W&B Models
less than a minute
W&B Models is the system of record for ML Practitioners who want to organize their models, boost productivity and collaboration, and deliver production ML at scale.
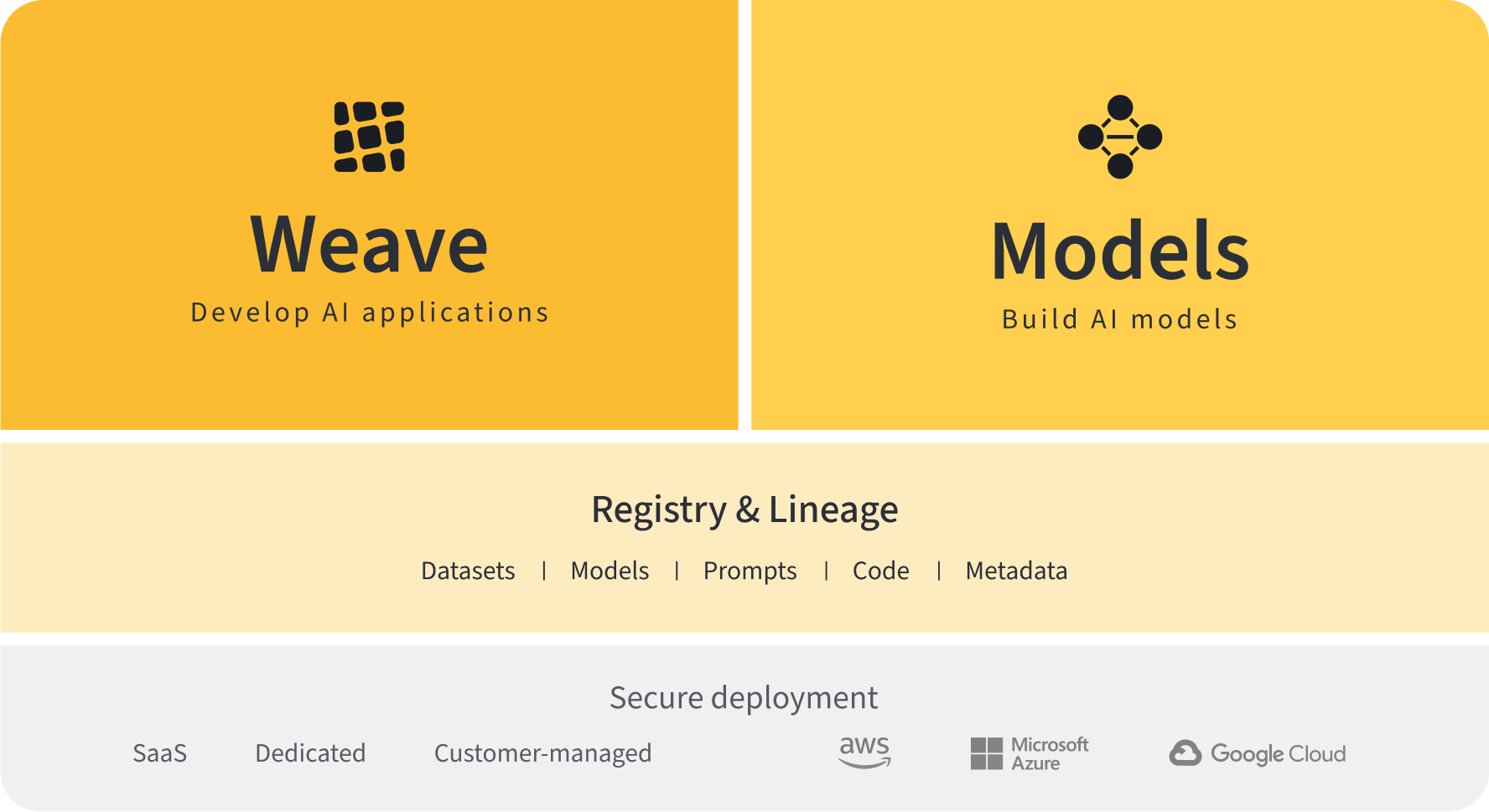
With W&B Models, you can:
- Track and visualize all ML experiments.
- Optimize and fine-tune models at scale with hyperparameter sweeps.
- Maintain a centralized hub of all models, with a seamless handoff point to devops and deployment teams.
- Configure custom automations that trigger key workflows for model CI/CD.
Machine learning practitioners rely on W&B Models as their ML system of record to track and visualize experiments, manage model versions and lineage, and optimize hyperparameters.
Feedback
Was this page helpful?
Glad to hear it! Please tell us how we can improve.
Sorry to hear that. Please tell us how we can improve.