W&B Quickstart
2 minute read
Install W&B to track, visualize, and manage machine learning experiments of any size.
Sign up and create an API key
To authenticate your machine with W&B, generate an API key from your user profile or at wandb.ai/authorize. Copy the API key and store it securely.
Install the wandb
library and log in
-
Set the
WANDB_API_KEY
environment variable.export WANDB_API_KEY=<your_api_key>
-
Install the
wandb
library and log in.pip install wandb wandb login
pip install wandb
import wandb
wandb.login()
!pip install wandb
import wandb
wandb.login()
Start a run and track hyperparameters
In your Python script or notebook, initialize a W&B run object with wandb.init()
. Use a dictionary for the config
parameter to specify hyperparameter names and values.
run = wandb.init(
project="my-awesome-project", # Specify your project
config={ # Track hyperparameters and metadata
"learning_rate": 0.01,
"epochs": 10,
},
)
A run serves as the core element of W&B, used to track metrics, create logs, and more.
Assemble the components
This mock training script logs simulated accuracy and loss metrics to W&B:
# train.py
import wandb
import random
wandb.login()
epochs = 10
lr = 0.01
run = wandb.init(
project="my-awesome-project", # Specify your project
config={ # Track hyperparameters and metadata
"learning_rate": lr,
"epochs": epochs,
},
)
offset = random.random() / 5
print(f"lr: {lr}")
# Simulate a training run
for epoch in range(2, epochs):
acc = 1 - 2**-epoch - random.random() / epoch - offset
loss = 2**-epoch + random.random() / epoch + offset
print(f"epoch={epoch}, accuracy={acc}, loss={loss}")
wandb.log({"accuracy": acc, "loss": loss})
# run.log_code()
Visit wandb.ai/home to view recorded metrics such as accuracy and loss and how they changed during each training step. The following image shows the loss and accuracy tracked from each run. Each run object appears in the Runs column with generated names.
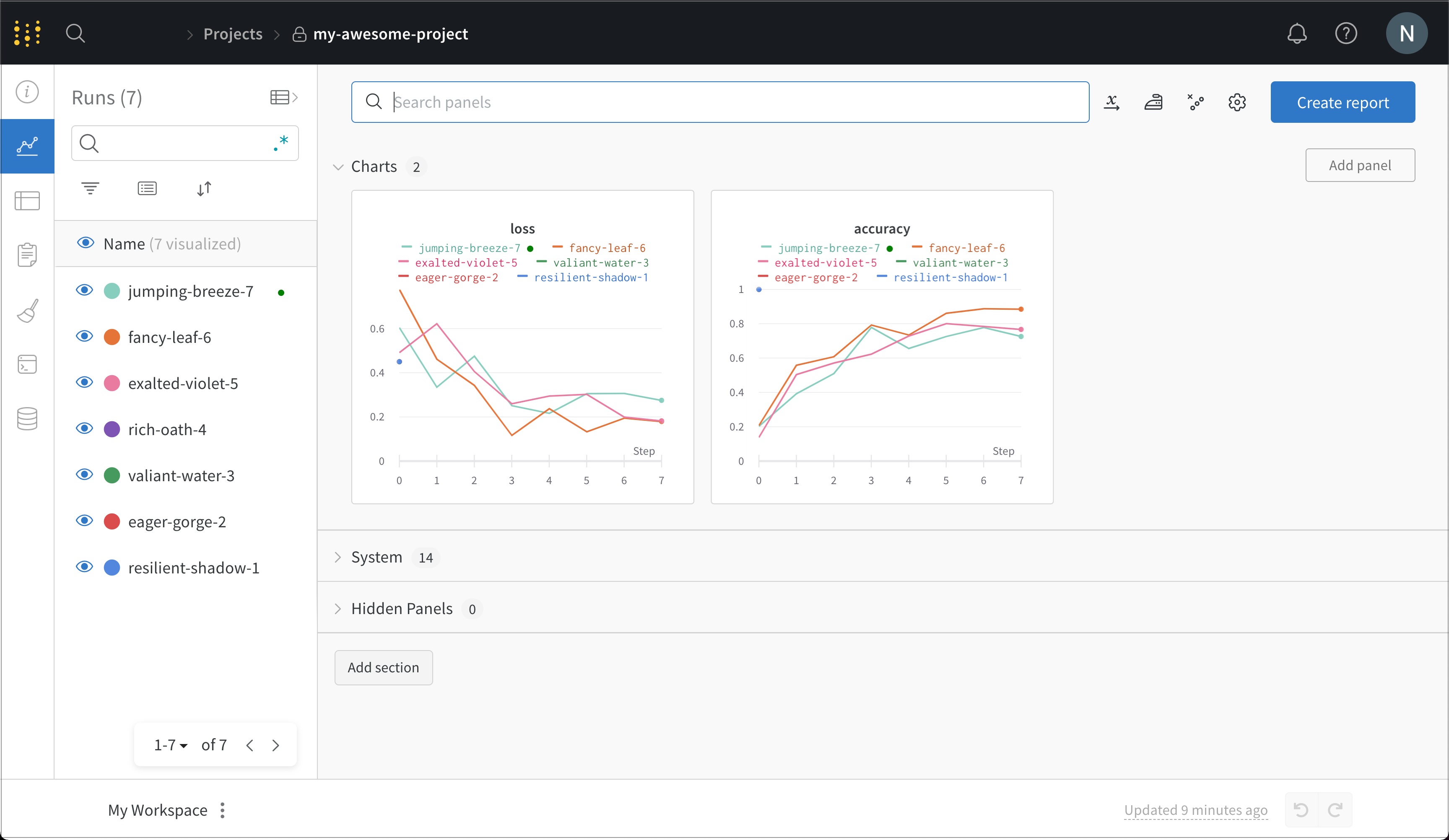
Next steps
Explore more features of the W&B ecosystem:
- Read the W&B Integration tutorials that combine W&B with frameworks like PyTorch, libraries like Hugging Face, and services like SageMaker.
- Organize runs, automate visualizations, summarize findings, and share updates with collaborators using W&B Reports.
- Create W&B Artifacts to track datasets, models, dependencies, and results throughout your machine learning pipeline.
- Automate hyperparameter searches and optimize models with W&B Sweeps.
- Analyze runs, visualize model predictions, and share insights on a central dashboard.
- Visit W&B AI Academy to learn about LLMs, MLOps, and W&B Models through hands-on courses.
Feedback
Was this page helpful?
Glad to hear it! Please tell us how we can improve.
Sorry to hear that. Please tell us how we can improve.