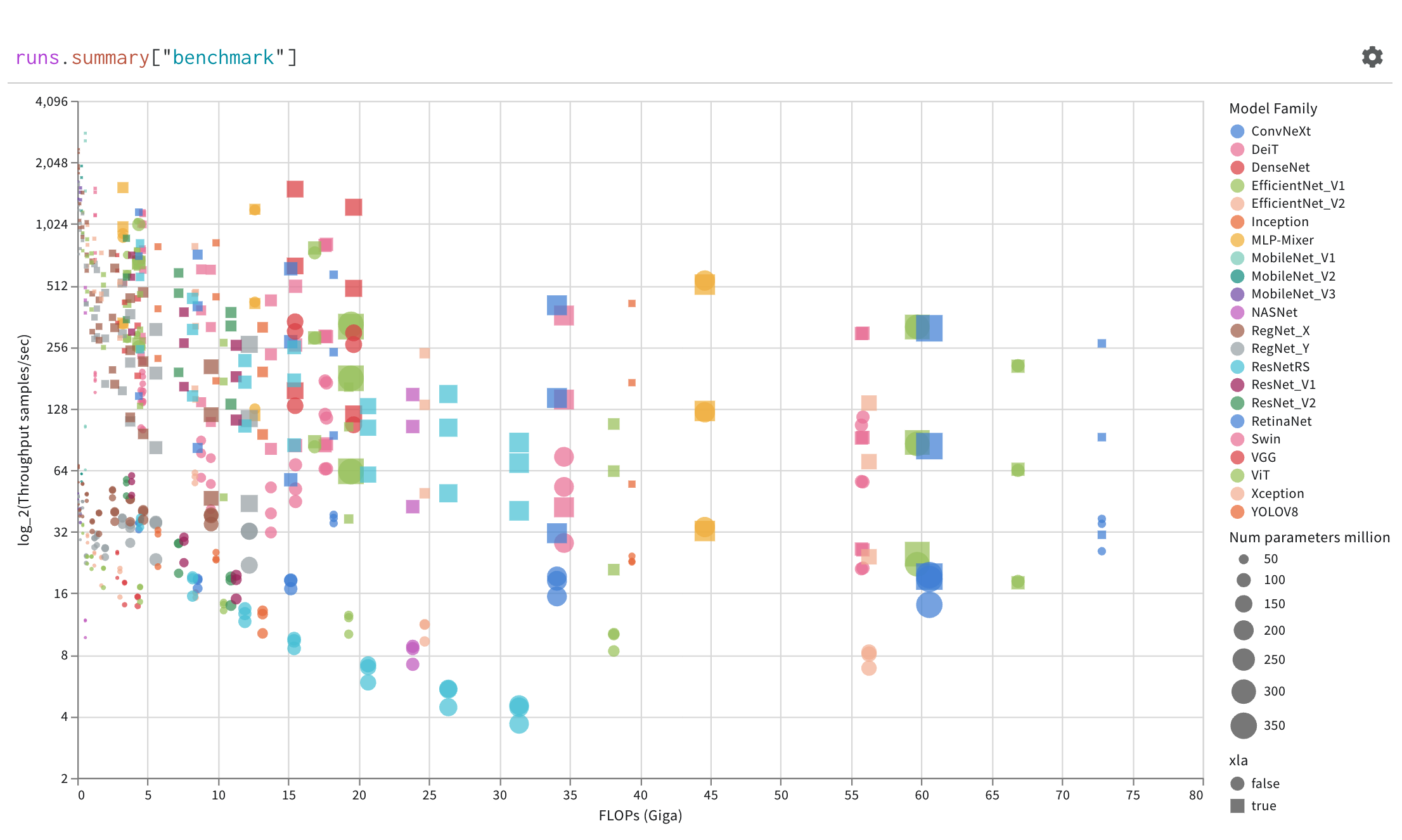
Create a query panel
Add a query to your workspace or within a report.
- Navigate to your project’s workspace.
- In the upper right hand corner, click
Add panel
. - From the dropdown, select
Query panel
.
Type and select /Query panel
.
