PyTorch Ignite
3 minute read
- See the resulting visualizations in this example W&B report →
- Try running the code yourself in this example hosted notebook →
Ignite supports Weights & Biases handler to log metrics, model/optimizer parameters, gradients during training and validation. It can also be used to log model checkpoints to the Weights & Biases cloud. This class is also a wrapper for the wandb module. This means that you can call any wandb function using this wrapper. See examples on how to save model parameters and gradients.
Basic setup
from argparse import ArgumentParser
import wandb
import torch
from torch import nn
from torch.optim import SGD
from torch.utils.data import DataLoader
import torch.nn.functional as F
from torchvision.transforms import Compose, ToTensor, Normalize
from torchvision.datasets import MNIST
from ignite.engine import Events, create_supervised_trainer, create_supervised_evaluator
from ignite.metrics import Accuracy, Loss
from tqdm import tqdm
class Net(nn.Module):
def __init__(self):
super(Net, self).__init__()
self.conv1 = nn.Conv2d(1, 10, kernel_size=5)
self.conv2 = nn.Conv2d(10, 20, kernel_size=5)
self.conv2_drop = nn.Dropout2d()
self.fc1 = nn.Linear(320, 50)
self.fc2 = nn.Linear(50, 10)
def forward(self, x):
x = F.relu(F.max_pool2d(self.conv1(x), 2))
x = F.relu(F.max_pool2d(self.conv2_drop(self.conv2(x)), 2))
x = x.view(-1, 320)
x = F.relu(self.fc1(x))
x = F.dropout(x, training=self.training)
x = self.fc2(x)
return F.log_softmax(x, dim=-1)
def get_data_loaders(train_batch_size, val_batch_size):
data_transform = Compose([ToTensor(), Normalize((0.1307,), (0.3081,))])
train_loader = DataLoader(MNIST(download=True, root=".", transform=data_transform, train=True),
batch_size=train_batch_size, shuffle=True)
val_loader = DataLoader(MNIST(download=False, root=".", transform=data_transform, train=False),
batch_size=val_batch_size, shuffle=False)
return train_loader, val_loader
Using WandBLogger