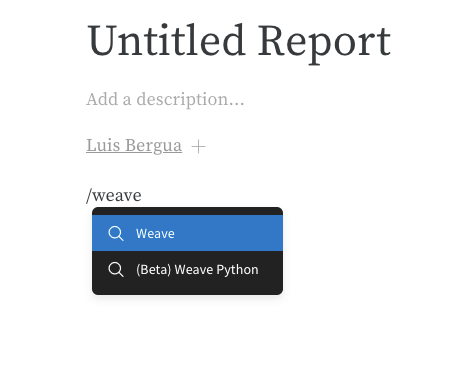
Alternatively, you can associate a query with a set of runs:
- Within your report, type and select
/Panel grid
. - Click the
Add panel
button. - From the dropdown, select
Query panel
.
Query components
Expressions
Use query expressions to query your data stored in W&B such as runs, artifacts, models, tables, and more.
Example: Query a table
Suppose you want to query a W&B Table. In your training code you log a table called "cifar10_sample_table"
:
import wandb
wandb.log({"cifar10_sample_table":<MY_TABLE>})
Within the query panel you can query your table with:
runs.summary["cifar10_sample_table"]

Breaking this down:
runs
is a variable automatically injected in Query Panel Expressions when the Query Panel is in a Workspace. Its “value” is the list of runs which are visible for that particular Workspace. Read about the different attributes available within a run here.summary
is an op which returns the Summary object for a Run. Ops are mapped, meaning this op is applied to each Run in the list, resulting in a list of Summary objects.["cifar10_sample_table"]
is a Pick op (denoted with brackets), with a parameter ofpredictions
. Since Summary objects act like dictionaries or maps, this operation picks thepredictions
field off of each Summary object.
To learn how to write your own queries interactively, see this report.
Configurations
Select the gear icon on the upper left corner of the panel to expand the query configuration. This allows the user to configure the type of panel and the parameters for the result panel.
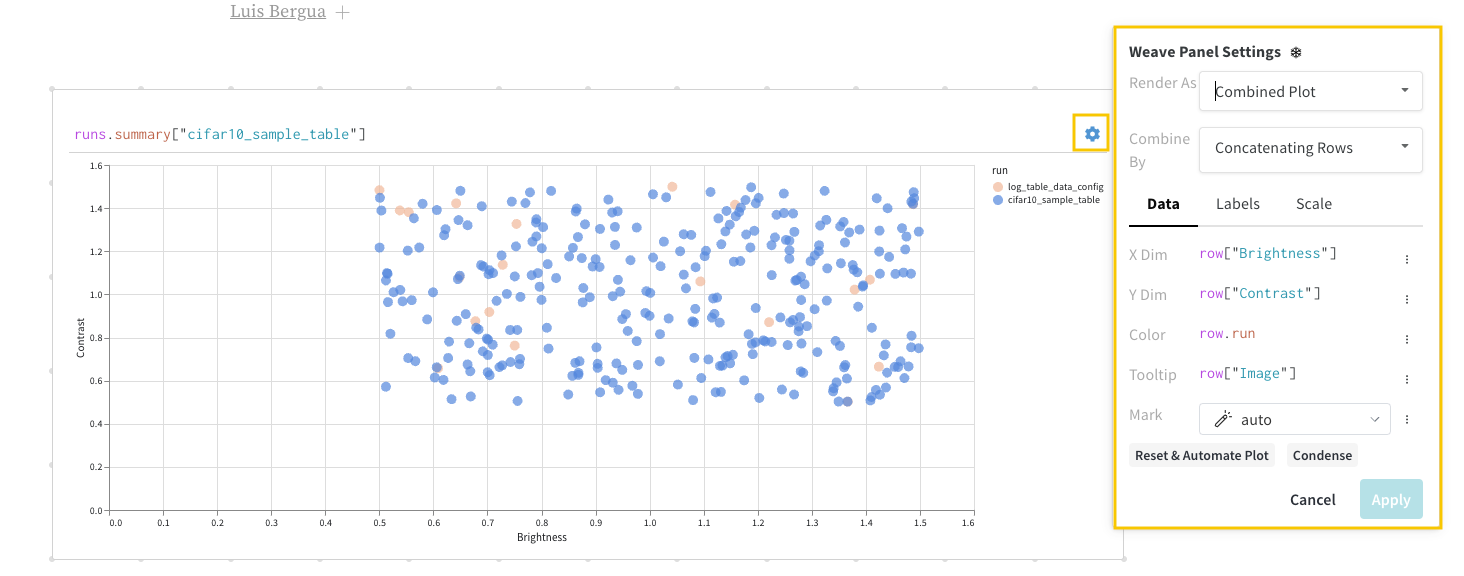
Result panels
Finally, the query result panel renders the result of the query expression, using the selected query panel, configured by the configuration to display the data in an interactive form. The following images shows a Table and a Plot of the same data.
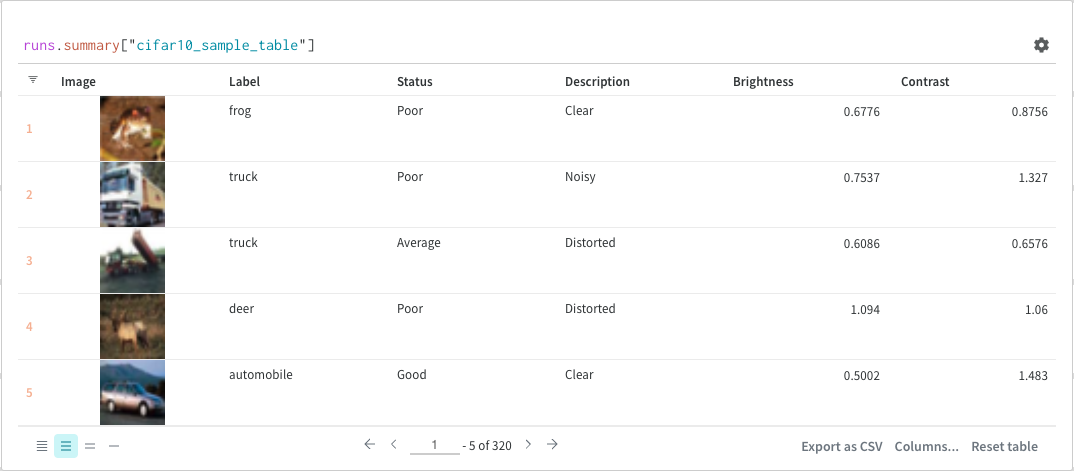
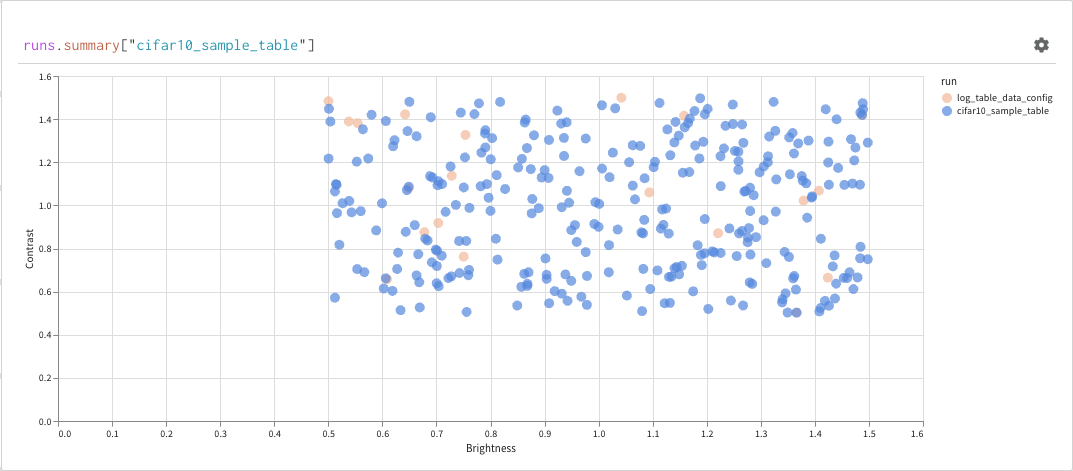
Basic operations
The following common operations you can make within your query panels.
Sort
Sort from the column options:
Filter
You can either filter directly in the query or using the filter button in the top left corner (second image)
Map
Map operations iterate over lists and apply a function to each element in the data. You can do this directly with a panel query or by inserting a new column from the column options.
Groupby
You can groupby using a query or from the column options.