in ignite is a modular process. First, you create a WandBLogger object. Next, you attach it to a trainer or evaluator to automatically log the metrics. This example:
- Logs training loss, attached to the trainer object.
- Logs validation loss, attached to the evaluator.
- Logs optional Parameters, such as learning rate.
- Watches the model.
from ignite.contrib.handlers.wandb_logger import *
def run(train_batch_size, val_batch_size, epochs, lr, momentum, log_interval):
train_loader, val_loader = get_data_loaders(train_batch_size, val_batch_size)
model = Net()
device = 'cpu'
if torch.cuda.is_available():
device = 'cuda'
optimizer = SGD(model.parameters(), lr=lr, momentum=momentum)
trainer = create_supervised_trainer(model, optimizer, F.nll_loss, device=device)
evaluator = create_supervised_evaluator(model,
metrics={'accuracy': Accuracy(),
'nll': Loss(F.nll_loss)},
device=device)
desc = "ITERATION - loss: {:.2f}"
pbar = tqdm(
initial=0, leave=False, total=len(train_loader),
desc=desc.format(0)
)
#WandBlogger Object Creation
wandb_logger = WandBLogger(
project="pytorch-ignite-integration",
name="cnn-mnist",
config={"max_epochs": epochs,"batch_size":train_batch_size},
tags=["pytorch-ignite", "mninst"]
)
wandb_logger.attach_output_handler(
trainer,
event_name=Events.ITERATION_COMPLETED,
tag="training",
output_transform=lambda loss: {"loss": loss}
)
wandb_logger.attach_output_handler(
evaluator,
event_name=Events.EPOCH_COMPLETED,
tag="training",
metric_names=["nll", "accuracy"],
global_step_transform=lambda *_: trainer.state.iteration,
)
wandb_logger.attach_opt_params_handler(
trainer,
event_name=Events.ITERATION_STARTED,
optimizer=optimizer,
param_name='lr' # optional
)
wandb_logger.watch(model)
You can optionally utilize ignite EVENTS
to log the metrics directly to the terminal
@trainer.on(Events.ITERATION_COMPLETED(every=log_interval))
def log_training_loss(engine):
pbar.desc = desc.format(engine.state.output)
pbar.update(log_interval)