Concat
The concat operation allows you to concatenate 2 tables and concatenate or join from the panel settings
Join
It is also possible to join tables directly in the query. Consider the following query expression:
project("luis_team_test", "weave_example_queries").runs.summary["short_table_0"].table.rows.concat.join(\
project("luis_team_test", "weave_example_queries").runs.summary["short_table_1"].table.rows.concat,\
(row) => row["Label"],(row) => row["Label"], "Table1", "Table2",\
"false", "false")
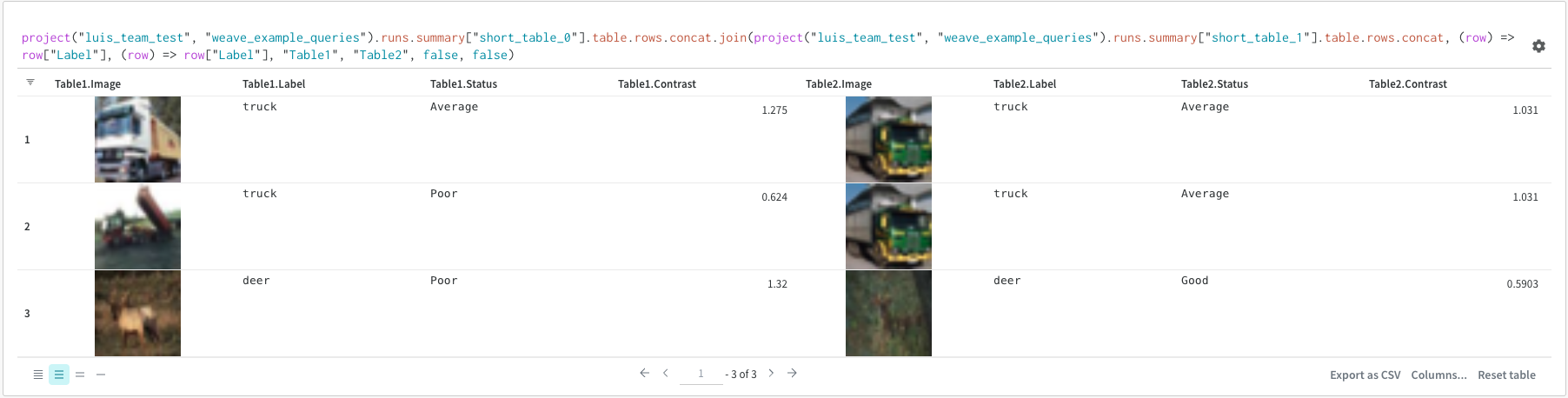
The table on the left is generated from:
project("luis_team_test", "weave_example_queries").\
runs.summary["short_table_0"].table.rows.concat.join
The table in the right is generated from:
project("luis_team_test", "weave_example_queries").\
runs.summary["short_table_1"].table.rows.concat
Where:
(row) => row["Label"]
are selectors for each table, determining which column to join on"Table1"
and"Table2"
are the names of each table when joinedtrue
andfalse
are for left and right inner/outer join settings
Runs object
Use query panels to access the runs
object. Run objects store records of your experiments. You can find more details about it in this section of the report but, as quick overview, runs
object has available:
summary
: A dictionary of information that summarizes the run’s results. This can be scalars like accuracy and loss, or large files. By default,wandb.log()
sets the summary to the final value of a logged time series. You can set the contents of the summary directly. Think of the summary as the run’s outputs.history
: A list of dictionaries meant to store values that change while the model is training such as loss. The commandwandb.log()
appends to this object.config
: A dictionary of the run’s configuration information, such as the hyperparameters for a training run or the preprocessing methods for a run that creates a dataset Artifact. Think of these as the run’s “inputs”
Access Artifacts
Artifacts are a core concept in W&B. They are a versioned, named collection of files and directories. Use Artifacts to track model weights, datasets, and any other file or directory. Artifacts are stored in W&B and can be downloaded or used in other runs. You can find more details and examples in this section of the report. Artifacts are normally accessed from the project
object:
project.artifactVersion()
: returns the specific artifact version for a given name and version within a projectproject.artifact("")
: returns the artifact for a given name within a project. You can then use.versions
to get a list of all versions of this artifactproject.artifactType()
: returns theartifactType
for a given name within a project. You can then use.artifacts
to get a list of all artifacts with this typeproject.artifactTypes
: returns a list of all artifact types under the project