@trainer.on(Events.EPOCH_COMPLETED)
def log_training_results(engine):
pbar.refresh()
evaluator.run(train_loader)
metrics = evaluator.state.metrics
avg_accuracy = metrics['accuracy']
avg_nll = metrics['nll']
tqdm.write(
"Training Results - Epoch: {} Avg accuracy: {:.2f} Avg loss: {:.2f}"
.format(engine.state.epoch, avg_accuracy, avg_nll)
)
@trainer.on(Events.EPOCH_COMPLETED)
def log_validation_results(engine):
evaluator.run(val_loader)
metrics = evaluator.state.metrics
avg_accuracy = metrics['accuracy']
avg_nll = metrics['nll']
tqdm.write(
"Validation Results - Epoch: {} Avg accuracy: {:.2f} Avg loss: {:.2f}"
.format(engine.state.epoch, avg_accuracy, avg_nll))
pbar.n = pbar.last_print_n = 0
trainer.run(train_loader, max_epochs=epochs)
pbar.close()
if __name__ == "__main__":
parser = ArgumentParser()
parser.add_argument('--batch_size', type=int, default=64,
help='input batch size for training (default: 64)')
parser.add_argument('--val_batch_size', type=int, default=1000,
help='input batch size for validation (default: 1000)')
parser.add_argument('--epochs', type=int, default=10,
help='number of epochs to train (default: 10)')
parser.add_argument('--lr', type=float, default=0.01,
help='learning rate (default: 0.01)')
parser.add_argument('--momentum', type=float, default=0.5,
help='SGD momentum (default: 0.5)')
parser.add_argument('--log_interval', type=int, default=10,
help='how many batches to wait before logging training status')
args = parser.parse_args()
run(args.batch_size, args.val_batch_size, args.epochs, args.lr, args.momentum, args.log_interval)
This code generates these visualizations::
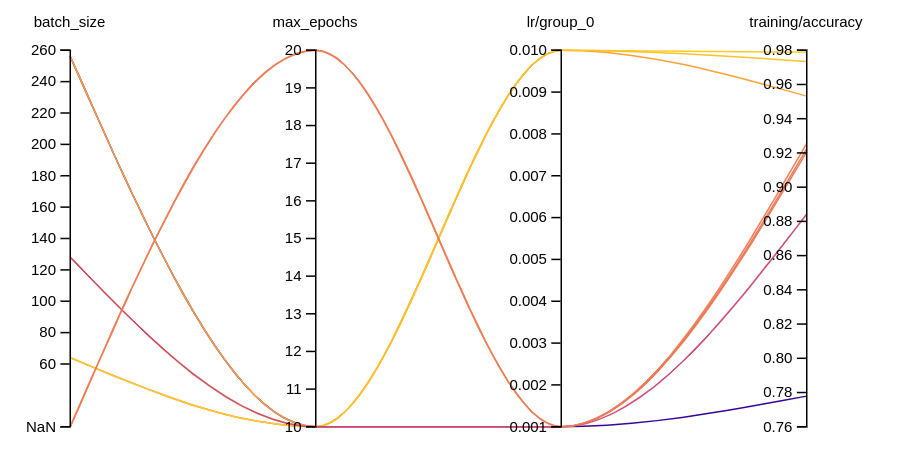
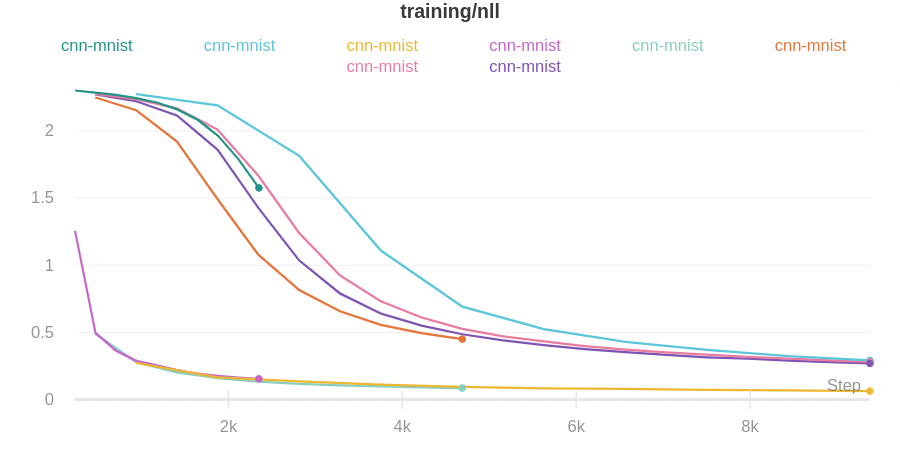
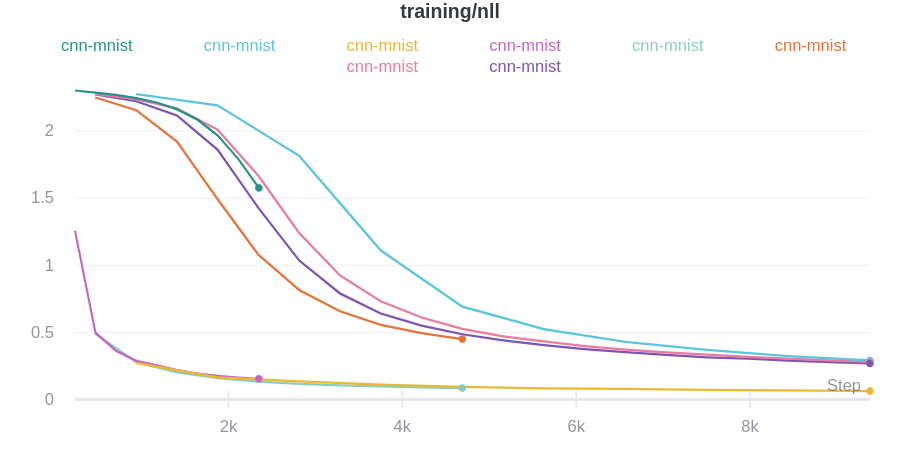
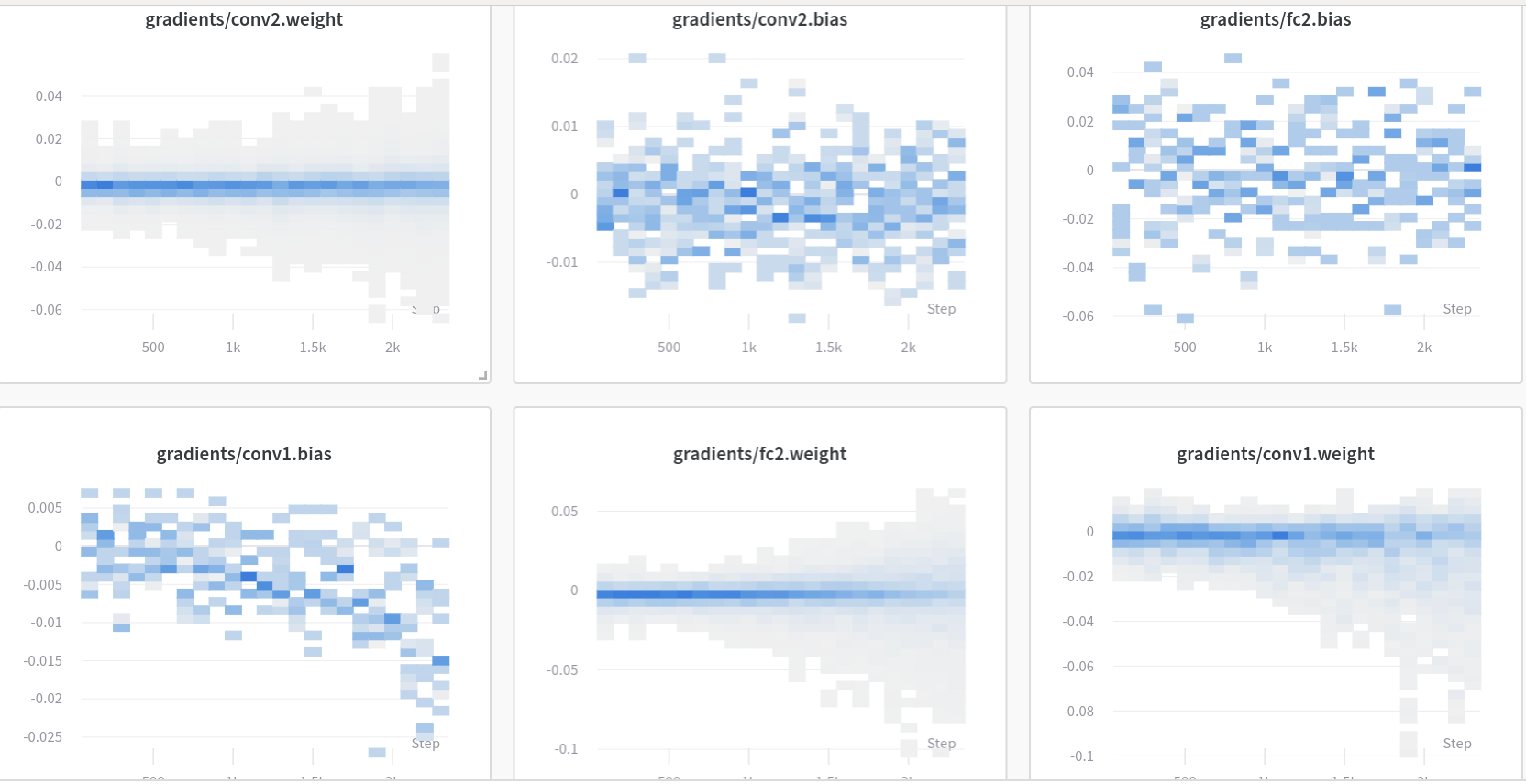
Refer to the Ignite Docs for more details.
Feedback
Was this page helpful?
Glad to hear it! Please tell us how we can improve.
Sorry to hear that. Please tell us how we can improve.